MULTI-LEVEL ADAPTIVE REGION OF INTEREST AND GRAPH LEARNING FOR FACIAL ACTION UNIT RECOGNITION
2021 IEEE INTERNATIONAL CONFERENCE ON ACOUSTICS, SPEECH AND SIGNAL PROCESSING (ICASSP 2021)(2021)
摘要
In facial action unit (AU) recognition tasks, regional feature learning and AU relation modeling are two effective aspects which are worth exploring. However, the limited representation capacity of regional features makes it difficult for relation models to embed AU relationship knowledge. In this paper, we propose a novel multi-level adaptive ROI and graph learning (MARGL) framework to tackle this problem. Specifically, an adaptive ROI learning module is designed to automatically adjust the location and size of the predefined AU regions. Meanwhile, besides relationship between AUs, there exists strong relevance between regional features across multiple levels of the backbone network as level-wise features focus on different aspects of representation. In order to incorporate the intra-level AU relation and inter-level AU regional relevance simultaneously, a multi-level AU relation graph is constructed and graph convolution is performed to further enhance AU regional features of each level. Experiments on BP4D and DISFA demonstrate the proposed MARGL significantly outperforms the previous state-of-the-art methods.
更多查看译文
关键词
AU Recognition, Adaptive Regional Feature Learning, Multi-Level Graph Learning
AI 理解论文
溯源树
样例
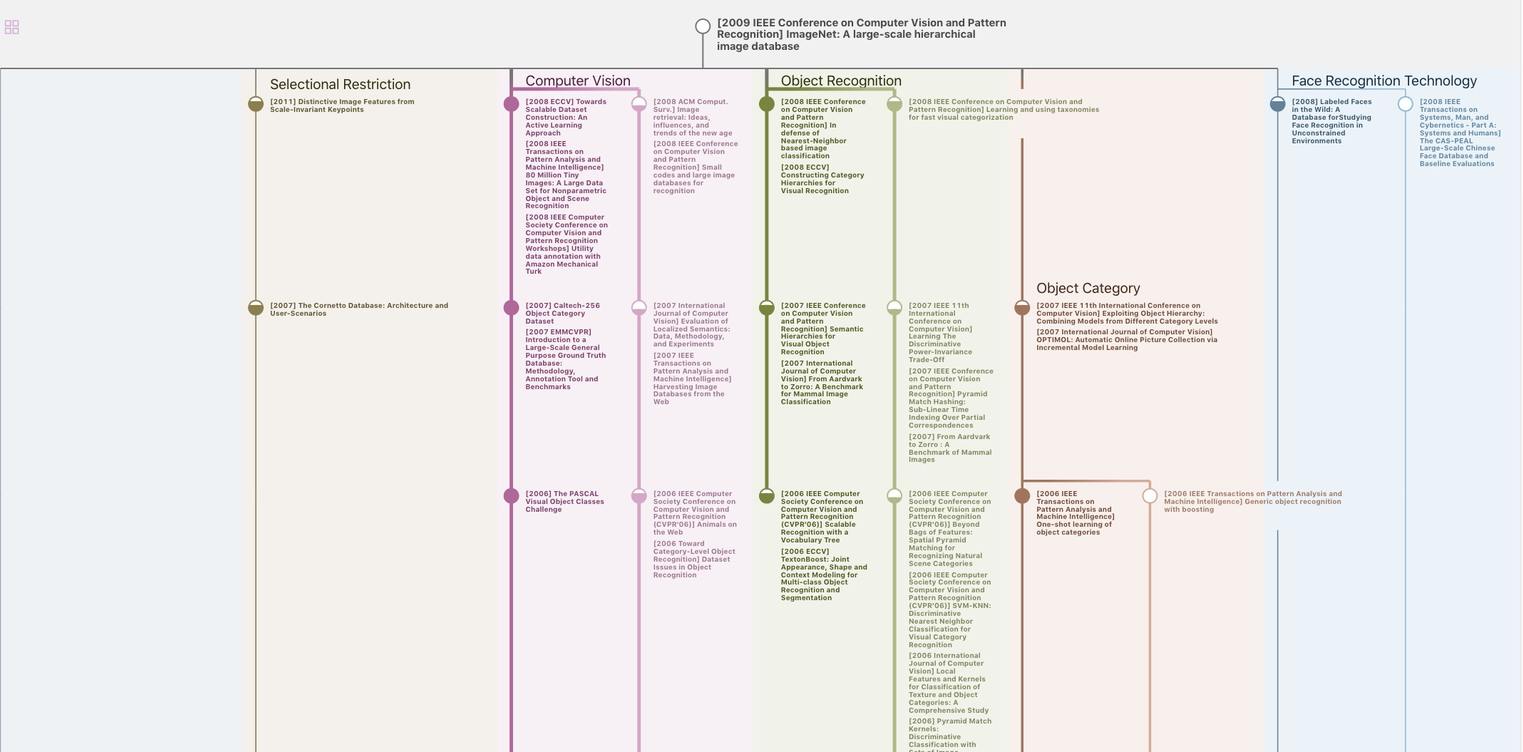
生成溯源树,研究论文发展脉络
Chat Paper
正在生成论文摘要