GRAD-MATCH: A Gradient Matching Based Data Subset Selection for Efficient Learning
ICML 2021(2021)
摘要
The great success of modern machine learning models on large datasets is contingent on extensive computational resources with high financial and environmental costs. One way to address this is by extracting subsets that generalize on par with the full data. In this work, we propose a general framework, GRAD-MATCH, which finds subsets that closely match the gradient of the training or validation set. We find such subsets effectively using an orthogonal matching pursuit algorithm. We show rigorous theoretical and convergence guarantees of the proposed algorithm and, through our extensive experiments on real-world datasets, show the effectiveness of our proposed framework. We show that GRAD-MATCH significantly and consistently outperforms several recent data-selection algorithms and is Pareto-optimal with respect to the accuracy-efficiency trade-off. The code of GRADMATCH is available as a part of the CORDS toolkit: https://github.com/decile-team/cords.
更多查看译文
关键词
efficient learning,selection,matching,grad-match
AI 理解论文
溯源树
样例
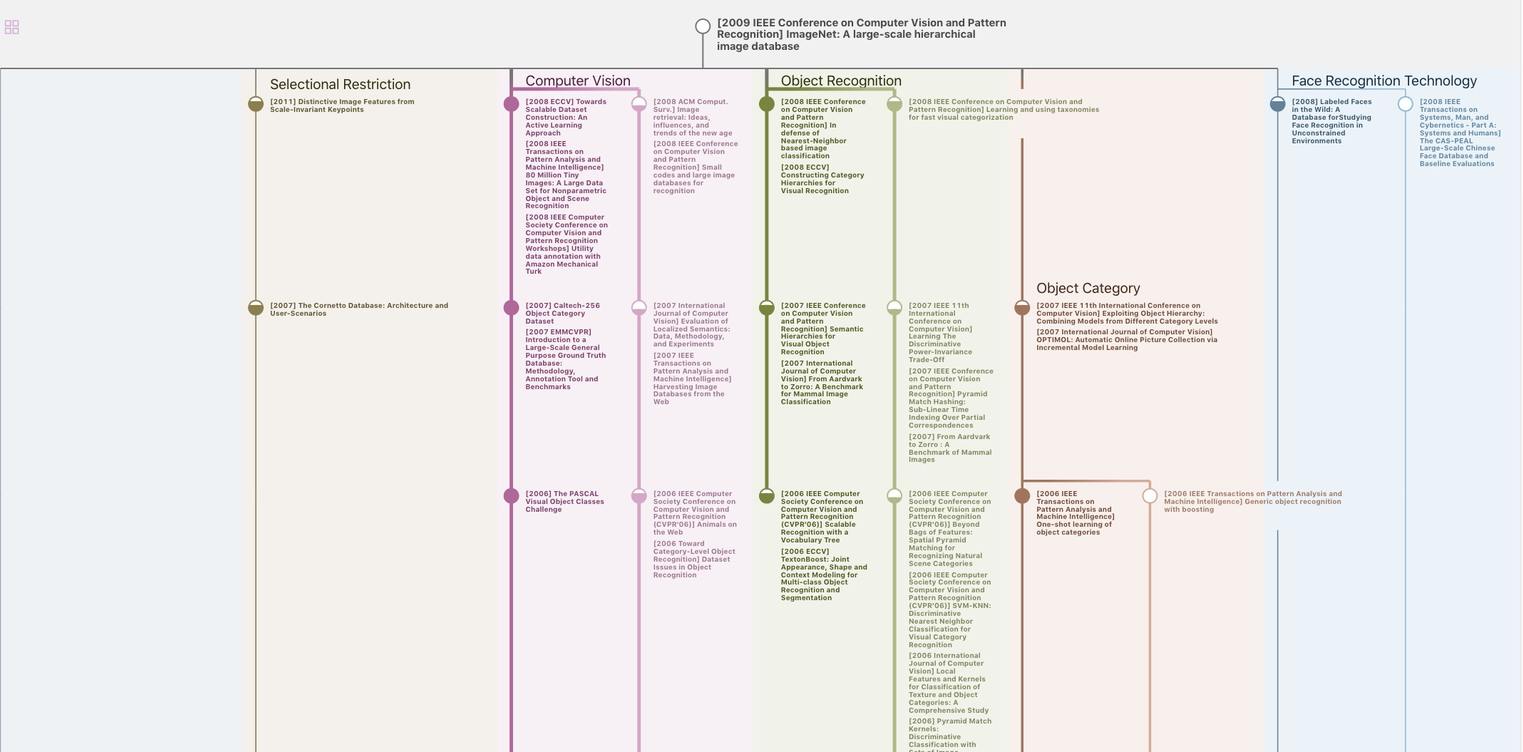
生成溯源树,研究论文发展脉络
Chat Paper
正在生成论文摘要