Graph Regularized Bayesian Tensor Factorization Based On Kronecker-Decomposable Dictionary
COMPUTERS & ELECTRICAL ENGINEERING(2021)
摘要
The tensor factorization techniques preserve the intrinsic structure information better than the matrix factorization technique. However, the outliers on each mode are usually non-independent and identically distributed (non-i.i.d) and the incoherence is non-uniform. The existing matrix factorization methods cannot deal with the non-i.i.d outliers and the non-uniform incoherence well. Although Kronecker-Decomposable sparse dictionary learning (KDSDL) model has shown effectiveness in dealing with the non-uniform incoherence, the non-i.i.d outliers and the correlation between different mode are still not considered. To address these problems, we propose a graph regularized Bayesian tensor factorization based on KDSDL model. First, to consider the non-i.i.d noise in real scenario, we embed the mixture of outliers in each mode through KDSDL model; Second, to capture the correlation between different modes, a novel graph sparsity-inducing regularization is proposed. The experiments on tensor data with complex outliers demonstrate the superiority of the proposed methods over the existing state-of-the-art methods.
更多查看译文
关键词
Bayesian tensor factorization, Kronecker-Decomposition, Dictionary learning, Graph sparsity
AI 理解论文
溯源树
样例
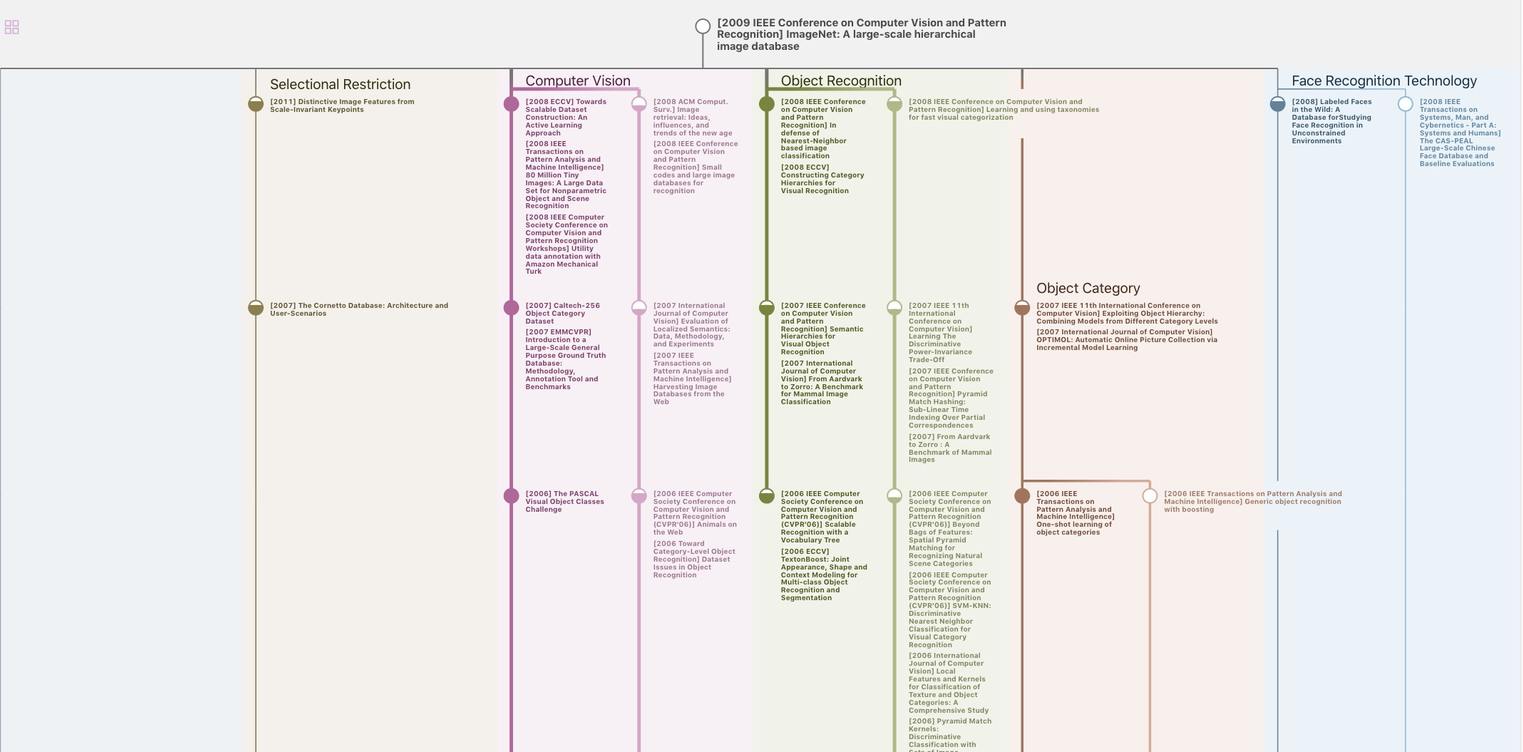
生成溯源树,研究论文发展脉络
Chat Paper
正在生成论文摘要