Efficient computational algorithms for approximate optimal designs
JOURNAL OF STATISTICAL COMPUTATION AND SIMULATION(2022)
摘要
In this paper, we propose two simple yet efficient computational algorithms to obtain approximate optimal designs for multi-dimensional linear regression on a large variety of design spaces. We focus on the two commonly used optimal criteria, D- and A-optimal criteria. For D-optimality, we provide an alternative proof for the monotonic convergence for D-optimal criterion and propose an efficient computational algorithm to obtain the approximate D-optimal design. We further show that the proposed algorithm converges to the D-optimal design and then proves that the approximate D-optimal design converges to the continuous D-optimal design under certain conditions. For A-optimality, we provide an efficient algorithm to obtain approximate A-optimal design and conjecture the monotonicity of the proposed algorithm. Numerical comparisons suggest that the proposed algorithms perform well and they are comparable or superior to some existing algorithms.
更多查看译文
关键词
Approximate experimental design, D-optimal, A-optimal, Regression model
AI 理解论文
溯源树
样例
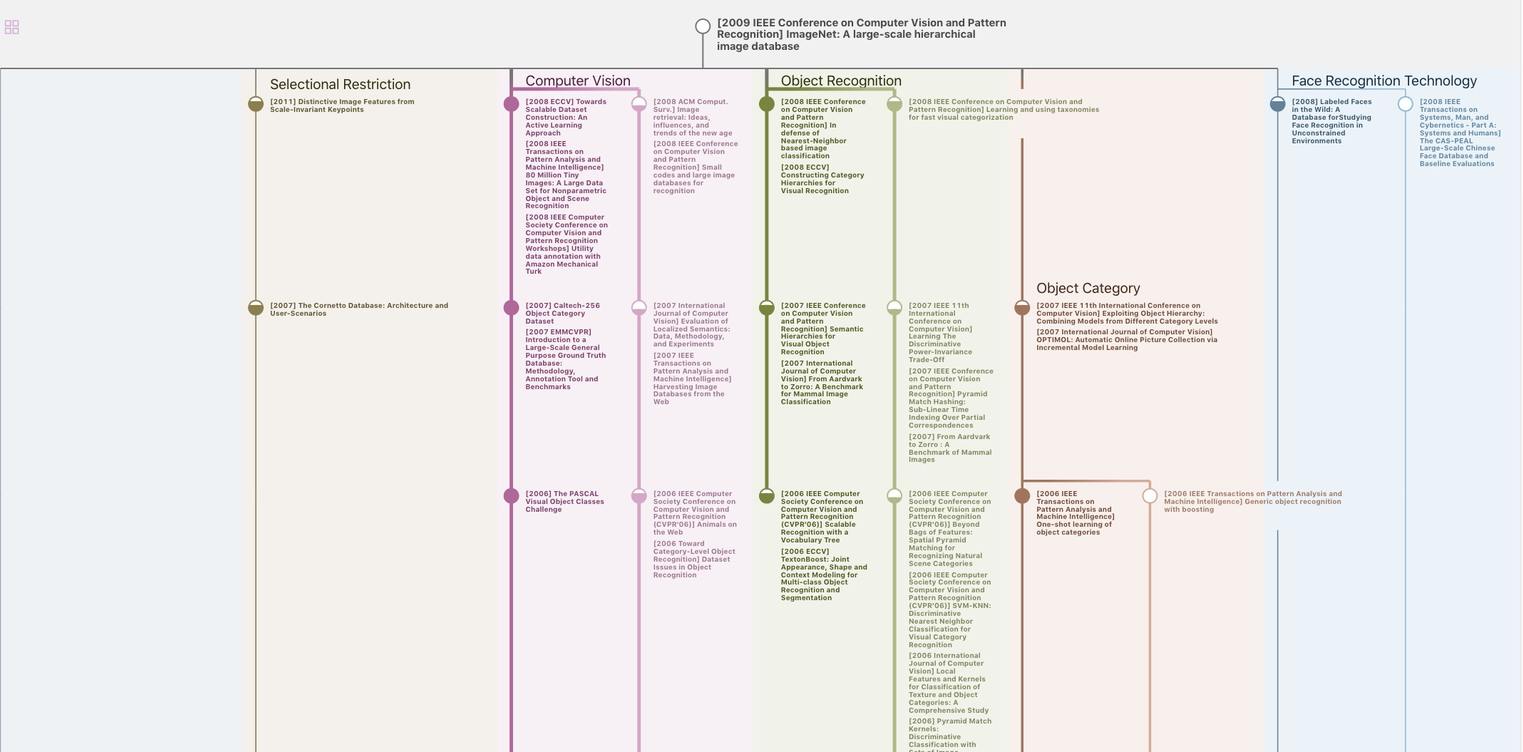
生成溯源树,研究论文发展脉络
Chat Paper
正在生成论文摘要