The Effectiveness Of Ensemble-Neural Network Techniques To Predict Peak Uplift Resistance Of Buried Pipes In Reinforced Sand
APPLIED SCIENCES-BASEL(2021)
摘要
Buried pipes are extensively used for oil transportation from offshore platforms. Under unfavorable loading combinations, the pipe's uplift resistance may be exceeded, which may result in excessive deformations and significant disruptions. This paper presents findings from a series of small-scale tests performed on pipes buried in geogrid-reinforced sands, with the measured peak uplift resistance being used to calibrate advanced numerical models employing neural networks. Multilayer perceptron (MLP) and Radial Basis Function (RBF) primary structure types have been used to train two neural network models, which were then further developed using bagging and boosting ensemble techniques. Correlation coefficients in excess of 0.954 between the measured and predicted peak uplift resistance have been achieved. The results show that the design of pipelines can be significantly improved using the proposed novel, reliable and robust soft computing models.
更多查看译文
关键词
maximum uplift resistance, pipeline, ensemble neural network, neural network, reinforced soil
AI 理解论文
溯源树
样例
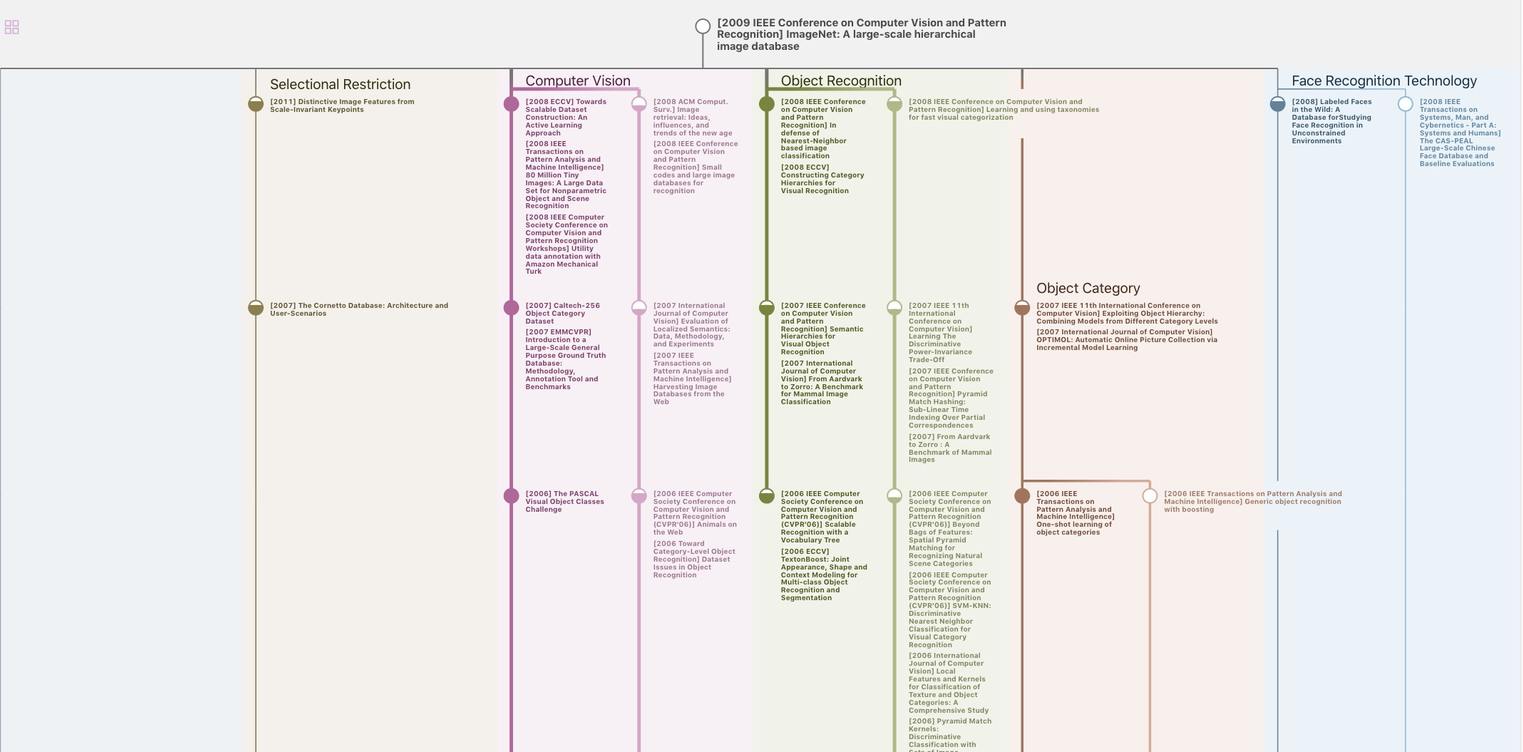
生成溯源树,研究论文发展脉络
Chat Paper
正在生成论文摘要