Cramer-Rao Lower Bound Analysis Of Data Fusion For Fingerprinting Localization In Non-Line-Of-Sight Environments
IEEE ACCESS(2021)
摘要
This paper proposes a novel framework for analyzing the localization accuracy of data fusion for fingerprinting approaches in non-line-of-sight (NLOS) environments. Using simulation data generated for two very different NLOS environments (a suburban area of 3.3km x 3.3km in Santa Clara, California, and a mountainous area of 11.4km x 11.4km in the Caspian region), we establish novel channel models for measurement differences of three data types (received signal strength indicator (RSS), time of arrival (TOA) and direction of arrival (DOA)) at K neighboring nodes of an arbitrary node. The crucial point is that the modeling errors for each of the three data types are shown to be jointly Gaussian distributed. Based on these measurement difference models, Cramer-Rao Lower Bound (CRLB) is used as a benchmark to evaluate K-nearest neighbor (KNN) andWeighted K-Nearest Neighbor (WKNN). It is shown that the proposed CRLB analyses can be employed to evaluate fingerprinting systems with various designs (such as different data types and fusion options) and different configurations (such as densities of reference nodes and numbers of anchor nodes) in diverse NLOS environments(such as suburban and mountainous regions).
更多查看译文
关键词
Localization, NLOS, 5G, weighted K-nearest neighborhood, RSS, TOA, DOA
AI 理解论文
溯源树
样例
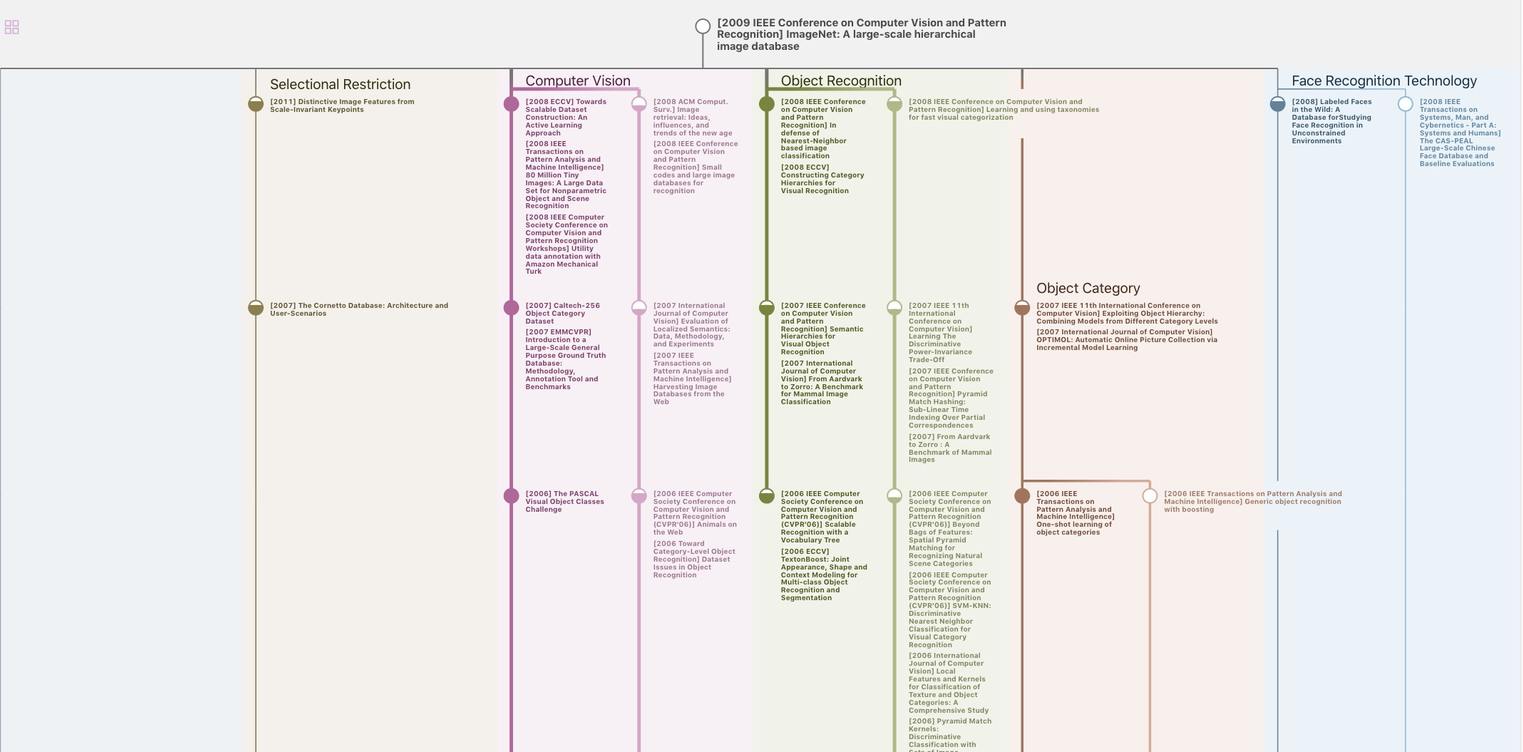
生成溯源树,研究论文发展脉络
Chat Paper
正在生成论文摘要