Spline parameterization of neural network controls for deep learning
ArXiv(2021)
摘要
Based on the continuous interpretation of deep learning cast as an optimal control problem, this paper investigates the benefits of employing B-spline basis functions to parameterize neural network controls across the layers. Rather than equipping each layer of a discretized ODE-network with a set of trainable weights, we choose a fixed number of B-spline basis functions whose coefficients are the trainable parameters of the neural network. Decoupling the trainable parameters from the layers of the neural network enables us to investigate and adapt the accuracy of the network propagation separated from the optimization learning problem. We numerically show that the spline-based neural network increases robustness of the learning problem towards hyperparameters due to increased stability and accuracy of the network propagation. Further, training on B-spline coefficients rather than layer weights directly enables a reduction in the number of trainable parameters.
更多查看译文
关键词
spline parameterization,neural network controls,neural network
AI 理解论文
溯源树
样例
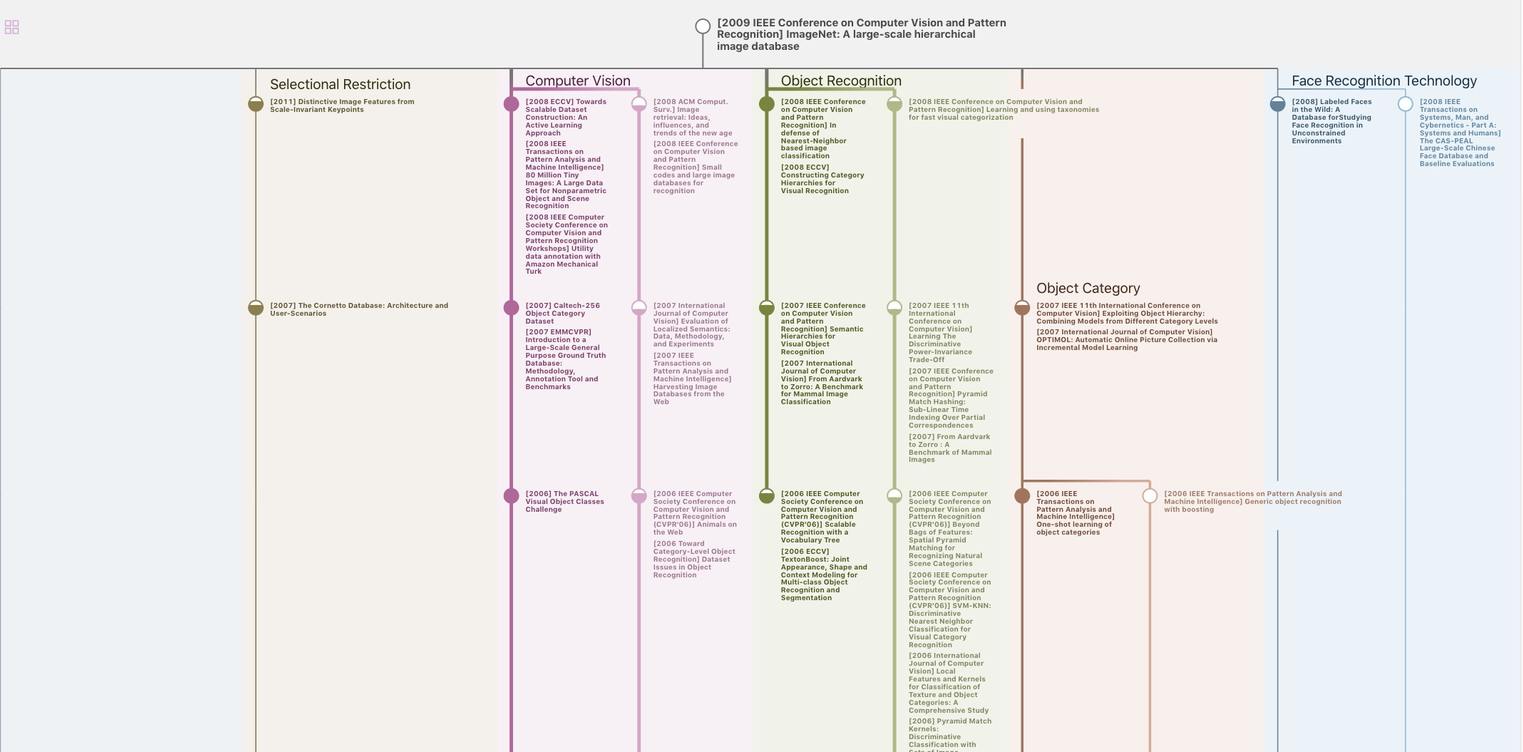
生成溯源树,研究论文发展脉络
Chat Paper
正在生成论文摘要