Gbras-Net: A Convolutional Neural Network Architecture For Spatial Image Steganalysis
IEEE ACCESS(2021)
摘要
Advances in Deep Learning (DL) have provided alternative approaches to various complex problems, including the domain of spatial image steganalysis using Convolutional Neural Networks (CNN). Several CNN architectures have been developed in recent years, which have improved the detection accuracy of steganographic images. This work presents a novel CNN architecture which involves a preprocessing stage using filter banks to enhance steganographic noise, a feature extraction stage using depthwise and separable convolutional layers, and skip connections. Performance was evaluated using the BOSSbase 1.01 and BOWS 2 datasets with different experimental setups, including adaptive steganographic algorithms, namely WOW, S-UNIWARD, MiPOD, HILL and HUGO. Our results outperformed works published in the last few years in every experimental setting. This work improves classification accuracies on all algorithms and bits per pixel (bpp), reaching 80.3% on WOW with 0.2 bpp and 89.8% on WOW with 0.4 bpp, 73.6% and 87.1% on S-UNIWARD (0.2 and 0.4 bpp respectively), 68.3% and 81.4% on MiPOD (0.2 and 0.4 bpp), 68.5% and 81.9% on HILL (0.2 and 0.4 bpp), 74.6% and 84.5% on HUGO (0.2 and 0.4 bpp), using BOSSbase 1.01 test data.
更多查看译文
关键词
Convolution, Computer architecture, Feature extraction, Convolutional neural networks, Task analysis, Payloads, Licenses, Convolutional neural network, deep learning, GBRAS-Net, steganalysis, steganography
AI 理解论文
溯源树
样例
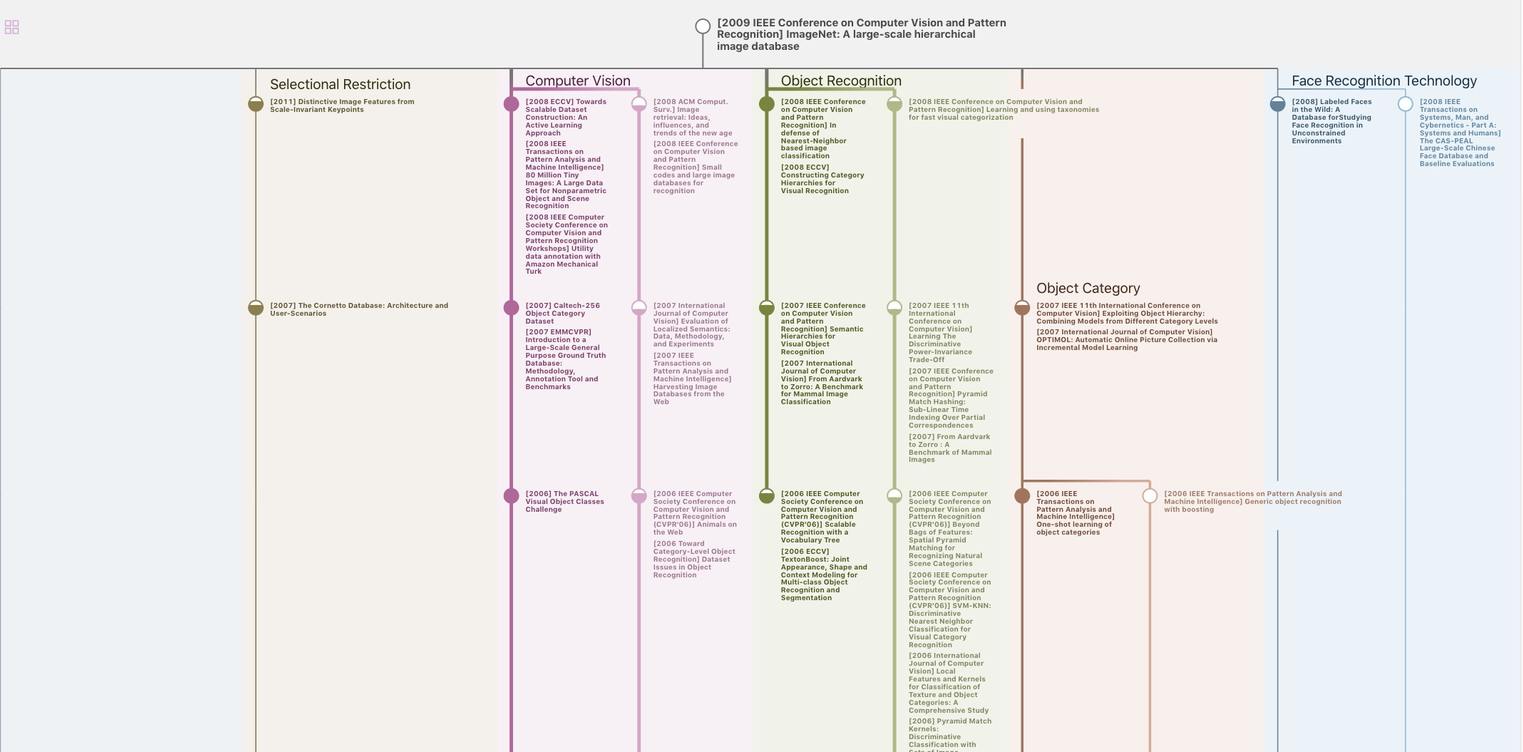
生成溯源树,研究论文发展脉络
Chat Paper
正在生成论文摘要