Deep-FDA: Using Functional Data Analysis and Neural Networks to Characterize Network Services Time Series
IEEE Transactions on Network and Service Management(2021)
摘要
In network management, it is important to model baselines, trends, and regular behaviors to adequately deliver network services. However, their characterization is complex, so network operation and system alarming become a challenge. Several problems exist: Gaussian assumptions cannot be made, time series have different trends, and it is difficult to reduce their dimensionality. To overcome this situation, we propose Deep-FDA, a novel approach for network service modeling that combines functional data analysis (FDA) and neural networks. Specifically, we explore the use of functional clustering and functional depth measurements to characterize network services with time series generated from enriched flow records, showing how this method can detect different separated trends. Moreover, we augment this statistical approach with the use of autoencoder neural networks, improving the classification results. To evaluate and check the applicability of our proposal, we performed experiments with synthetic and real-world data, where we show graphically and numerically the performance of our method compared to other state-of-the-art alternatives. We also exemplify its application in different network management use cases. The results show that FDA and neural networks are complementary, as they can help each other to improve the drawbacks that both analysis methods have when are applied separately.
更多查看译文
关键词
Functional data analysis,network monitoring and management,autoencoders,service characterization,time series,baseline model%
AI 理解论文
溯源树
样例
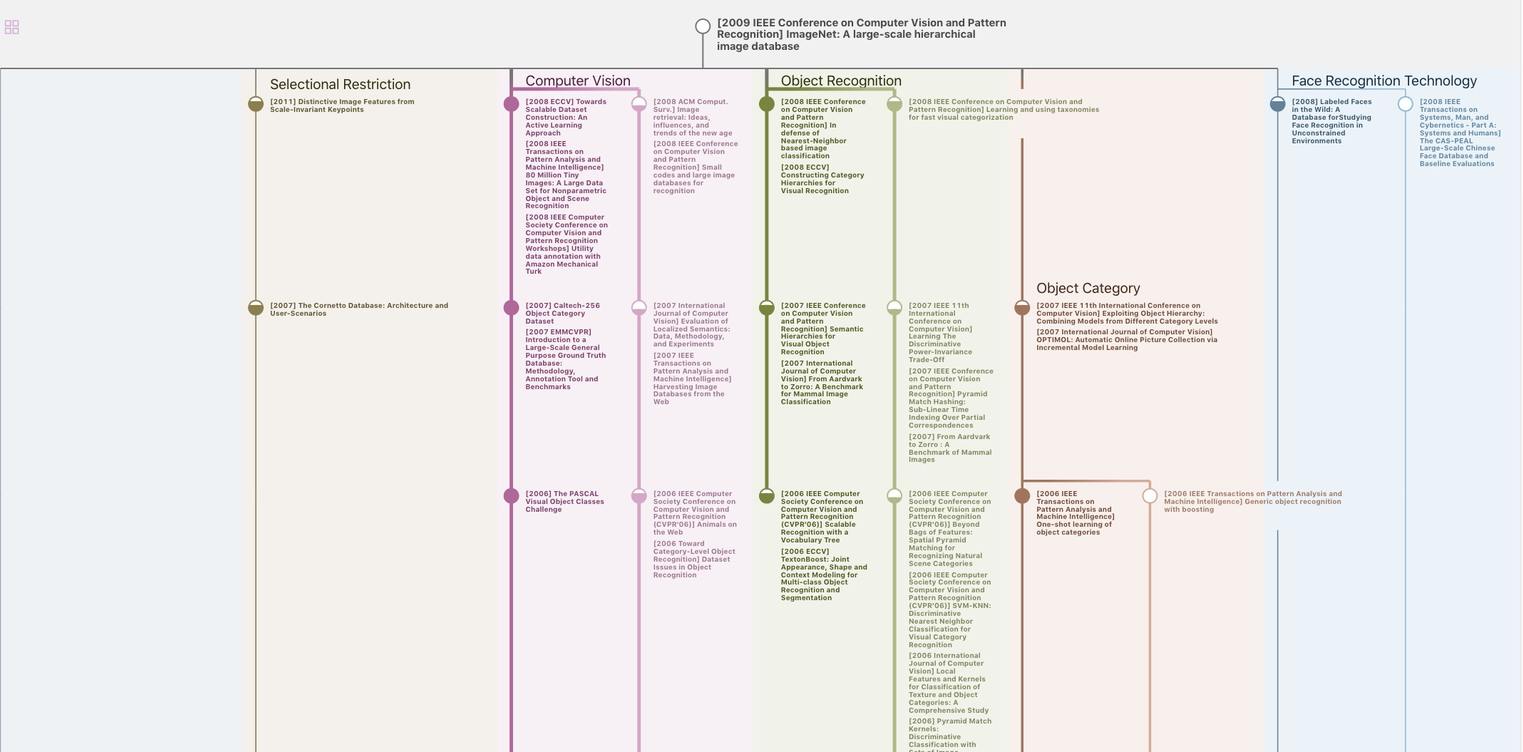
生成溯源树,研究论文发展脉络
Chat Paper
正在生成论文摘要