Knowing Where To Leverage: Context-Aware Graph Convolutional Network With An Adaptive Fusion Layer For Contextual Spoken Language Understanding
IEEE-ACM TRANSACTIONS ON AUDIO SPEECH AND LANGUAGE PROCESSING(2021)
摘要
Spoken language understanding (SLU) systems aim to understand users' utterance, which is a key component of task-oriented dialogue systems. In this paper, we focus on improving the contextual SLU. The contextual SLU systems mainly focus on how to effectively incorporate dialog context information (contextual information). The existing approaches all use the same contextual information to guide slot filling at all tokens, which may inject the irrelevant information and result in ambiguity. To tackle this problem, we propose a context-aware graph convolutional network (GCN) with an adaptive fusion layer for contextual SLU. The context-aware GCN is proposed to automatically aggregate the contextual information, which frees our model from the manually designed heuristic aggregation function. Meanwhile, an adaptive fusion layer is applied at each token to dynamically incorporate relevant contextual information, which achieves a fine-grained contextual information transfer to guide the token-level slot filling. Experiments on the Simulated Dialog Dataset show that our model achieves state-of-the-art performance and outperforms other previous methods by a large margin (+3.67% on Sim-R, +4.18% on Sim-M and +3.75% on Overall dataset). In addition, we explore and analyze the pre-trained model (i.e., BERT) in our framework. We show that incorporating BERT brings a large improvement in low-resource setting.
更多查看译文
关键词
Context modeling, Task analysis, Speech processing, Predictive models, Bit error rate, Adaptive systems, Motion pictures, Contextual spoken langauge understanding, task-oriented dialog systems, graph convolutional network, BERT
AI 理解论文
溯源树
样例
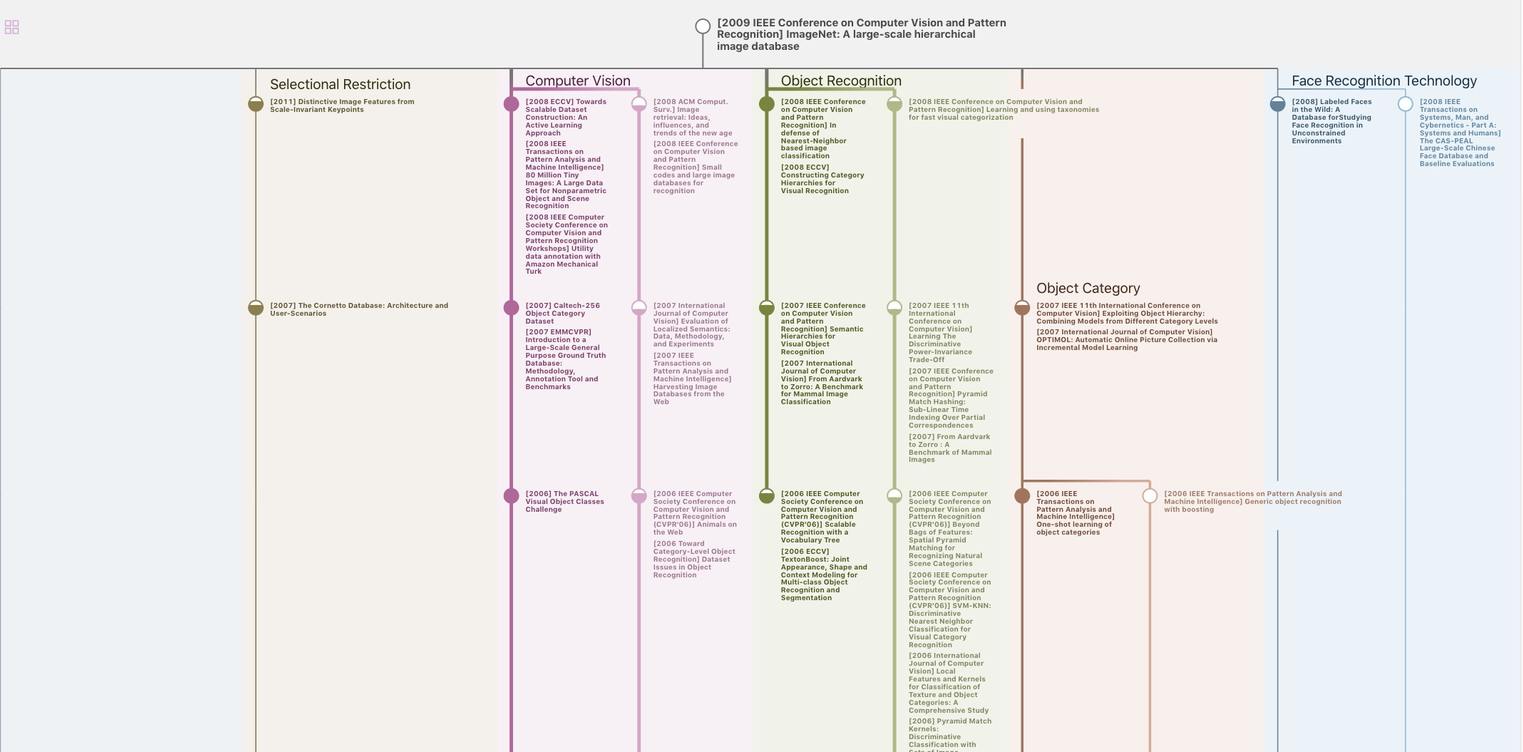
生成溯源树,研究论文发展脉络
Chat Paper
正在生成论文摘要