A Deep Learning Method To Impute Missing Values And Compress Genome-Wide Polymorphism Data In Rice
PROCEEDINGS OF THE 14TH INTERNATIONAL JOINT CONFERENCE ON BIOMEDICAL ENGINEERING SYSTEMS AND TECHNOLOGIES, VOL 3: BIOINFORMATICS(2021)
摘要
Missing value imputation and compressing genome-wide DNA polymorphism data are considered as a challenging task in genomic data analysis. Missing data consists in the lack of information in a dataset that directly influences data analysis performance. The aim is to develop a deep learning model named Autoencoder Genome Imputation and Compression (AGIC) which can impute missing values and compress genome-wide polymorphism data using a separated neural network model to reduce the computational time. This research will challenge the construction of a model by using Autoencoder for genomic analysis, in other words, a fusion research between agriculture and information sciences. Moreover, there is no knowledge of missing value imputation and genome-wide polymorphism data compression using Separated Stacking Autoencoder Model. The main contributions are: (1) missing value imputation of genome-wide polymorphism data, (2) genome-wide polymorphism data compression of Rice DNA. To demonstrate the usage of AGIC model, real genome-wide polymorphism data from a rice MAGIC population has been used.
更多查看译文
关键词
Genome-wide DNA Polymorphisms, Stacked Autoencoder, Deep Neural Network, Separate Stacking Model, Genome Compression, Missing Value Imputation
AI 理解论文
溯源树
样例
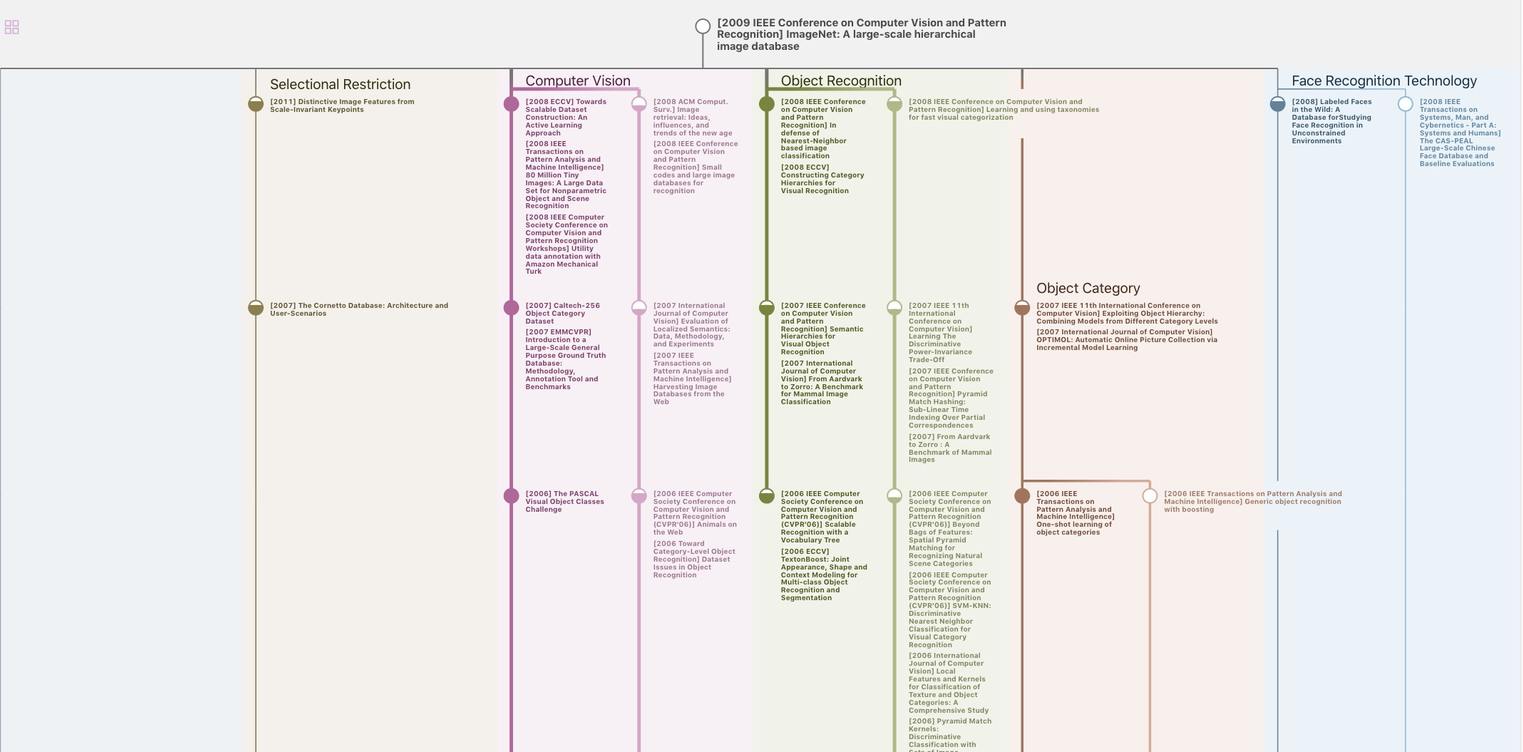
生成溯源树,研究论文发展脉络
Chat Paper
正在生成论文摘要