The Power of (Non-)Linear Shrinking: A Review and Guide to Covariance Matrix Estimation
Journal of Financial Econometrics(2020)
摘要
Many econometric and data-science applications require a reliable estimate of the covariance matrix, such as Markowitz's portfolio selection. When the number of variables is of the same magnitude as the number of observations, this constitutes a difficult estimation problem; the sample covariance matrix certainly will not do. In this article, we review our work in this area, going back 15+ years. We have promoted various shrinkage estimators, which can be classified into linear and nonlinear. Linear shrinkage is simpler to understand, to derive, and to implement. But nonlinear shrinkage can deliver another level of performance improvement, especially if overlaid with stylized facts such as time-varying co-volatility or factor models.
更多查看译文
关键词
dynamic conditional correlations, factor models, large-dimensional asymptotics, Markowitz's portfolio selection, rotation equivariance
AI 理解论文
溯源树
样例
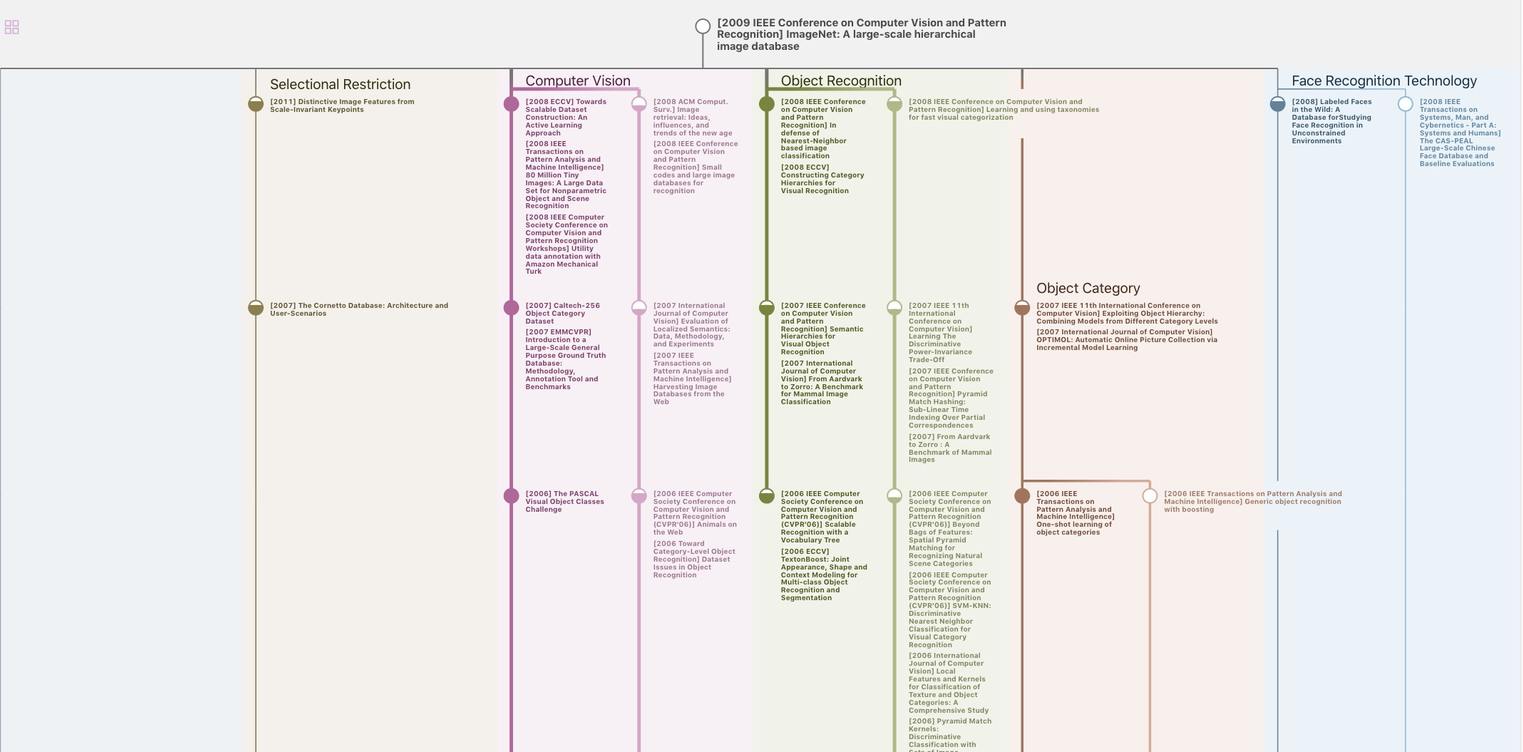
生成溯源树,研究论文发展脉络
Chat Paper
正在生成论文摘要