Camdar-Adv: Generating Adversarial Patches On 3d Object
INTERNATIONAL JOURNAL OF INTELLIGENT SYSTEMS(2021)
摘要
Deep neural network model is the core technology for sensors of the autonomous driving platform to perceive the external environment. Recent research have shown that it has a certain vulnerability. The artificial designed adversarial examples can make the DNN model output the wrong results. These adversarial examples not only exist in the digital world, but also in the physical world. At present, research on autonomous driving platform mainly focus on attacking a single sensor. In this paper, we introduce Camdar-adv, a method for generating image adversarial examples on three-dimensional (3D) objects, which could potentially lunch a multisensor attack toward the autonomous driving platforms. Specifically, with objects that can attack LiDAR sensors, a geometric transformation can be used to project their shape onto the two-dimensional plane. Adversarial perturbations against optical image sensor could be added to the surface of the adversarial 3D objects precisely without changing its geometry. Test results on the open-source autonomous driving data set KITTI show that Camdar-adv can generate adversarial samples for the state of the art object detection model. From a fixed viewpoint, our method can achieve an attack success rate over 99%.
更多查看译文
关键词
Adversarial example, autonmous driving, geometric transformation
AI 理解论文
溯源树
样例
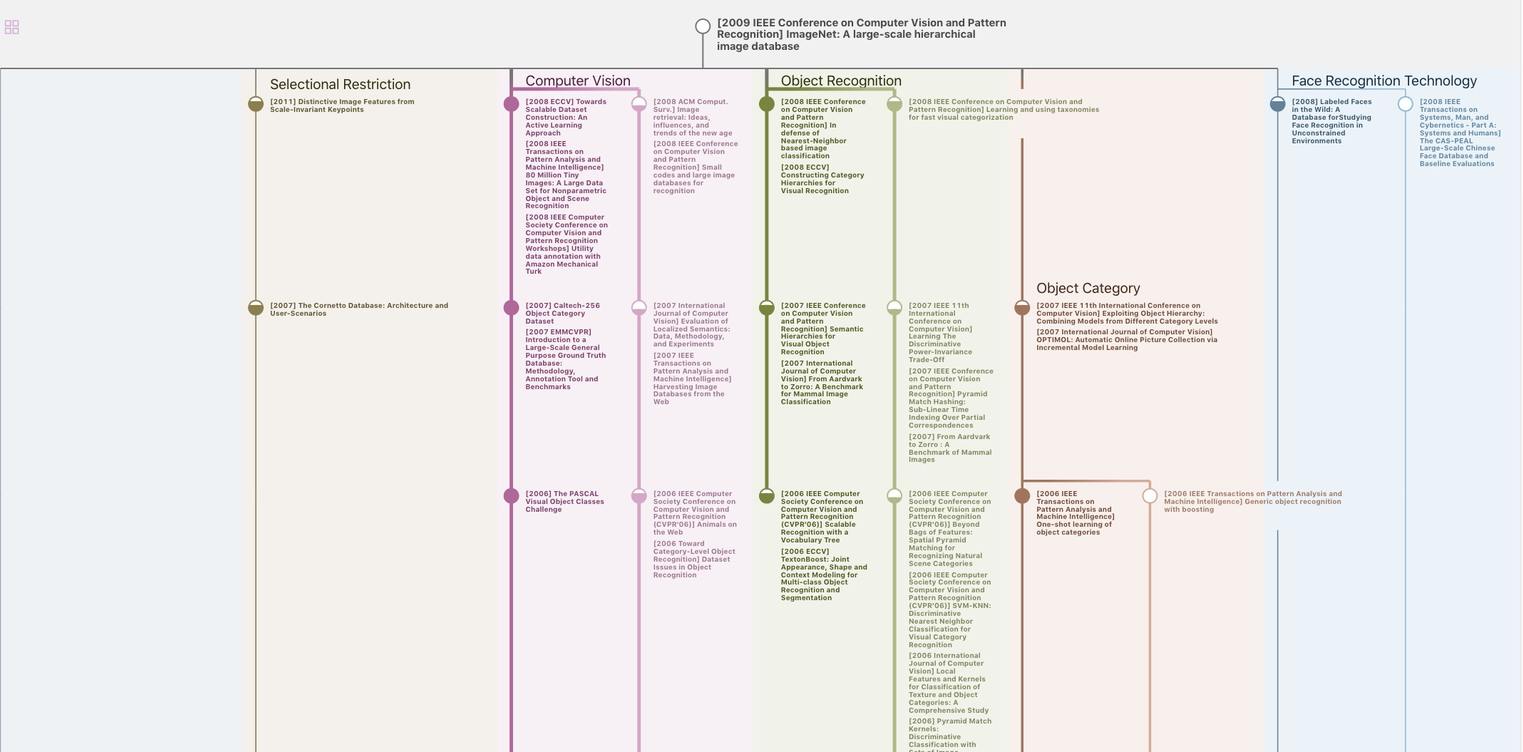
生成溯源树,研究论文发展脉络
Chat Paper
正在生成论文摘要