Channel-Quality Reporting Enabled By Machine Learning In Non-Stationary Environments
2020 IEEE 92ND VEHICULAR TECHNOLOGY CONFERENCE (VTC2020-FALL)(2020)
摘要
In this paper, we propose a novel channel quality reporting approach for cellular communication systems. The proposed approach features a differential coding scheme in stationary propagation conditions and a detector of non-stationary propagation conditions, which further triggers a channel-quality predictor for the non-stationary environment based on a machine learning method. In particular, the machine learning engine learns about the specific large variations of the channel quality by collecting signaling information from mobile terminals in a given region. Our simulations in a controlled urban environment with vehicular users show that the proposed solution can effectively replace the 4-bit channel-quality reporting scheme of LTE and NR standards with a 2-bit one, providing correct channel-quality indication in non-stationary conditions with high probability.
更多查看译文
关键词
LTE, NR, CQI reporting, channel prediction, non-stationary propagation, machine learning
AI 理解论文
溯源树
样例
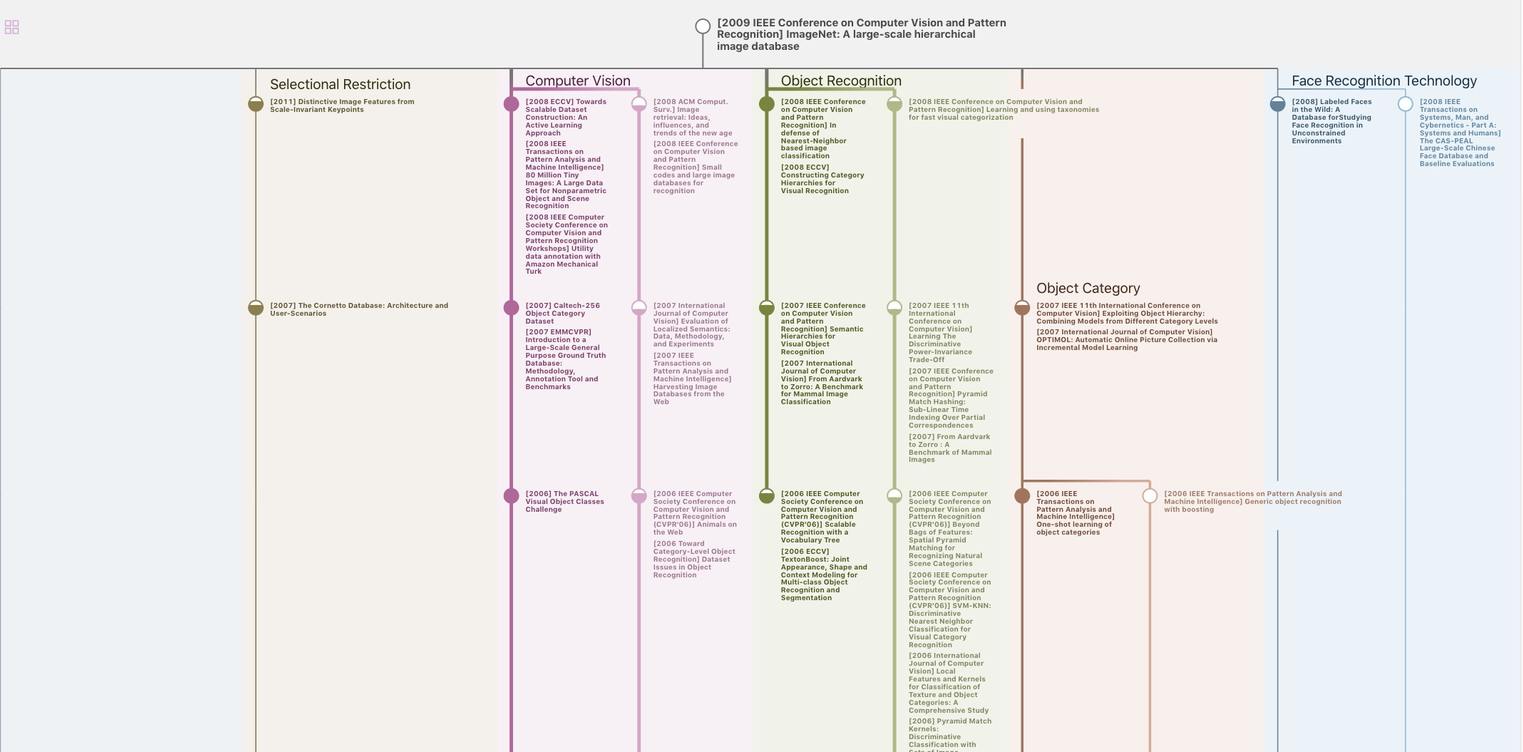
生成溯源树,研究论文发展脉络
Chat Paper
正在生成论文摘要