Time-Series Imputation with Wasserstein Interpolation for Optimal Look-Ahead-Bias and Variance Tradeoff
arxiv(2023)
摘要
Missing time-series data is a prevalent practical problem. Imputation methods in time-series data often are applied to the full panel data with the purpose of training a model for a downstream out-of-sample task. For example, in finance, imputation of missing returns may be applied prior to training a portfolio optimization model. Unfortunately, this practice may result in a look-ahead-bias in the future performance on the downstream task. There is an inherent trade-off between the look-ahead-bias of using the full data set for imputation and the larger variance in the imputation from using only the training data. By connecting layers of information revealed in time, we propose a Bayesian posterior consensus distribution which optimally controls the variance and look-ahead-bias trade-off in the imputation. We demonstrate the benefit of our methodology both in synthetic and real financial data.
更多查看译文
关键词
wasserstein interpolation,imputation,variance tradeoff,time-series,look-ahead-bias
AI 理解论文
溯源树
样例
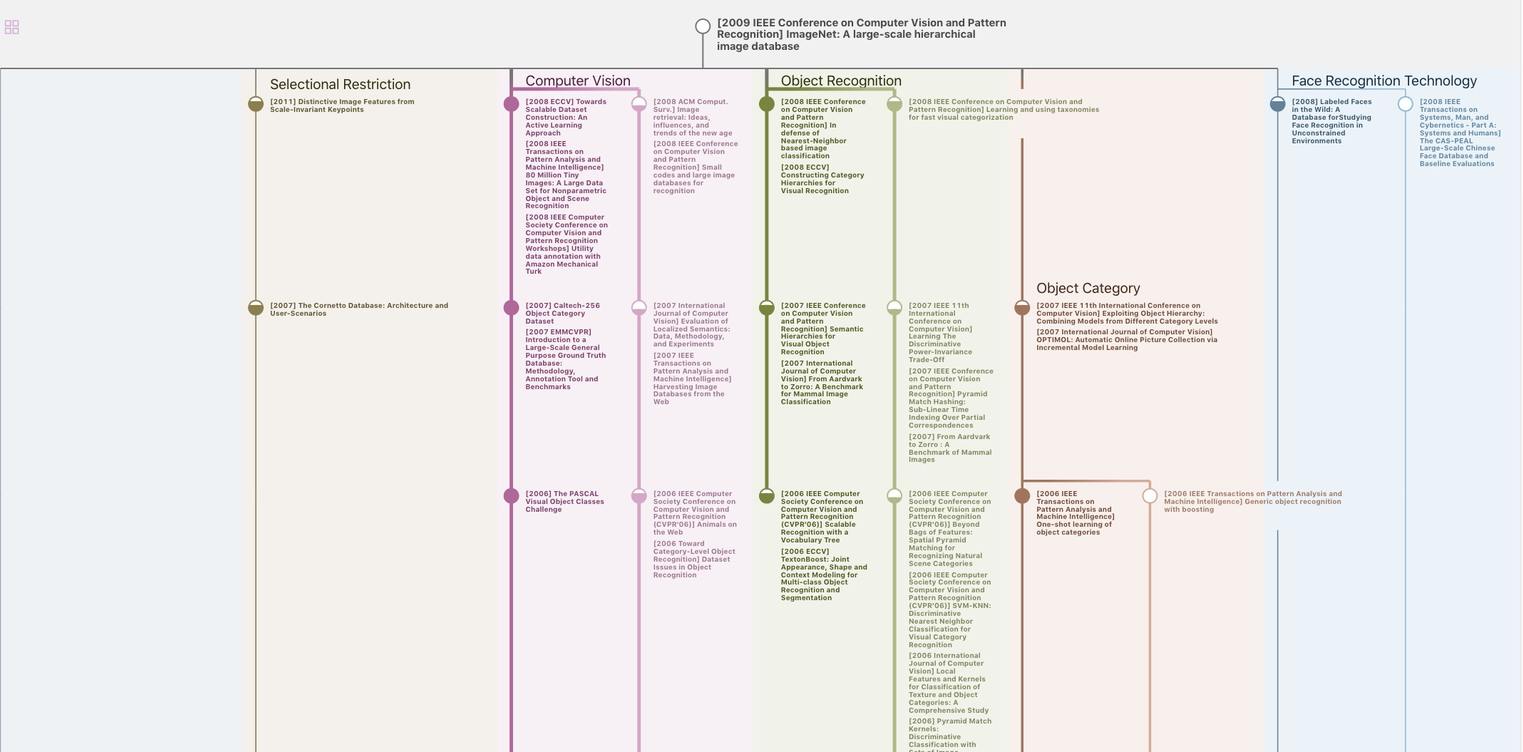
生成溯源树,研究论文发展脉络
Chat Paper
正在生成论文摘要