Evaluation of Random Forest for Complex Human Activity Recognition Using Wearable Sensors
2020 International Conference on Networking and Network Applications (NaNA)(2020)
摘要
The recognition of human activities plays a central role in bridging the gap between the raw sensor signals and high-level pervasive applications. However, the complex nature of human behavior presents a great challenge to the choice of representative features and a discriminant classification model and further makes it quite difficult to develop an accurate and robust activity recognizer. Random forest benefits from the idea of bootstrap and random feature sampling, while only preliminary experimentation for simple activity recognition has been previously reported and few studies, as far as we know, have comprehensively and systematically discussed its performance, especially in wearable-based complex activity recognition. The objective of this study is to present the first empirical results obtained with random forest and to compare its performance with other commonly used prediction models and dimensionality reduction methods. We conduct extensive comparative experiments on three benchmarked datasets. The results indicate the superiority of random forest-based activity recognizers over five individual classifiers, three ensemble models, one feature extractor as well as two feature selectors in terms of recognition performance across scenarios. Besides, the parameter sensitivity analysis recommends the default setting for the parameters and the time cost analysis indicates its applicability to practical applications.
更多查看译文
关键词
Complex activity recognition,ensemble learning,random forest,feature selection,generalization
AI 理解论文
溯源树
样例
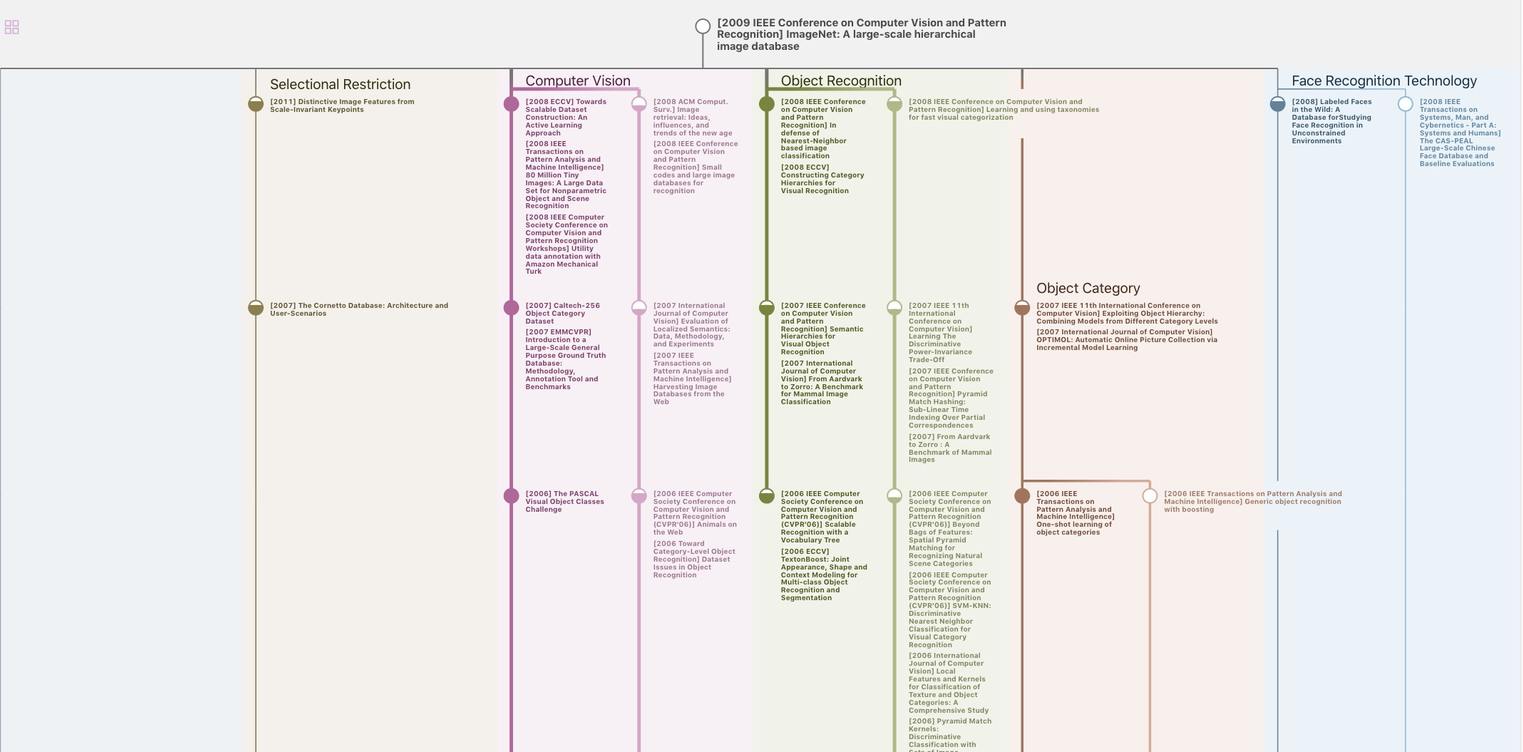
生成溯源树,研究论文发展脉络
Chat Paper
正在生成论文摘要