Do Missing Values Influence Outcomes in a Cross-sectional Mail Survey?
Mayo Clinic Proceedings: Innovations, Quality & Outcomes(2021)
摘要
Objective
To determine the effects of missing and inconsistent data on a weight management mail survey results.
Patients and Methods
Weight management surveys were sent to 5000 overweight and obese individuals in the Learning Health System Network. Survey information was collected between October 27, 2017, and March 1, 2018. Some participants reported body mass index (BMI) values inconsistent with the intended overweight and obese sampling cohort. Analyses were performed after excluding these surveys and also performed again after setting these low BMI values to missing. Models were run after imputing missing values using expectation-maximization, Markov chain Monte Carlo, random forest imputation, multivariate imputation by chained equations, and multiple imputation and replacing missing BMI values with the minimum, maximum, mean, or median of the known BMI values.
Results
Of 2799 surveys, 222 (8%) had missing BMI values and 155 (6%) reported invalid BMI values. Overall, 725 of these 2799 surveys (26%) were missing at least 1 variable that was essential to the main analyses. Different imputation methods consistently found that BMI was related to age, sex, race, marital status, and education. Patients with a BMI of 35.0 kg/m2 or greater were more likely to feel judged because of their weight, and patients with a BMI of 40.0 kg/m2 or greater were more likely to feel they were not always treated with respect and treated as an equal.
Conclusion
Analyses using different imputation methods were consistent with the original published results. Missing data likely did not affect the study results.
更多查看译文
关键词
BMI,MAR,MCAR,MCMC,MNAR,OR
AI 理解论文
溯源树
样例
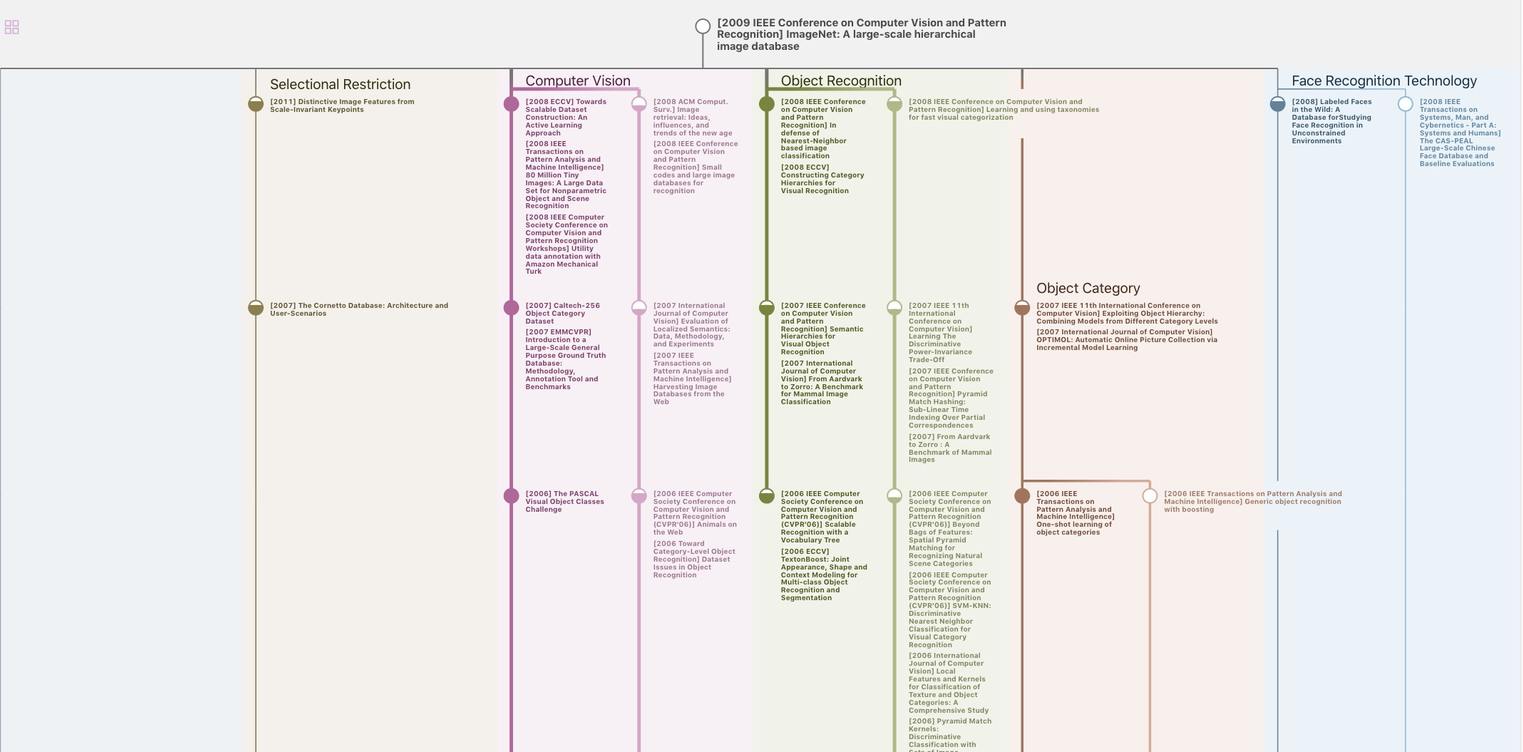
生成溯源树,研究论文发展脉络
Chat Paper
正在生成论文摘要