Particle Filter Refinement Based On Clustering Procedures For High-Dimensional Localization And Mapping Systems
ROBOTICS AND AUTONOMOUS SYSTEMS(2021)
摘要
Developing safe autonomous robotic applications for outdoor agricultural environments is a research field that still presents many challenges. Simultaneous Localization and Mapping can be crucial to endow the robot to localize itself with accuracy and, consequently, perform tasks such as crop monitoring and harvesting autonomously. In these environments, the robotic localization and mapping systems usually benefit from the high density of visual features. When using filter-based solutions to localize the robot, such an environment usually uses a high number of particles to perform accurately. These two facts can lead to computationally expensive localization algorithms that are intended to perform in real-time. This work proposes a refinement step to a standard high-dimensional filter based localization solution through the novelty of downsampling the filter using an online clustering algorithm and applying a scan-match procedure to each cluster. Thus, this approach allows scan matchers without high computational cost, even in high dimensional filters. Experiments using real data in an agricultural environment show that this approach improves the Particle Filter performance estimating the robot pose. Additionally, results show that this approach can build a precise 3D reconstruction of agricultural environments using visual scans, i.e., 3D scans with RGB information. (c) 2021 Elsevier B.V. All rights reserved.
更多查看译文
关键词
SLAM, Clustering, Agricultural robotics
AI 理解论文
溯源树
样例
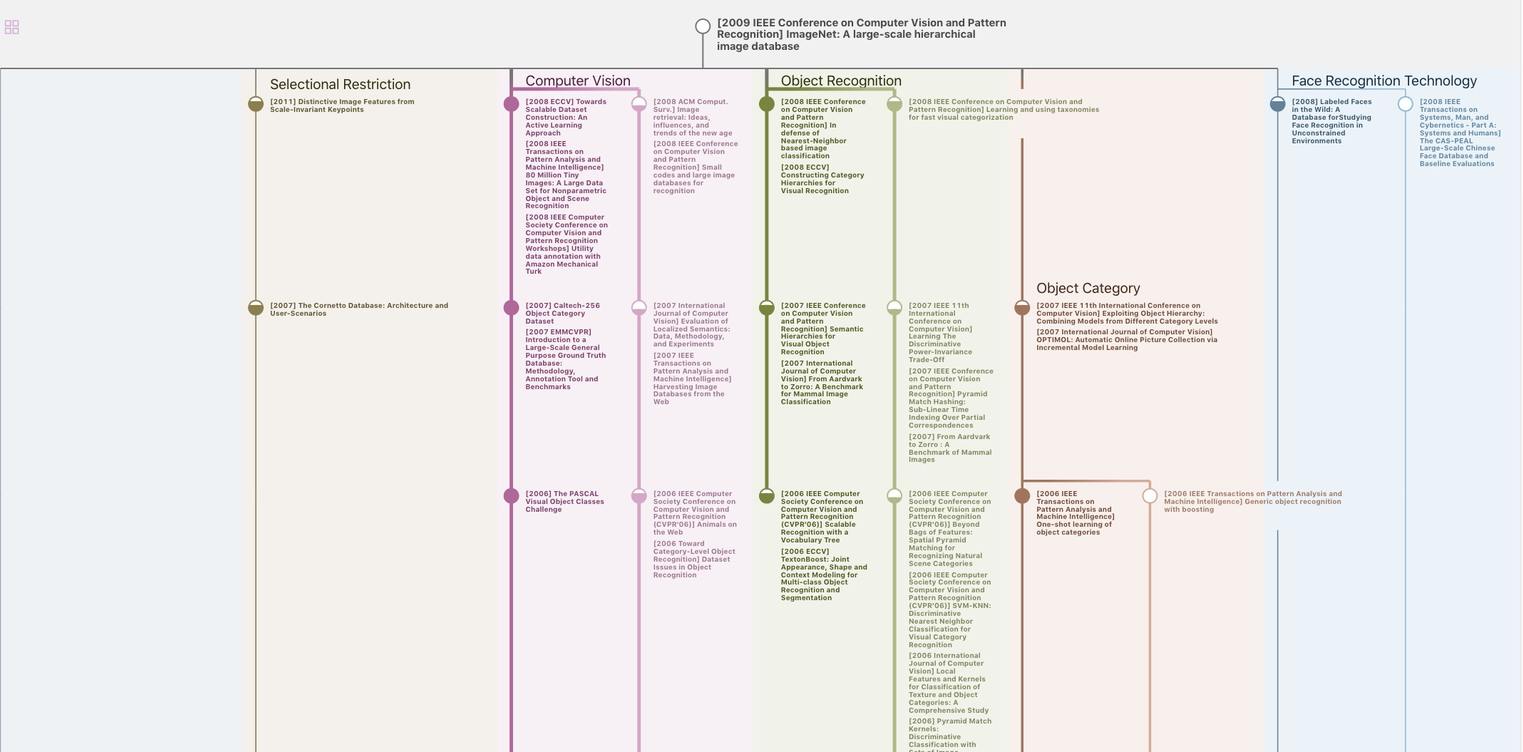
生成溯源树,研究论文发展脉络
Chat Paper
正在生成论文摘要