Image-Based Prediction Of Granular Flow Behaviors In A Wedge-Shaped Hopper By Combing Dem And Deep Learning Methods
POWDER TECHNOLOGY(2021)
摘要
Granular flow has solid-, liquid-, or even gas-like behaviors, which can be described through discrete element method (DEM)-based simulations. Although the DEM simulation has advantages in studying particle-scale information, it is computationally intensive. Alternatively, this work proposes to combine the DEM and deep learning methods to predict granular flow behaviors in a wedge-shaped hopper. As the image-based labels are extracted from the DEM simulation, an Alexnet-fully connection (FC) model can make point-to-point predictions about the discharge time. Furthermore, when the first 20% of image-based datasets in the timing sequence are used to train a convolutional neural network (CNN)-long short-term memory (LSTM) network, it can make process predictions about the number ratio of remaining particles (NRRP) in the hopper vs. the discharge time. Although these attempts have some shortcomings at the present stage, more efforts are encouraged to stimulate the future potential of image-based prediction through the combined methods.(c) 2021 Elsevier B.V. All rights reserved.
更多查看译文
关键词
Image-based prediction, DEM, Deep learning, Combined methods, Granular flow, Wedge-shaped hopper
AI 理解论文
溯源树
样例
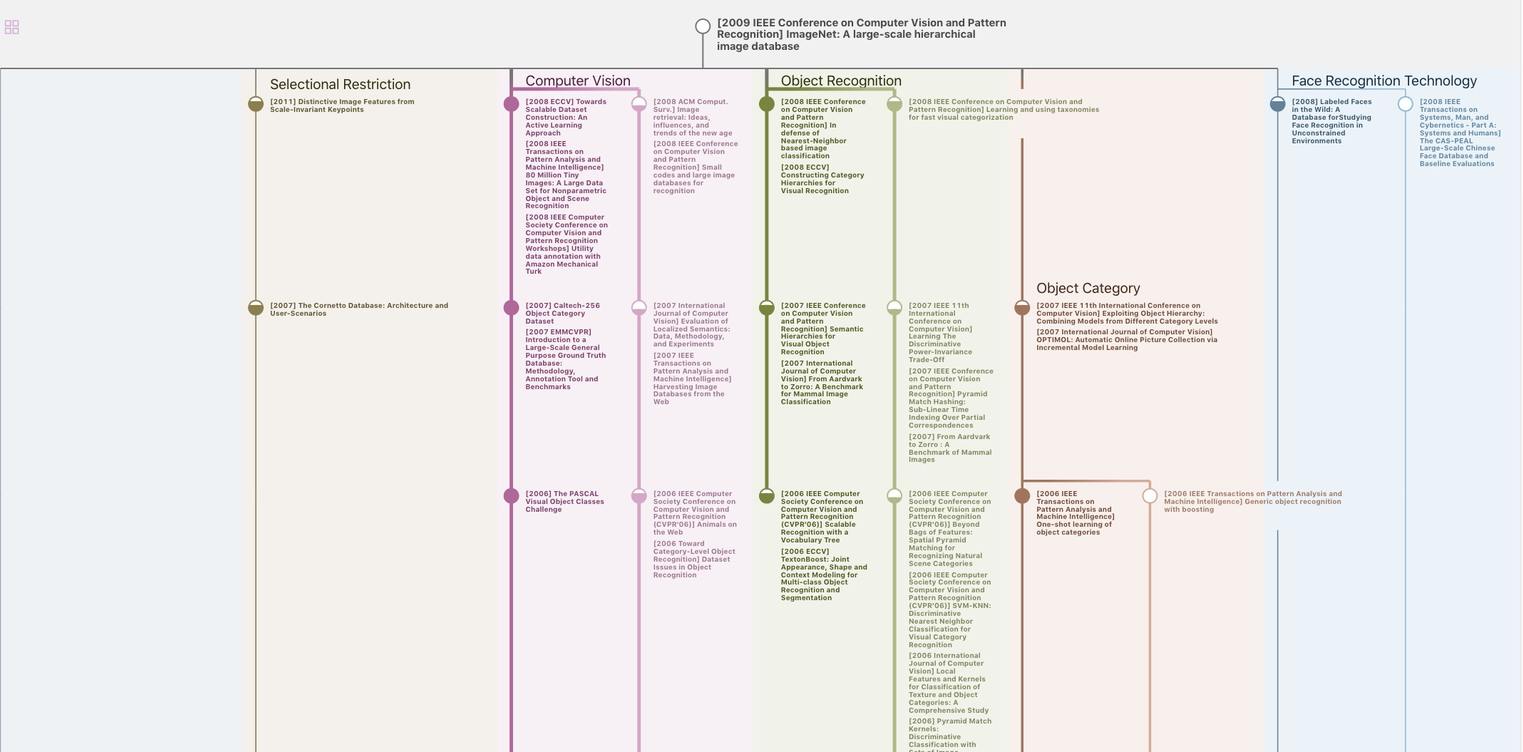
生成溯源树,研究论文发展脉络
Chat Paper
正在生成论文摘要