Depth from Camera Motion and Object Detection
2021 IEEE/CVF CONFERENCE ON COMPUTER VISION AND PATTERN RECOGNITION, CVPR 2021(2021)
摘要
This paper addresses the problem of learning to estimate the depth of detected objects given some measurement of camera motion (e.g., from robot kinematics or vehicle odometry). We achieve this by 1) designing a recurrent neural network (DBox) that estimates the depth of objects using a generalized representation of bounding boxes and uncalibrated camera movement and 2) introducing the Object Depth via Motion and Detection Dataset (ODMD). ODMD training data are extensible and configurable, and the ODMD benchmark includes 21,600 examples across four validation and test sets. These sets include mobile robot experiments using an end-effector camera to locate objects from the YCB dataset and examples with perturbations added to camera motion or bounding box data. In addition to the ODMD benchmark, we evaluate DBox in other monocular application domains, achieving state-of-the-art results on existing driving and robotics benchmarks and estimating the depth of objects using a camera phone.
更多查看译文
关键词
camera motion,object detection,depth
AI 理解论文
溯源树
样例
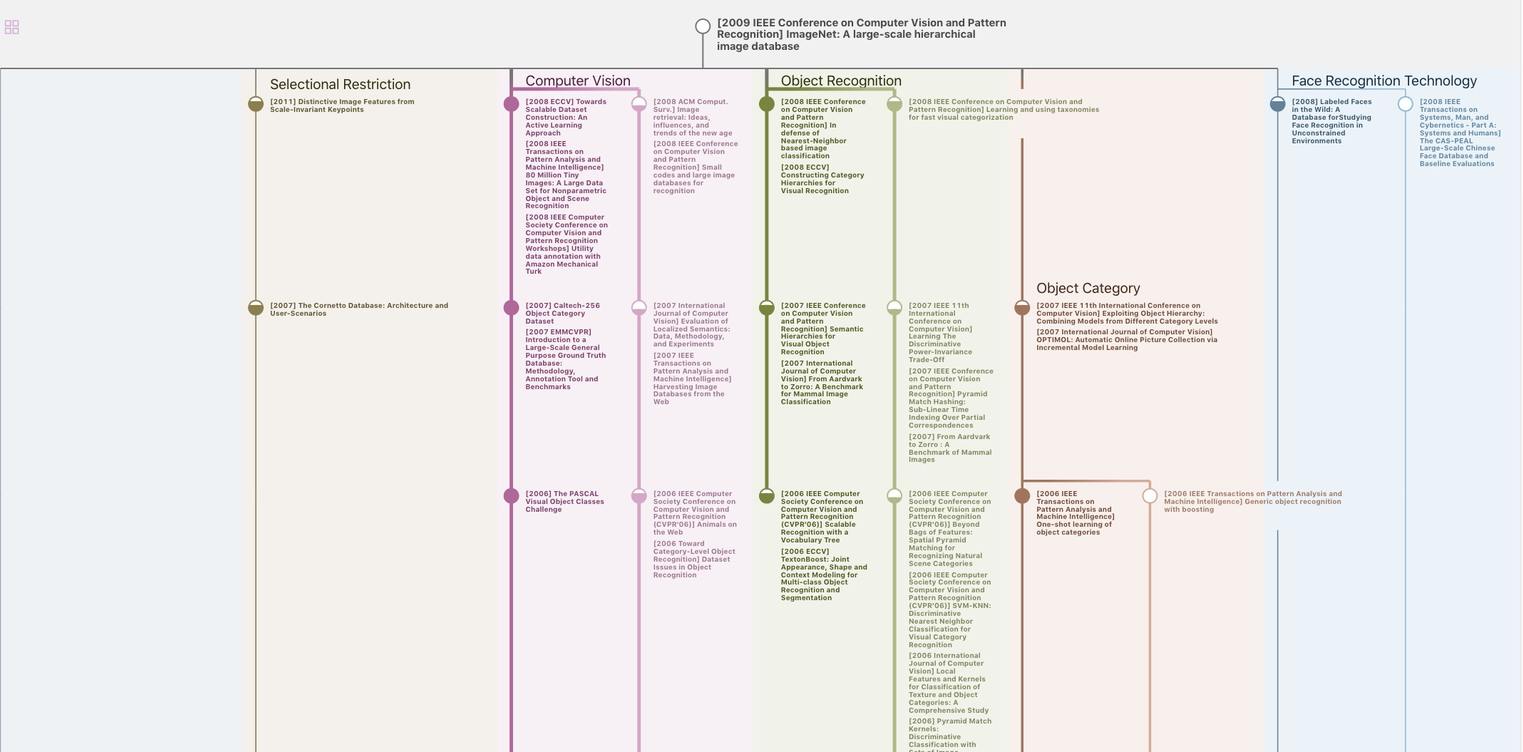
生成溯源树,研究论文发展脉络
Chat Paper
正在生成论文摘要