Synthetic-Aperture Radar Image Based Positioning In Gps-Denied Environments Using Deep Cosine Similarity Neural Networks
INVERSE PROBLEMS AND IMAGING(2021)
摘要
Navigating unmanned aerial vehicles in precarious environments is of great importance. It is necessary to rely on alternative information processing techniques to attain spatial information that is required for navigation in such settings. This paper introduces a novel deep learning-based approach for navigating that exclusively relies on synthetic aperture radar (SAR) images. The proposed method utilizes deep neural networks (DNNs) for image matching, retrieval, and registration. To this end, we introduce Deep Cosine Similarity Neural Networks (DCSNNs) for mapping SAR images to a global descriptive feature vector. We also introduce a fine-tuning algorithm for DC-SNNs, and DCSNNs are used to generate a database of feature vectors for SAR images that span a geographic area of interest, which, in turn, are compared against a feature vector of an inquiry image. Images similar to the inquiry are retrieved from the database by using a scalable distance measure between the feature vector outputs of DCSNN. Methods for reranking the retrieved SAR images that are used to update position coordinates of an inquiry SAR image by estimating from the best retrieved SAR image are also introduced. Numerical experiments comparing with baselines on the Polarimetric SAR (PolSAR) images are presented.
更多查看译文
关键词
Synthetic Aperture Radar (SAR), polarimetric SAR (PolSAR), Deep Cosine Similarity Neural Networks, image graphs, image retrieval, image registration, scale-invariant feature transform (SIFT), SAR-SIFT, remote sensing
AI 理解论文
溯源树
样例
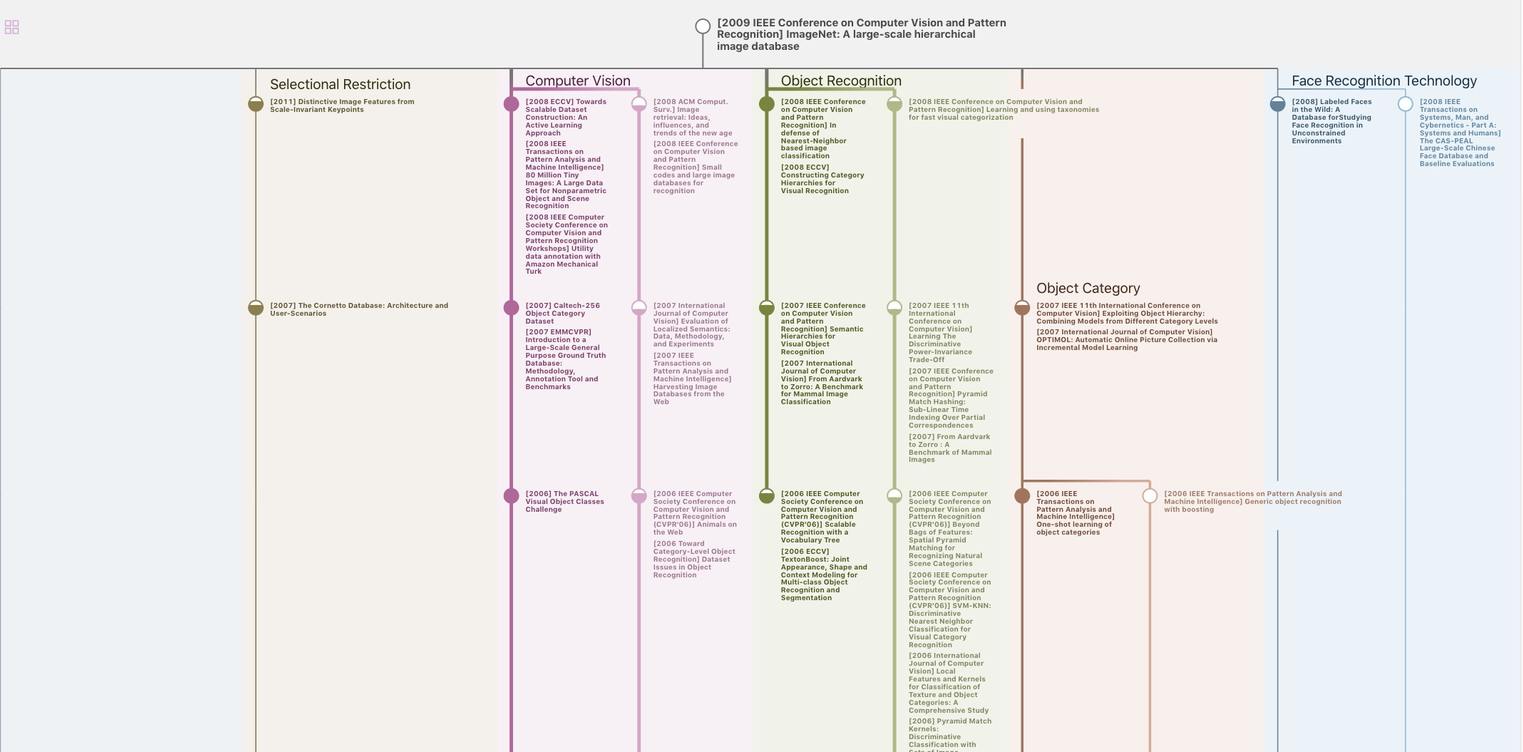
生成溯源树,研究论文发展脉络
Chat Paper
正在生成论文摘要