What Do Compressed Deep Neural Networks Forget
user-5f8411ab4c775e9685ff56d3(2019)
摘要
Deep neural network pruning and quantization techniques have demonstrated it is possible to achieve high levels of compression with surprisingly little degradation to test set accuracy. However, this measure of performance conceals significant differences in how different classes and images are impacted by model compression techniques. We find that models with radically different numbers of weights have comparable top-line performance metrics but diverge considerably in behavior on a narrow subset of the dataset. This small subset of data points, which we term Pruning Identified Exemplars (PIEs) are systematically more impacted by the introduction of sparsity. Compression disproportionately impacts model performance on the underrepresented long-tail of the data distribution. PIEs over-index on atypical or noisy images that are far more challenging for both humans and algorithms to classify. Our work provides intuition into the role of capacity in deep neural networks and the trade-offs incurred by compression. An understanding of this disparate impact is critical given the widespread deployment of compressed models in the wild.
更多查看译文
关键词
Artificial neural network,Test set,Quantization (signal processing),Data point,Machine learning,Computer science,Software deployment,Artificial intelligence,Deep neural networks,Intuition,Model compression
AI 理解论文
溯源树
样例
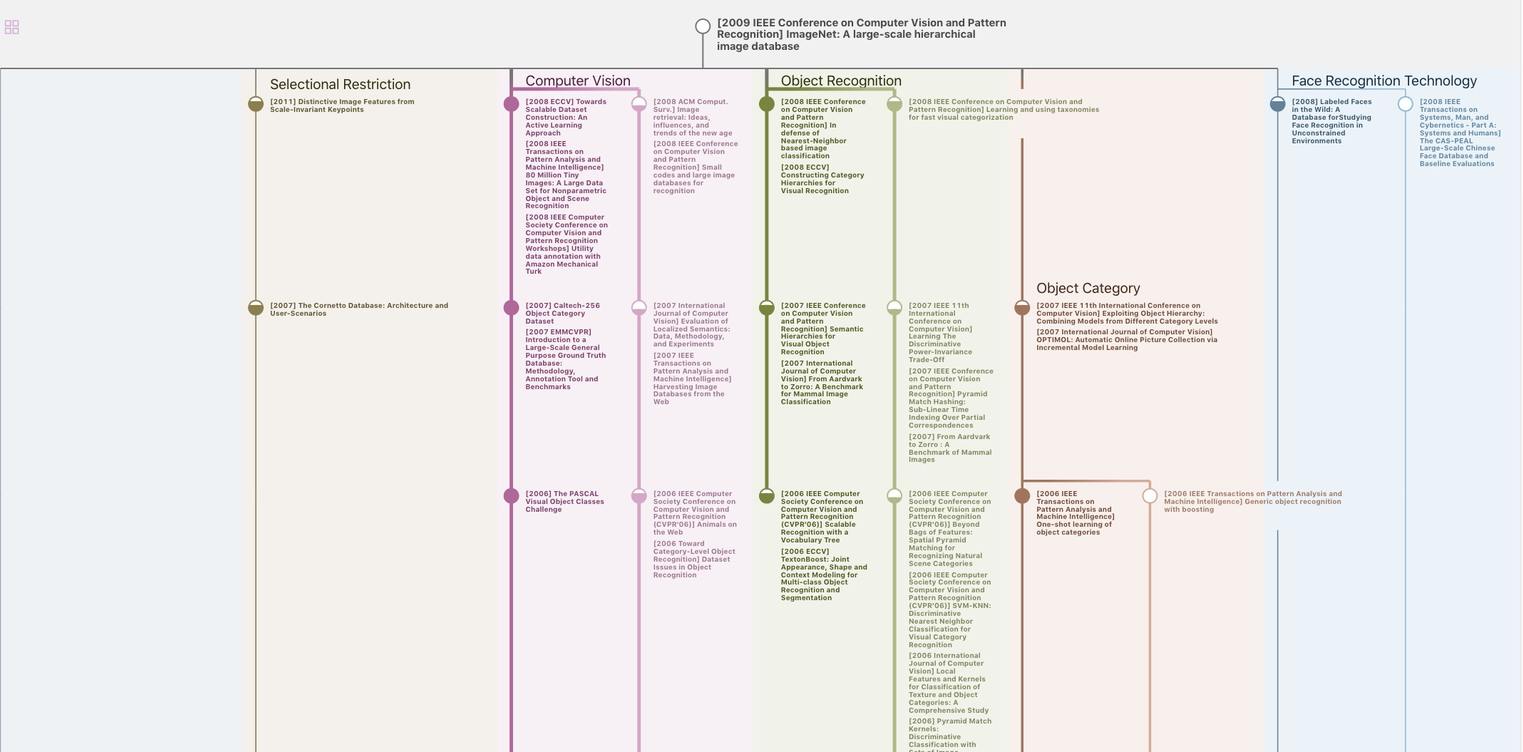
生成溯源树,研究论文发展脉络
Chat Paper
正在生成论文摘要