Large-Scale Subspace Clustering by Independent Distributed and Parallel Coding
IEEE Transactions on Cybernetics(2022)
摘要
Subspace clustering is a popular method to discover underlying low-dimensional structures of high-dimensional multimedia data (e.g., images, videos, and texts). In this article, we consider a large-scale subspace clustering (LS
2
C) problem, that is, partitioning million data points with a millon dimensions. To address this, we explore an independent distributed and parallel framework by dividing big data/variable matrices and regularization by both columns and rows. Specifically, LS
2
C is independently decomposed into many subproblems by distributing those matrices into different machines by columns since the regularization of the code matrix is equal to a sum of that of its submatrices (e.g., square-of-Frobenius/
$\ell _{1}$
-norm). Consensus optimization is designed to solve these subproblems in a parallel way for saving communication costs. Moreover, we provide theoretical guarantees that LS
2
C can recover consensus subspace representations of high-dimensional data points under broad conditions. Compared with the state-of-the-art LS
2
C methods, our approach achieves better clustering results in public datasets, including a million images and videos.
更多查看译文
关键词
Distributed and parallel computing,least-squares regression (LSR),low-rank representation (LRR),over-high dimensional big data,sparse subspace clustering (SSC),subspace clustering
AI 理解论文
溯源树
样例
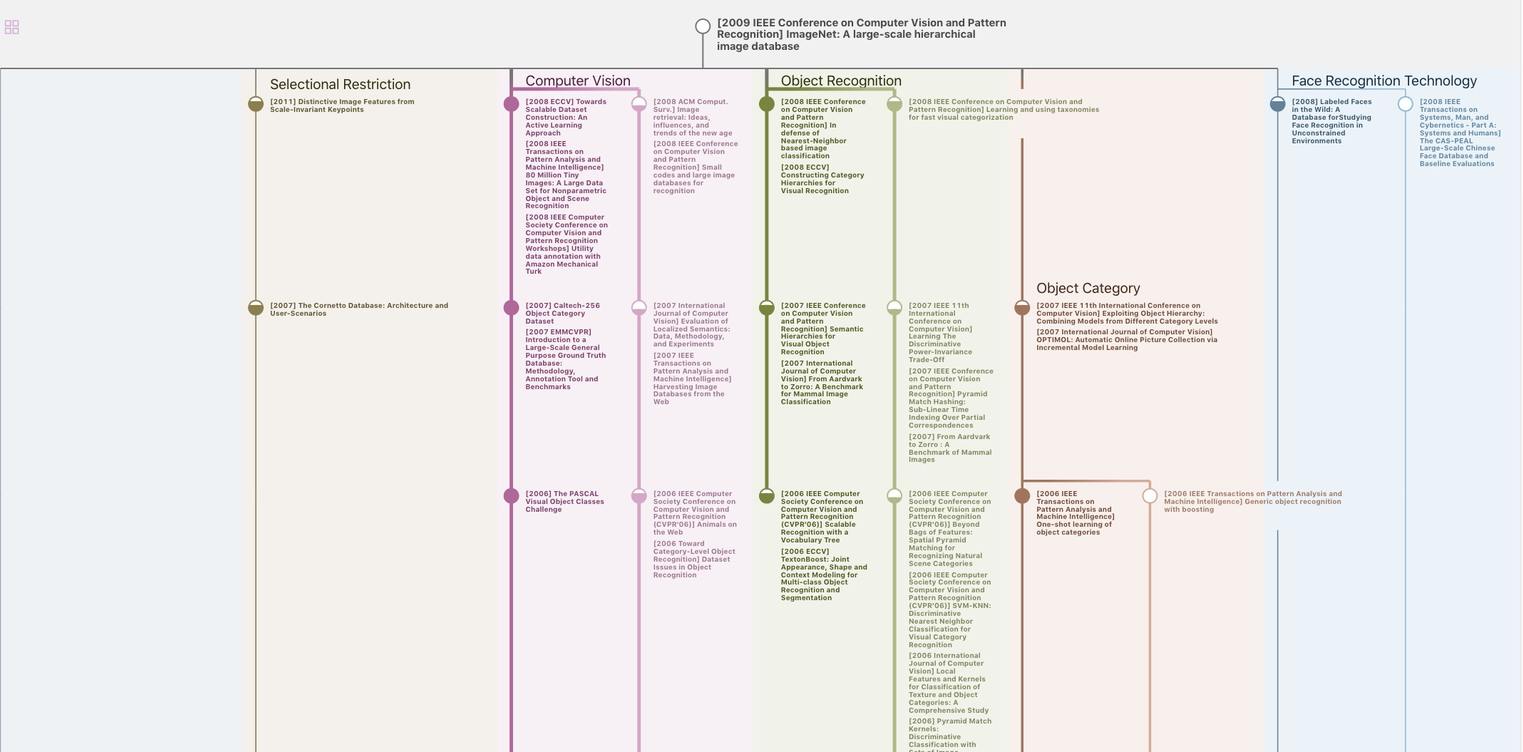
生成溯源树,研究论文发展脉络
Chat Paper
正在生成论文摘要