Predicting Driver Fatigue in Automated Driving with Explainability
arxiv(2021)
摘要
Research indicates that monotonous automated driving increases the incidence of fatigued driving. Although many prediction models based on advanced machine learning techniques were proposed to monitor driver fatigue, especially in manual driving, little is known about how these black-box machine learning models work. In this paper, we proposed a combination of eXtreme Gradient Boosting (XGBoost) and SHAP (SHapley Additive exPlanations) to predict driver fatigue with explanations due to their efficiency and accuracy. First, in order to obtain the ground truth of driver fatigue, PERCLOS (percentage of eyelid closure over the pupil over time) between 0 and 100 was used as the response variable. Second, we built a driver fatigue regression model using both physiological and behavioral measures with XGBoost and it outperformed other selected machine learning models with 3.847 root-mean-squared error (RMSE), 1.768 mean absolute error (MAE) and 0.996 adjusted $R^2$. Third, we employed SHAP to identify the most important predictor variables and uncovered the black-box XGBoost model by showing the main effects of most important predictor variables globally and explaining individual predictions locally. Such an explainable driver fatigue prediction model offered insights into how to intervene in automated driving when necessary, such as during the takeover transition period from automated driving to manual driving.
更多查看译文
AI 理解论文
溯源树
样例
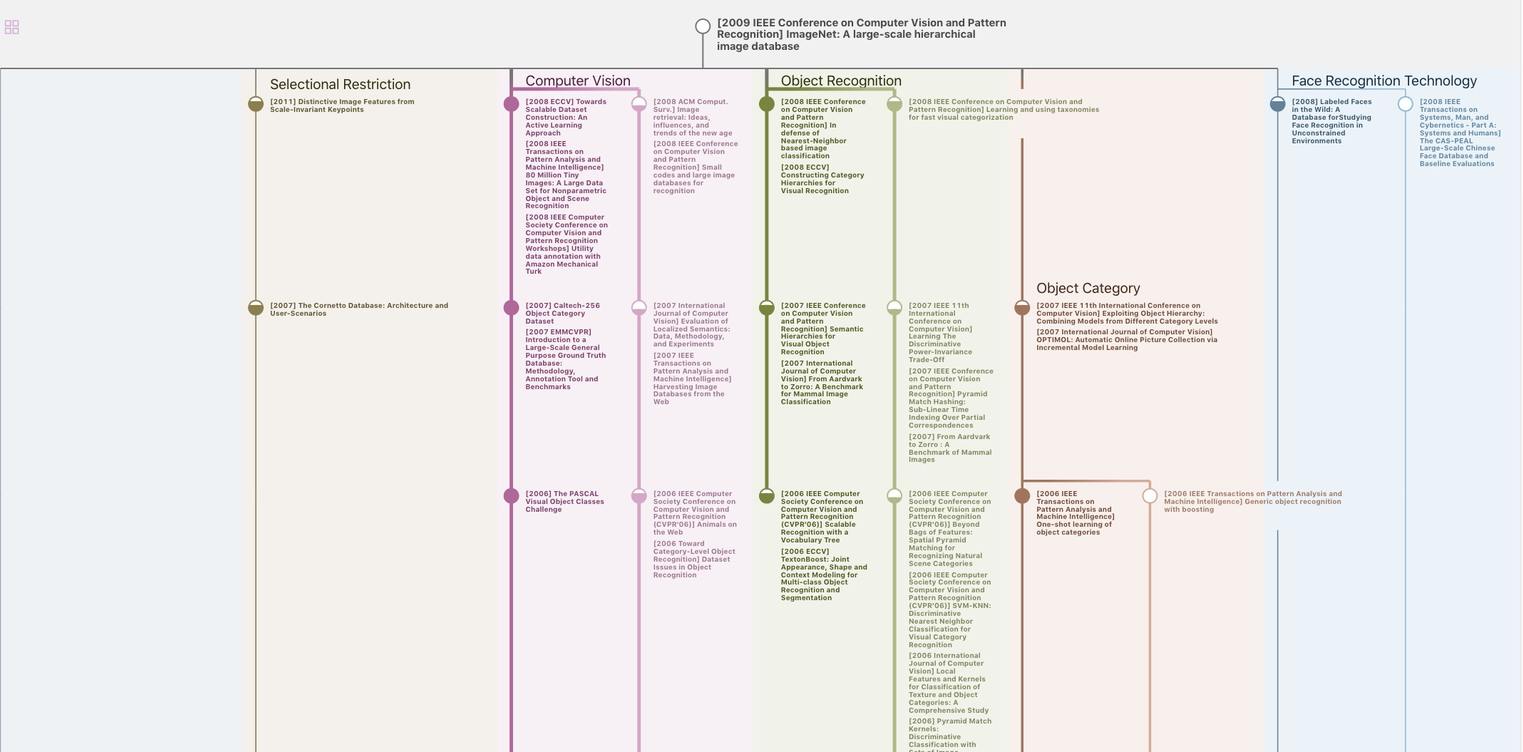
生成溯源树,研究论文发展脉络
Chat Paper
正在生成论文摘要