Lossless Compression of Bilevel ROI Maps of Hyperspectral Images Using Optimization Algorithms
IEEE GEOSCIENCE AND REMOTE SENSING LETTERS(2022)
摘要
In this letter, we propose a novel scheme for the compression of the bilevel region of interest (ROI) maps of hyperspectral images. The scheme can achieve high compression ratios by novel ways of partitioning the intricate regions of the image into smaller blocks. We first analyzed the effect of a low-order linear predictive model on the original image. The analysis showed that linear prediction results in lower-entropy residual images compared to the original images, which in turn helps in improving the compression efficiency. We then use the optimization algorithm that finds the best combination of scan directions for the nonzero blocks. It is shown to offer increasingly better compression with additional iterations. The simulation results on various data sets show that our scheme outperforms the international standard for binary image compression [joint bi-level image expert (JBIG2)].
更多查看译文
关键词
Image coding, Hyperspectral imaging, Optimization, Redundancy, Prediction algorithms, Entropy, Search methods, Bilevel images, discrete particle swarm optimization (DPSO), lossless compression, region of interest (ROI) maps
AI 理解论文
溯源树
样例
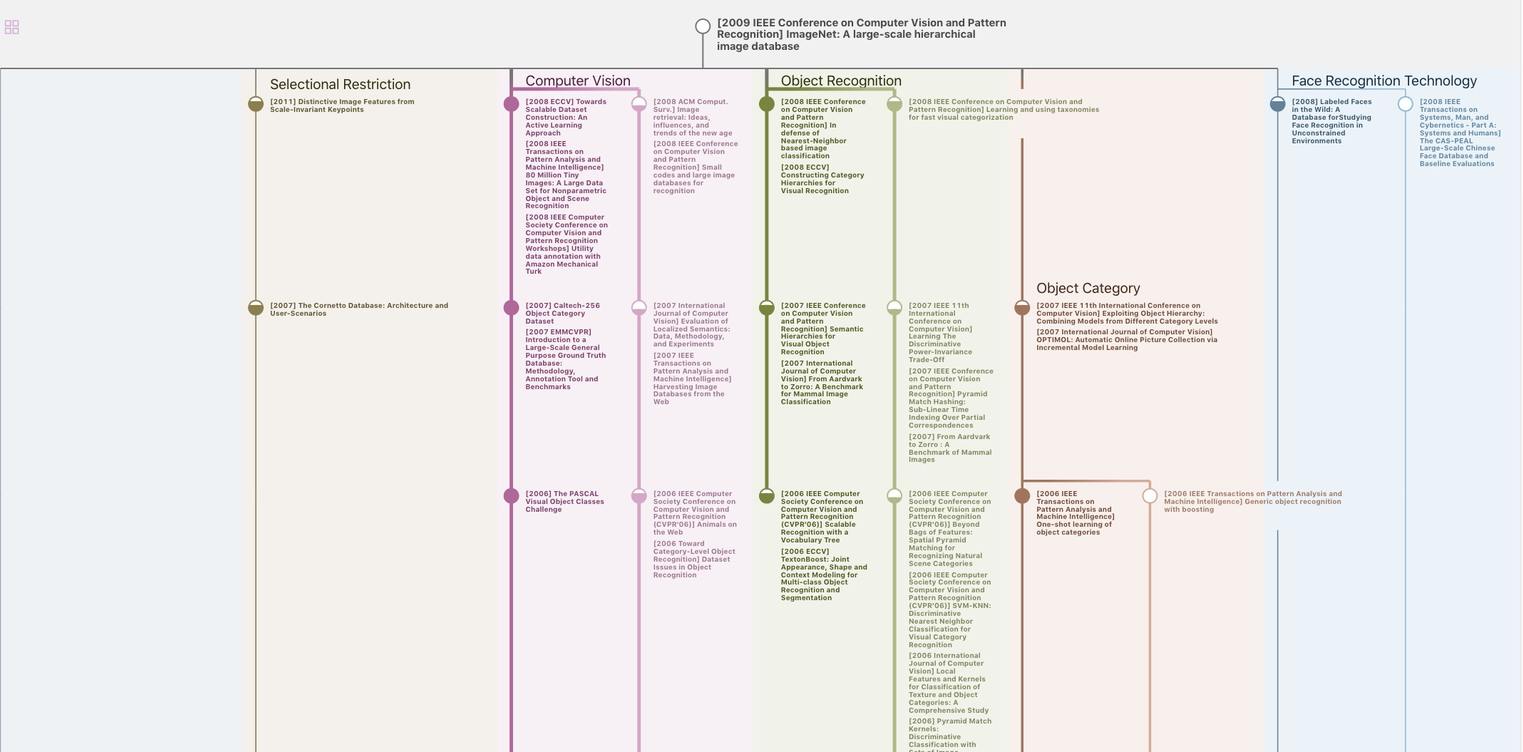
生成溯源树,研究论文发展脉络
Chat Paper
正在生成论文摘要