Real-time semantic segmentation via sequential knowledge distillation.
Neurocomputing(2021)
摘要
Deep model-based semantic segmentation has received ever increasing research focus in recent years. However, due to the complex model architectures, existing works are still unable to achieve high accuracy in real-time applications. In this paper, we propose a novel Sequential Prediction Network (termed SPNet) to seek a better trade-off between accuracy and efficiency. SPNet is also an end-to-end encoder-decoder architecture, which introduces a sequential prediction method to spread the contextual information from the low-level layers to the high-level layers. Besides, the proposed method is equipped with a stream Spatial Semantic and Edge Loss (termed SEL) and an adversarial network at multiple resolutions, which greatly improves the segmentation accuracy with a negligible increase in computation cost. To further utilize the extra unlabeled data, we present a knowledge distillation scheme to distill the structured knowledge from cumbersome to compact networks. Without using any pre-trained model, our method achieves state-of-the-art performance among exiting real-time segmentation models on several challenging datasets. Impressively, on the Cityscapes test dataset, it obtains 75.8% mIoU at a speed of 61.2 FPS.
更多查看译文
关键词
Semantic segmentation,Spatial refinement constraint,Adversarial network,Knowledge distillation
AI 理解论文
溯源树
样例
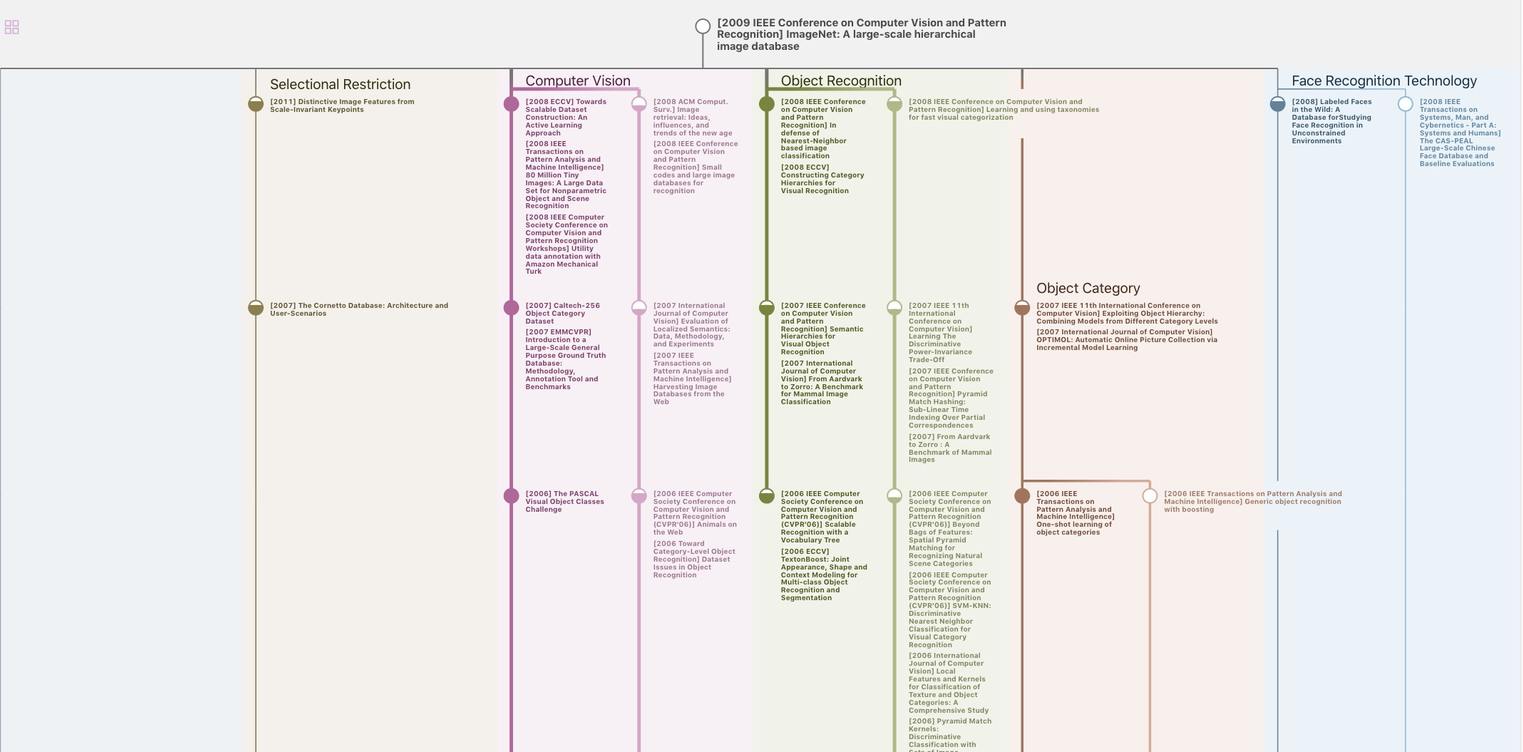
生成溯源树,研究论文发展脉络
Chat Paper
正在生成论文摘要