Attend to Chords: Improving Harmonic Analysis of Symbolic Music Using Transformer-Based Models.
Trans. Int. Soc. Music. Inf. Retr.(2021)
摘要
Automatic chord recognition (ACR) has long been a topic of interest in the field of Music Information Retrieval (MIR), due to not only its commercial applications, but also its support for advanced music analysis. While a lot of ACR-related work deals with audio data, ACR from symbolic music has received less attention. In addition, conventional ACR systems specify chords in a key-dependent way (usually with the root note and the chord quality) and hence are unable to reveal the high-level patterns and harmonic structures. These issues hinder the developments of music analysis and music generation via ACR systems. With the success of deep learning, it is viable to build a symbolic ACR system using a more comprehensive chord vocabulary such as functional harmony. Recently, two advanced models, namely the Bi-directional Transformer for Chord Recognition (BTC) and the Harmony Transformer (HT), introduced for the first time the multi-head attention mechanism to ACR, showing the great capability of the attention mechanism to improve the performance of ACR. In this paper, we systematically study the performance of the BTC and the HT in terms of symbolic ACR, and propose an improved model. Experiments on conventional ACR and advanced functional harmony recognition indicate that the HT has the potential to surpass the BTC, especially in terms of chord segmentation quality. Also the overall performance of the HT is further improved by enhancing the learning of local context and positional information.
更多查看译文
关键词
automatic chord recognition,functional harmony recognition,symbolic music,transformer,multi-head attention,chord segmentation
AI 理解论文
溯源树
样例
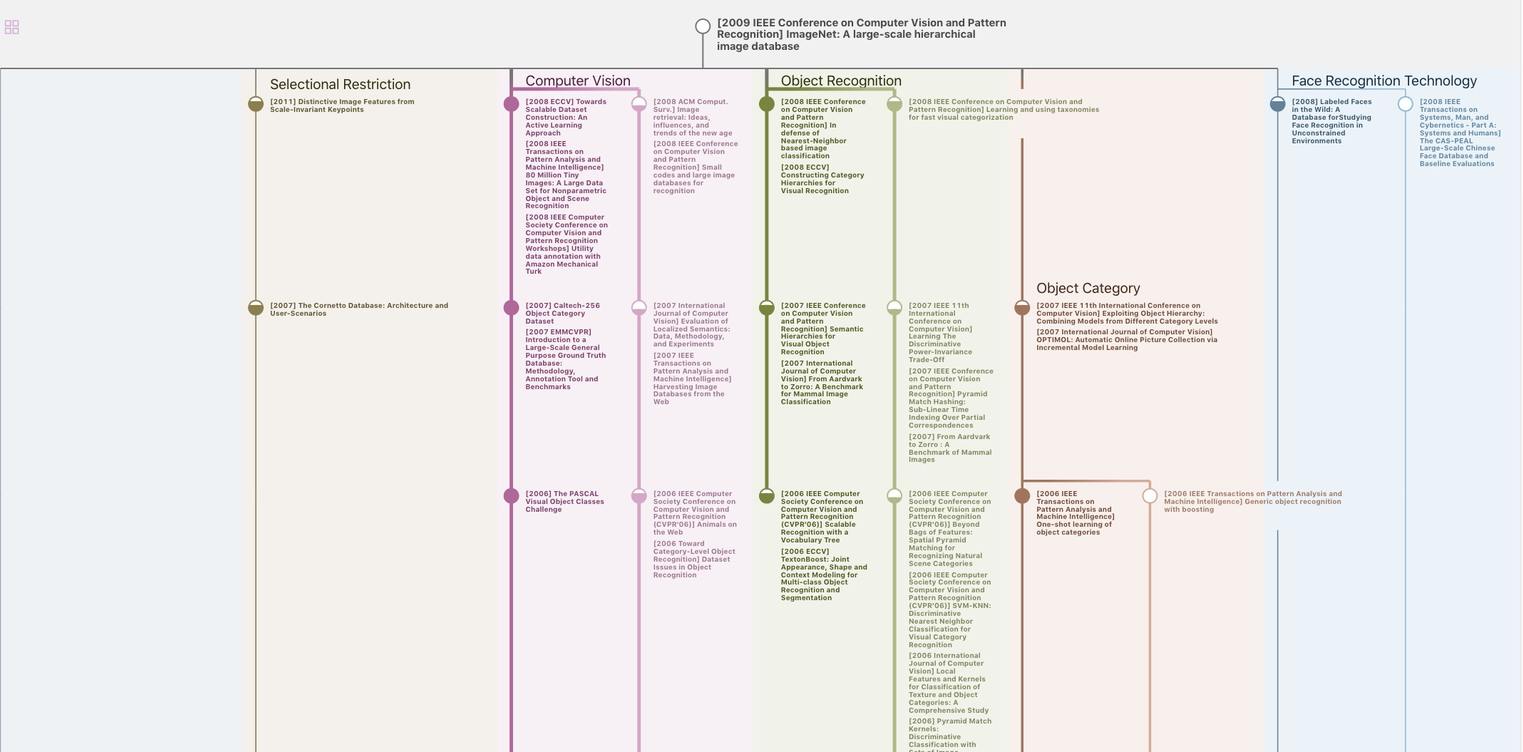
生成溯源树,研究论文发展脉络
Chat Paper
正在生成论文摘要