Development And Clinical Validation Of A Seven-Gene Prognostic Signature Based On Multiple Machine Learning Algorithms In Kidney Cancer
CELL TRANSPLANTATION(2021)
摘要
About a third of patients with kidney cancer experience recurrence or cancer-related progression. Clinically, kidney cancer prognoses may be quite different, even in patients with kidney cancer at the same clinical stage. Therefore, there is an urgent need to screen for kidney cancer prognosis biomarkers. Differentially expressed genes (DEGs) were identified using kidney cancer RNA sequencing data from the Gene Expression Omnibus (GEO) database. Biomarkers were screened using random forest (RF) and support vector machine (SVM) models, and a multigene signature was constructed using the least absolute shrinkage and selection operator (LASSO) regression analysis. Univariate and multivariate Cox regression analyses were performed to explore the relationships between clinical features and prognosis. Finally, the reliability and clinical applicability of the model were validated, and relationships with biological pathways were identified. Western blots were also performed to evaluate gene expression. A total of 50 DEGs were obtained by intersecting the RF and SVM models. A seven-gene signature (RNASET2, EZH2, FXYD5, KIF18A, NAT8, CDCA7, and WNT7B) was constructed by LASSO regression. Univariate and multivariate Cox regression analyses showed that the seven-gene signature was an independent prognostic factor for kidney cancer. Finally, a predictive nomogram was established in The Cancer Genome Atlas (TCGA) cohort and validated internally. In tumor tissue, RNASET2 and FXYD5 were highly expressed and NAT8 was lowly expressed at the protein and transcription levels. This model could complement the clinicopathological characteristics of kidney cancer and promote the personalized management of patients with kidney cancer.
更多查看译文
关键词
kidney cancer, SVM, RF, LASSO, gene signature, prognosis
AI 理解论文
溯源树
样例
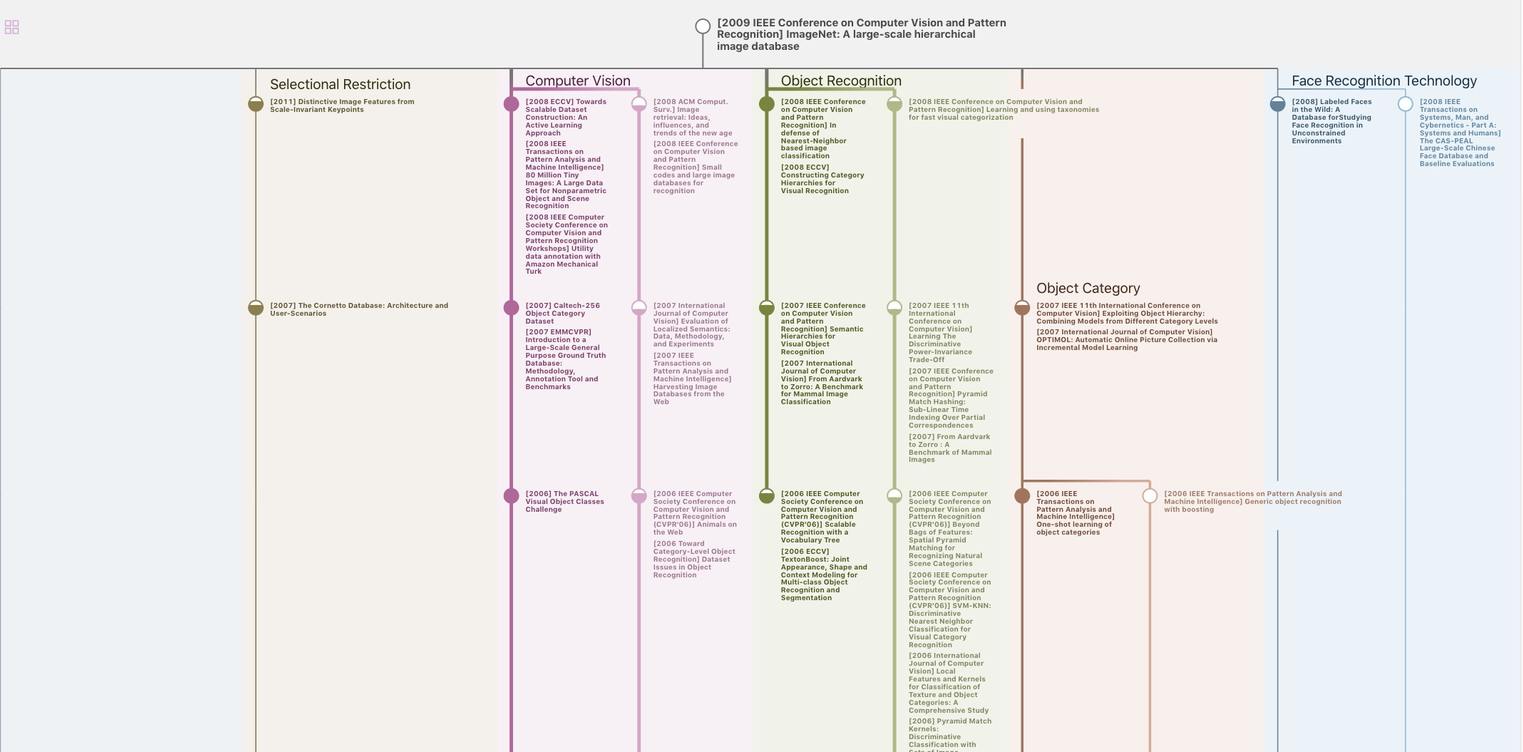
生成溯源树,研究论文发展脉络
Chat Paper
正在生成论文摘要