Robust And Efficient Classification For Underground Metal Target Using Dimensionality Reduction And Machine Learning
IEEE ACCESS(2021)
摘要
Underground metal target detection technology has been widely applied in industrial production, resource exploration, and engineering construction, etc. However, due to the influence of non-negligible noise and high dimensionality in collected data, achieving efficient and accurate underground target classification remains a grand challenge for further applications of underground metal target detection on portable devices with limited computing capability and energy supply. This study aimed to seek out robust and efficient data-based strategies to classify the underground metal targets of different shapes and materials based on electromagnetic induction detection. We investigated thirty-three classification strategies based on eleven dimensionality reduction methods, namely, the least absolute shrinkage and selection operator (LASSO), genetic algorithm-support vector machine (GA-SVM), Pearson correlation coefficient (PCC), mutual information (MI), maximal relevance minimal redundancy Pearson correlation (mRMRP), maximal relevance minimal redundancy mutual information (mRMRMI), statistical features (SF), principal component analysis (PCA), kernel principal component analysis (KPCA), locally linear embedding (LLE), and stacked denoising autoencoder (SDAE), and three machine learning models, namely, artificial neural network (ANN), linear support vector machine (L-SVM), and Gaussian Naive Bayes (GNB). Several parameters, including classification accuracy, the number of features after dimensionality, the feature type importance, and the time consumption were considered to evaluate the data-based classification strategies. Among the classification strategies investigated and considering the above evaluation parameters, the artificial neural network (ANN) classifier assisted with the kernel principal component analysis (KPCA) feature extraction method yielded the best performance in the material-based classification (accuracy:0.99) and the shape-based classification (accuracy:0.99). The locally linear embedding (LLE) improved the robustness of machine learning classifiers and efficiency of the artificial neural network in the material-based classification (improvement of average accuracy:0.17, reduction of classification time cost:14%) and shape-based classification (improvement of average accuracy:0.16, reduction of classification time cost:22%). Our comparative investigation provides a robust and efficient data-based strategy for underground metal target classification, which is significant for applications of underground metal target detection on portable devices with limited computing capability and energy supply. The cross-combination strategy of dimensionality reduction methods and machine learning models provides a way to find the optimal machine learning model for underground target detection.
更多查看译文
关键词
Dimensionality reduction, machine learning, underground target classification, underground target detection
AI 理解论文
溯源树
样例
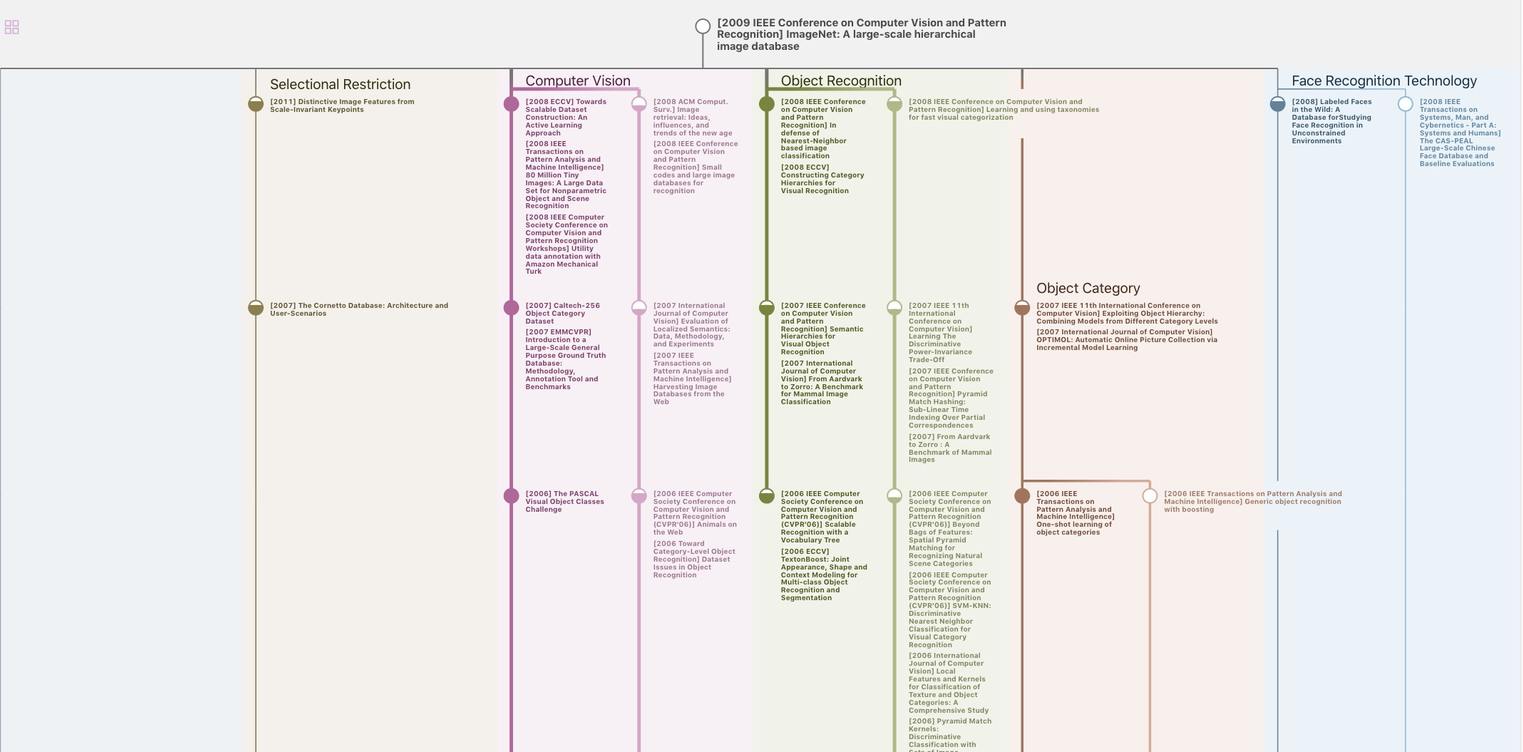
生成溯源树,研究论文发展脉络
Chat Paper
正在生成论文摘要