Simultaneous And Continuous Estimation Of Joint Angles Based On Surface Electromyography State-Space Model
IEEE SENSORS JOURNAL(2021)
摘要
Simultaneous and continuous joint angle estimation plays an important role in motion intention recognition and rehabilitation training. A surface electromyography (sEMG) state-space model is proposed to estimate simultaneous and continuous lower-limb-joint movements from sEMG signals in this article. The model combines the forward dynamics with Hill-based muscle model (HMM), making the extended model capable of estimating the lower-limb-joint motion directly. sEMG features including root-mean-square and wavelet coefficients are then extracted to construct a measurement equation used to reduce system error and external disturbances. With the proposed model, unscented Kalman filter is used to estimate joint angle from sEMG signals. In the experiments, sEMG signals were recorded from ten subjects during muscle contraction involving three lower-limb-joint motions (knee-joint motion, ankle-joint motion, and simultaneous knee-ankle-joint motion). Comprehensive experiments are conducted on three motions and the results show that the mean rootmean square error for knee-joint motion, ankle-joint motion, and simultaneous motion of the proposed model are 5.1143 5.2647, and 6.3941, respectively, and significant improvements are demonstrated compared with the traditional methods.
更多查看译文
关键词
Hidden Markov models, Muscles, Estimation, Mathematical model, Sensors, Force, Electromyography, Surface electromyography (sEMG), muscle model, feature extraction, continuous joint motion
AI 理解论文
溯源树
样例
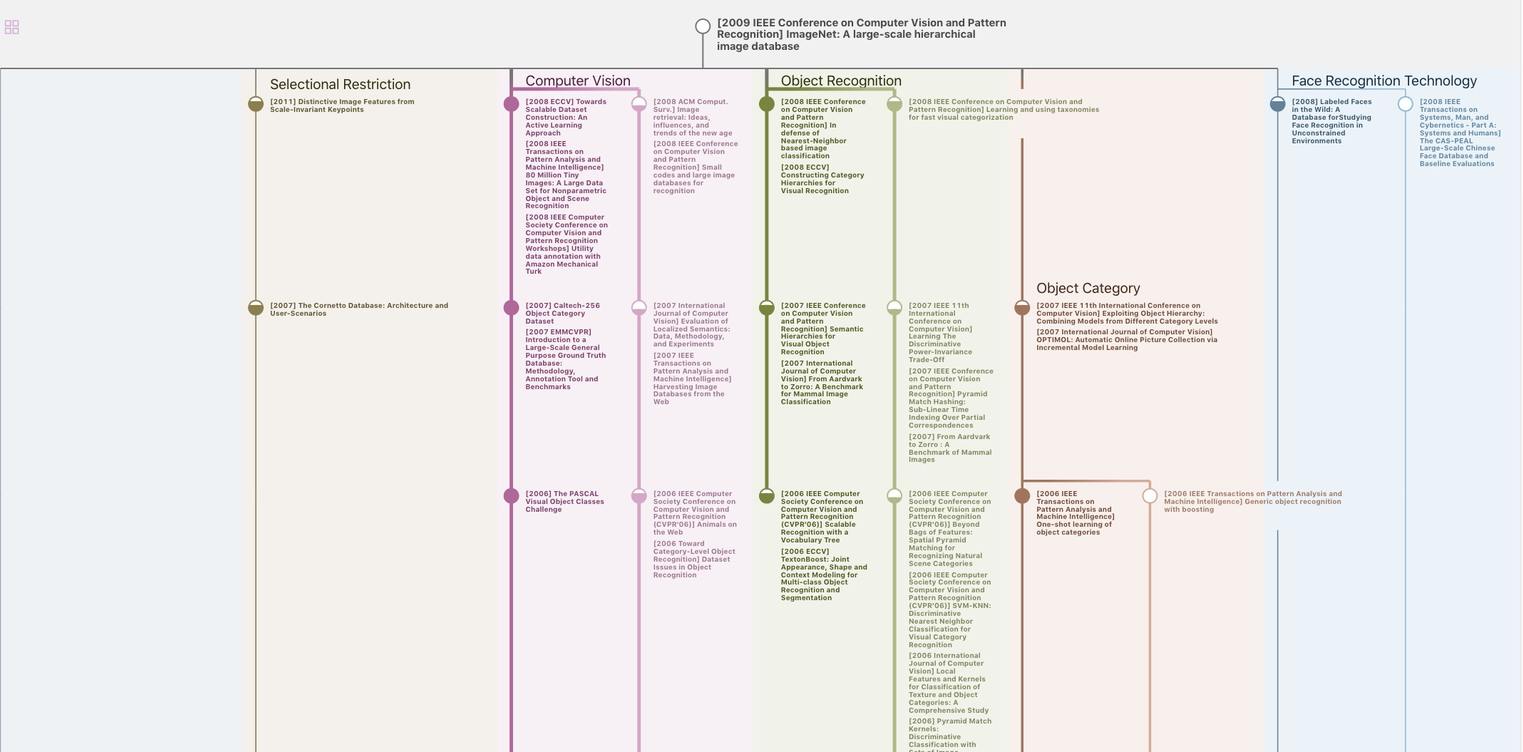
生成溯源树,研究论文发展脉络
Chat Paper
正在生成论文摘要