Uncertainty-Aware Ran Slicing Via Machine Learning Predictions In Next-Generation Networks
2020 IEEE 92ND VEHICULAR TECHNOLOGY CONFERENCE (VTC2020-FALL)(2020)
摘要
Network slicing enables 5G network operators to offer diverse services in the form of end-to-end isolated slices, over shared physical infrastructure. Wireless service providers are facing the need to plan and rapidly evolve their slices configuration to meet the varied tenants' demand. Network slicing unfolds a new market dimension to the infrastructure providers as well as to the tenants, who may acquire a network slice from the infrastructure provider to deliver a specific service to their respective subscribers. In this new context, there is a growing need for new network resource allocation algorithms to capture such proposition. This paper addresses this problem by introducing a family of online algorithms with the aim to (i) minimize tenants spectrum allocation costs, (ii) maximize radio resource utilization and (iii) ensure that the service level agreements (SLAs) provided to tenants are satisfied. We focus on improving the performance of prediction-based decisions that are made by a tenant when prediction models lack the desired accuracy. Our evaluations show that the proposed probabilistic approach can automatically adapt to prediction error variance, while largely improving network slice acquisition cost and resource utilization.
更多查看译文
关键词
RAN Slicing, spectrum management, 5G, traffic forecasting, machine learning, next generation wireless networks
AI 理解论文
溯源树
样例
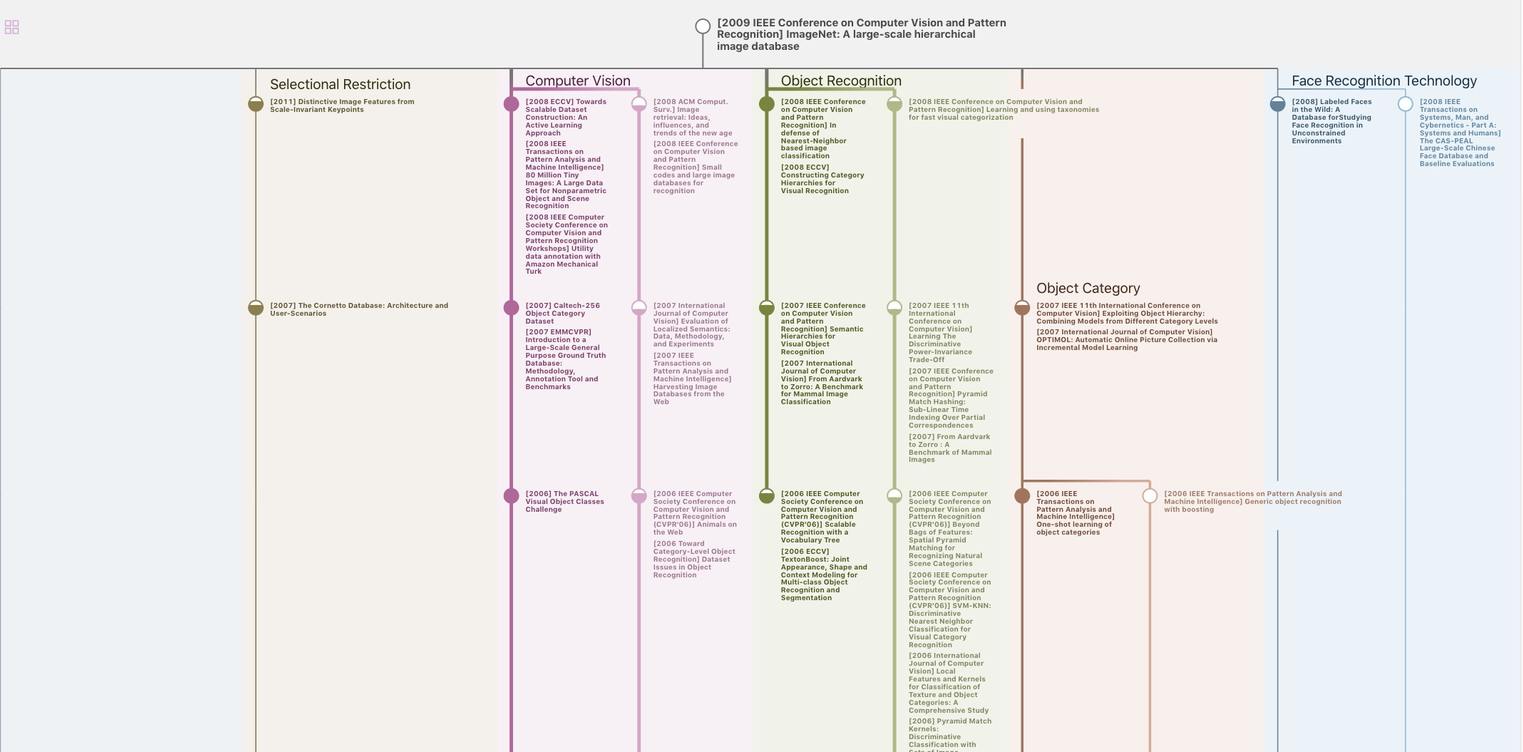
生成溯源树,研究论文发展脉络
Chat Paper
正在生成论文摘要