Robustness against Relational Adversary
user-5f8411ab4c775e9685ff56d3(2020)
摘要
Test-time adversarial attacks have posed serious challenges to the robustness of machine-learning models, and in many settings the adversarial perturbation need not be bounded by small $\ell_p$-norms. Motivated by the semantics-preserving attacks in vision and security domain, we investigate $\textit{relational adversaries}$, a broad class of attackers who create adversarial examples that are in a reflexive-transitive closure of a logical relation. We analyze the conditions for robustness and propose $\textit{normalize-and-predict}$ -- a learning framework with provable robustness guarantee. We compare our approach with adversarial training and derive an unified framework that provides benefits of both approaches. Guided by our theoretical findings, we apply our framework to image classification and malware detection. Results of both tasks show that attacks using relational adversaries frequently fool existing models, but our unified framework can significantly enhance their robustness.
更多查看译文
关键词
Robustness (computer science),Security domain,Malware,Adversarial system,Bounded function,Theoretical computer science,Adversary,Computer science,Contextual image classification
AI 理解论文
溯源树
样例
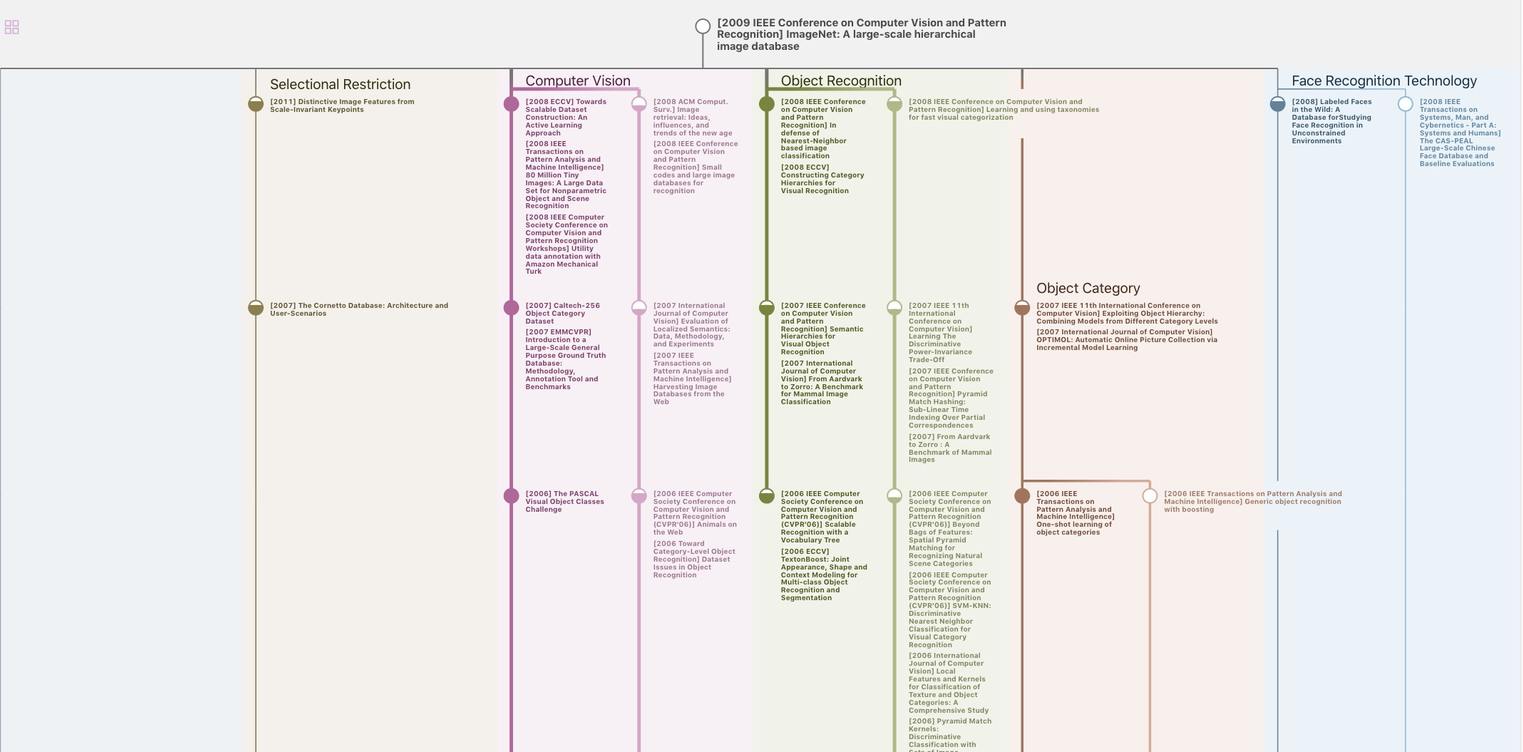
生成溯源树,研究论文发展脉络
Chat Paper
正在生成论文摘要