MASKCYCLEGAN-VC: LEARNING NON-PARALLEL VOICE CONVERSION WITH FILLING IN FRAMES
2021 IEEE INTERNATIONAL CONFERENCE ON ACOUSTICS, SPEECH AND SIGNAL PROCESSING (ICASSP 2021)(2021)
摘要
Non-parallel voice conversion (VC) is a technique for training voice converters without a parallel corpus. Cycle-consistent adversarial network-based VCs (CycleGAN-VC and CycleGAN-VC2) are widely accepted as benchmark methods. However, owing to their insufficient ability to grasp time-frequency structures, their application is limited to mel-cepstrum conversion and not mel-spectrogram conversion despite recent advances in mel-spectrogram vocoders. To overcome this, CycleGAN-VC3, an improved variant of CycleGAN-VC2 that incorporates an additional module called time-frequency adaptive normalization (TFAN), has been proposed. However, an increase in the number of learned parameters is imposed. As an alternative, we propose MaskCycleGAN-VC, which is another extension of CycleGAN-VC2 and is trained using a novel auxiliary task called filling in frames (FIF). With FIF, we apply a temporal mask to the input mel-spectrogram and encourage the converter to fill in missing frames based on surrounding frames. This task allows the converter to learn time-frequency structures in a self-supervised manner and eliminates the need for an additional module such as TFAN. A subjective evaluation of the naturalness and speaker similarity showed that MaskCycleGAN-VC outperformed both CycleGAN-VC2 and CycleGAN-VC3 with a model size similar to that of CycleGAN-VC2.(1)
更多查看译文
关键词
Voice conversion (VC), non-parallel VC, generative adversarial networks (GANs), CycleGAN-VC, mel-spectrogram conversion
AI 理解论文
溯源树
样例
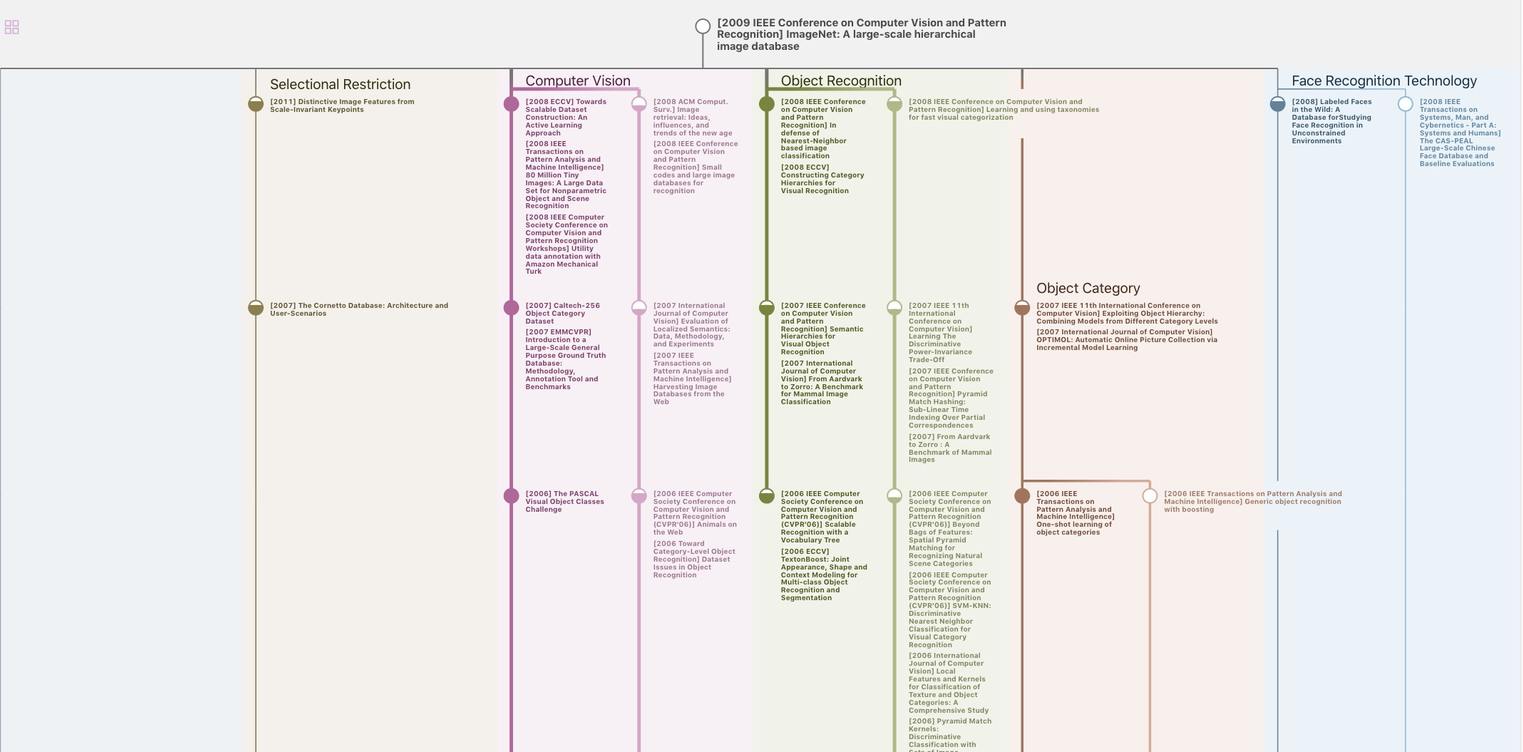
生成溯源树,研究论文发展脉络
Chat Paper
正在生成论文摘要