Efficient Attention Pyramid Network For Semantic Segmentation
IEEE ACCESS(2021)
摘要
Semantic segmentation is a task that covers most of the perception needs of intelligent vehicles in an unified way. Recent studies witnessed that attention mechanisms achieve impressive performance in computer vision task. Current attention mechanisms based segmentation methods differ with each other in position and form of the attention mechanism, and perform differently in practice. This paper firstly introduces the effectiveness of multi-scale context features and attention mechanisms in segmentation tasks. We find that multi-scale and channel attention can play a vital role in constructing effective context features. Based on this analysis, this paper proposes an efficient attention pyramid network (EAPNet) for semantic segmentation. Specifically, to efficient handle the problem of segmenting objects at multiple scales, we design efficient channel attention pyramid (ECAP) which employ atrous convolution with channel attention in cascade or in parallel to capture multi-scale context by using multiple atrous rates. Furthermore, we propose a residual attention fusion block (RAFB), whose purpose is to simultaneously focus on meaningful low-level feature maps and spatial location information. At the same time, we will explore different channel attention modules and spatial attention modules, and describe their impact on network performance. We empirically evaluate our EAPNet on two semantic segmentation datasets, including PASCAL VOC 2012 and Cityscapes datasets. Experimental results show that without MS COCO pre-training and any post-processing, EAPNet achieved 81.7% mIoU on the PASCAL VOC 2012 validation set. With deeplabv3+ as the benchmark, EAPNet improve the model performance of more than 1.50% mIoU.
更多查看译文
关键词
Semantics, Convolution, Feature extraction, Task analysis, Image segmentation, Decoding, Computer vision, Semantic segmentation, attention mechanism, spatial pyramid, PASCAL VOC 2012, Cityscapes
AI 理解论文
溯源树
样例
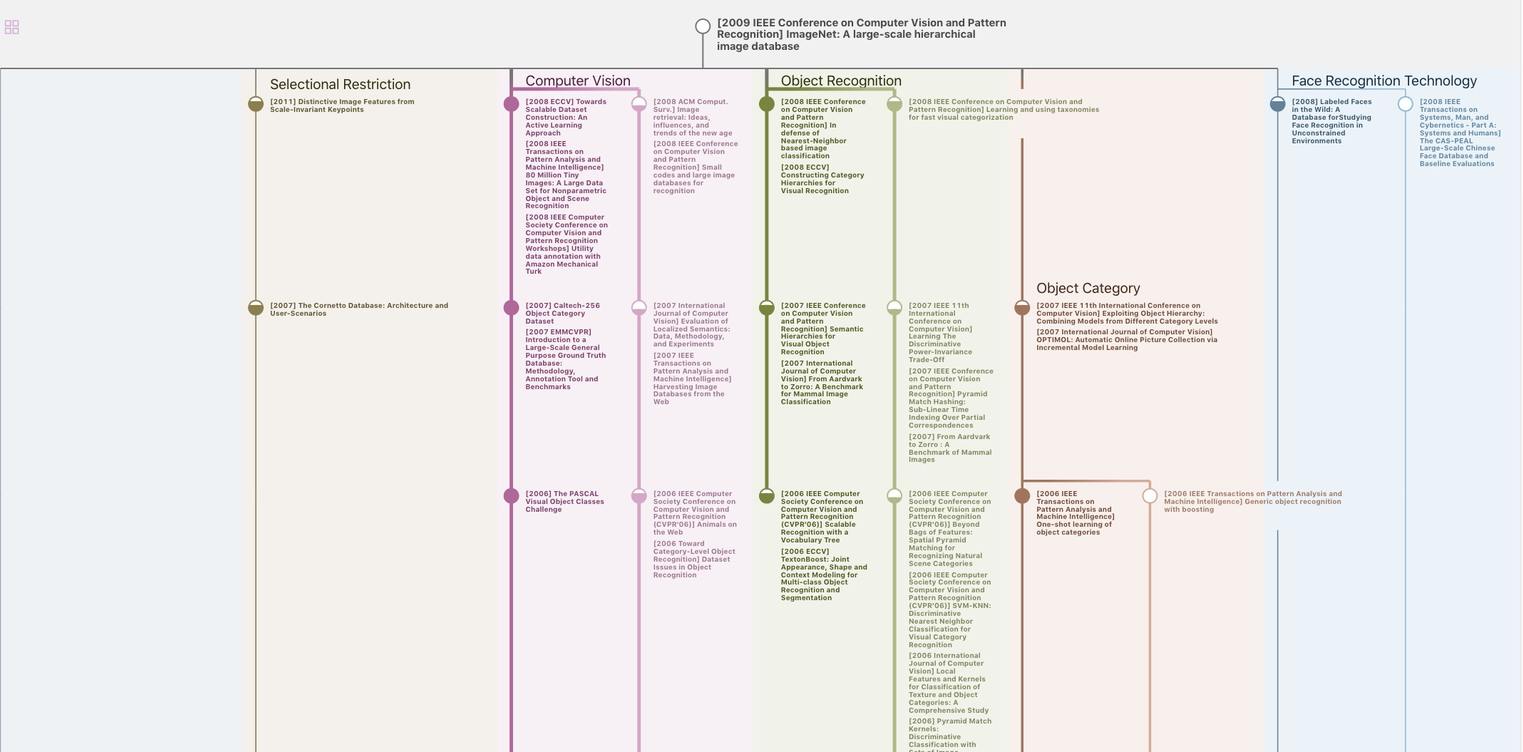
生成溯源树,研究论文发展脉络
Chat Paper
正在生成论文摘要