Extremely low-resource neural machine translation for Asian languages
MACHINE TRANSLATION(2021)
摘要
This paper presents a set of effective approaches to handle extremely low-resource language pairs for self-attention based neural machine translation (NMT) focusing on English and four Asian languages. Starting from an initial set of parallel sentences used to train bilingual baseline models, we introduce additional monolingual corpora and data processing techniques to improve translation quality. We describe a series of best practices and empirically validate the methods through an evaluation conducted on eight translation directions, based on state-of-the-art NMT approaches such as hyper-parameter search, data augmentation with forward and backward translation in combination with tags and noise, as well as joint multilingual training. Experiments show that the commonly used default architecture of self-attention NMT models does not reach the best results, validating previous work on the importance of hyper-parameter tuning. Additionally, empirical results indicate the amount of synthetic data required to efficiently increase the parameters of the models leading to the best translation quality measured by automatic metrics. We show that the best NMT models trained on large amount of tagged back-translations outperform three other synthetic data generation approaches. Finally, comparison with statistical machine translation (SMT) indicates that extremely low-resource NMT requires a large amount of synthetic parallel data obtained with back-translation in order to close the performance gap with the preceding SMT approach.
更多查看译文
关键词
Neural machine translation, Low-resource, Asian language, Transformer, Synthetic data, Hyper-parameter tuning
AI 理解论文
溯源树
样例
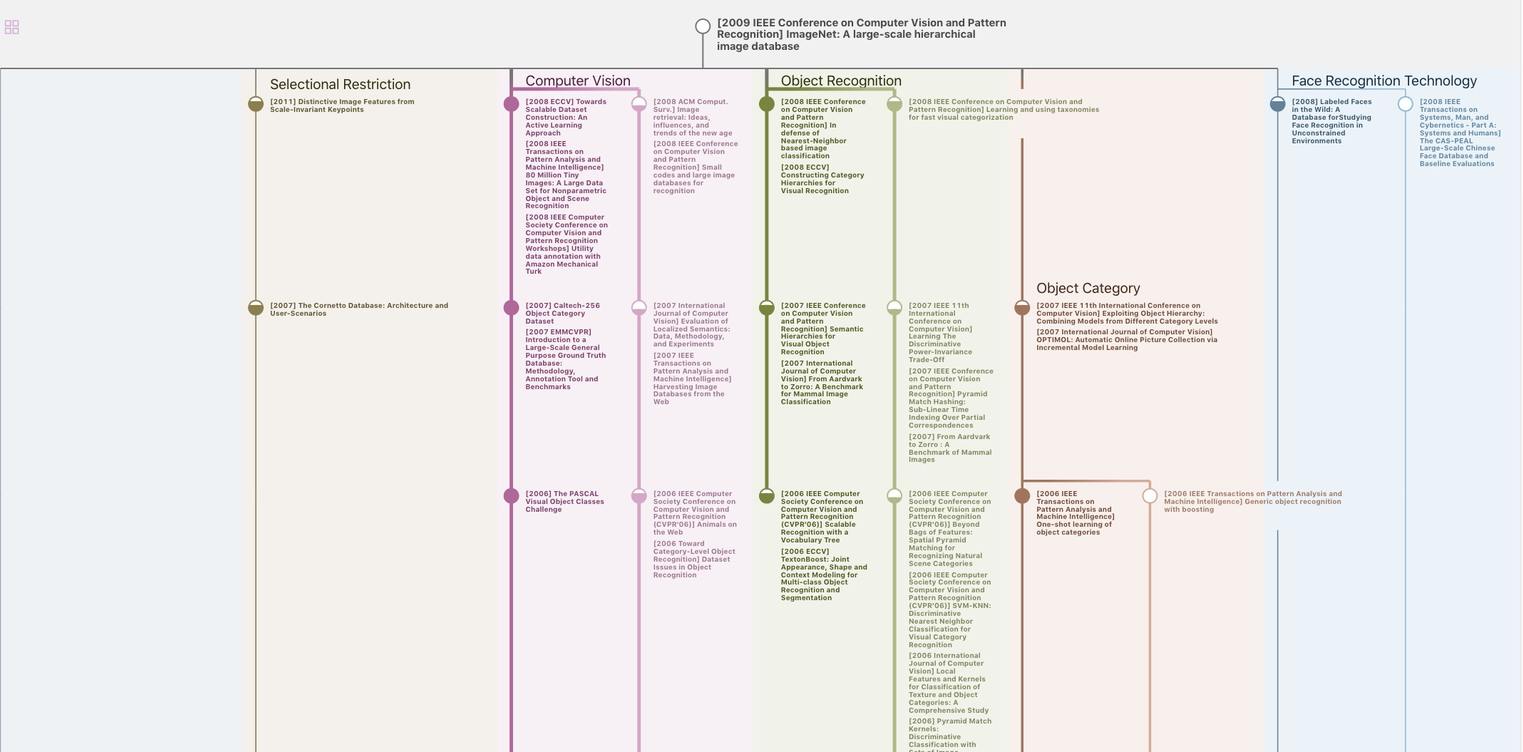
生成溯源树,研究论文发展脉络
Chat Paper
正在生成论文摘要