DEEP LEARNING MODELS FOR SPEECH RECOGNITION
user-5f8cf7e04c775ec6fa691c92(2019)
摘要
Presented herein are embodiments of state-of-the-art speech recognition systems developed using end-to-end deep learning. In embodiments, the model architecture is significantly simpler than traditional speech systems, which rely on laboriously engineered processing pipelines; these traditional systems also tend to perform poorly when used in noisy environments. In contrast, embodiments of the system do not need hand-designed components to model background noise, reverberation, or speaker variation, but instead directly learn a function that is robust to such effects. A phoneme dictionary, nor even the concept of a “phoneme,” is needed. Embodiments include a well-optimized recurrent neural network (RNN) training system that can use multiple GPUs, as well as a set of novel data synthesis techniques that allows for a large amount of varied data for training to be efficiently obtained. Embodiments of the system can also handle challenging noisy environments better than widely used, state-of-the-art commercial speech systems.
更多查看译文
关键词
Recurrent neural network,Deep learning,Background noise,Reverberation,Speech recognition,Commercial speech,Training system,Computer science,Artificial intelligence,Data synthesis,Model architecture
AI 理解论文
溯源树
样例
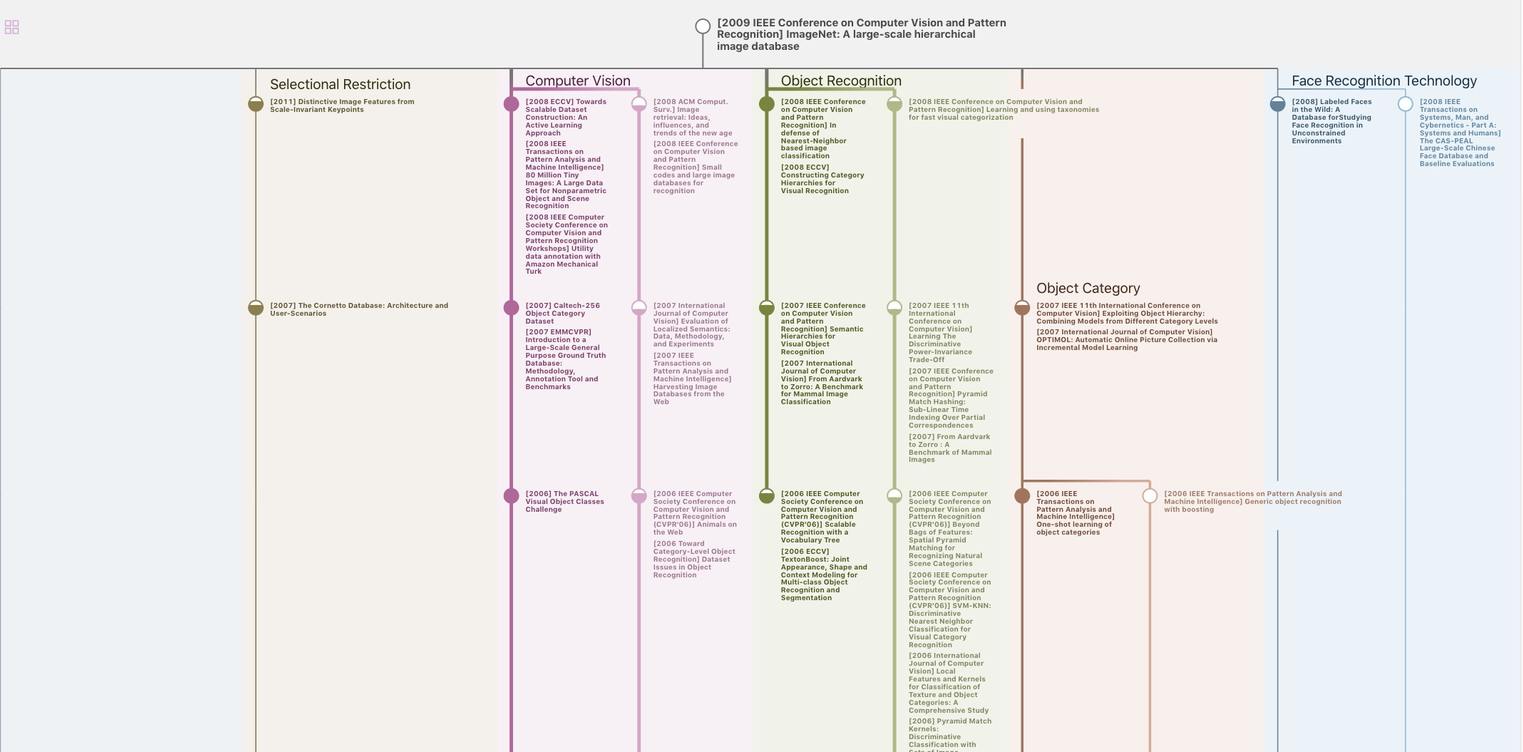
生成溯源树,研究论文发展脉络
Chat Paper
正在生成论文摘要