Anterior Mediastinal Lesion Segmentation Based On Two-Stage 3d Resunet With Attention Gates And Lung Segmentation
FRONTIERS IN ONCOLOGY(2021)
摘要
ObjectivesAnterior mediastinal disease is a common disease in the chest. Computed tomography (CT), as an important imaging technology, is widely used in the diagnosis of mediastinal diseases. Doctors find it difficult to distinguish lesions in CT images because of image artifact, intensity inhomogeneity, and their similarity with other tissues. Direct segmentation of lesions can provide doctors a method to better subtract the features of the lesions, thereby improving the accuracy of diagnosis.MethodAs the trend of image processing technology, deep learning is more accurate in image segmentation than traditional methods. We employ a two-stage 3D ResUNet network combined with lung segmentation to segment CT images. Given that the mediastinum is between the two lungs, the original image is clipped through the lung mask to remove some noises that may affect the segmentation of the lesion. To capture the feature of the lesions, we design a two-stage network structure. In the first stage, the features of the lesion are learned from the low-resolution downsampled image, and the segmentation results under a rough scale are obtained. The results are concatenated with the original image and encoded into the second stage to capture more accurate segmentation information from the image. In addition, attention gates are introduced in the upsampling of the network, and these gates can focus on the lesion and play a role in filtering the features. The proposed method has achieved good results in the segmentation of the anterior mediastinal.ResultsThe proposed method was verified on 230 patients, and the anterior mediastinal lesions were well segmented. The average Dice coefficient reached 87.73%. Compared with the model without lung segmentation, the model with lung segmentation greatly improved the accuracy of lesion segmentation by approximately 9%. The addition of attention gates slightly improved the segmentation accuracy.ConclusionThe proposed automatic segmentation method has achieved good results in clinical data. In clinical application, automatic segmentation of lesions can assist doctors in the diagnosis of diseases and may facilitate the automated diagnosis of illnesses in the future.
更多查看译文
关键词
anterior mediastinal lesion segmentation, deep learning, two-stage 3D ResUNet, attention gates, lung segmentation model
AI 理解论文
溯源树
样例
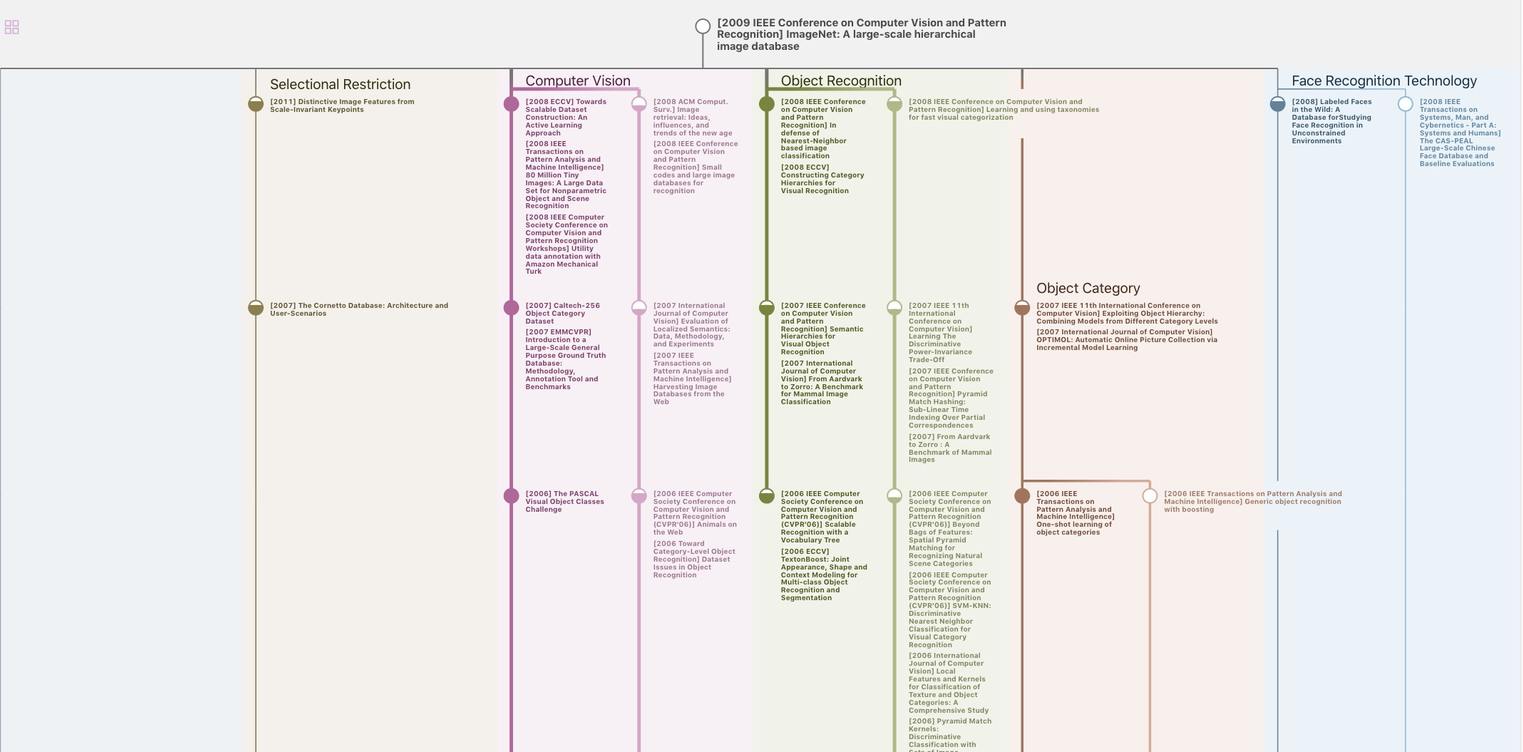
生成溯源树,研究论文发展脉络
Chat Paper
正在生成论文摘要