Robust Spline Adaptive Filtering Based On Accelerated Gradient Learning: Design And Performance Analysis
SIGNAL PROCESSING(2021)
摘要
This paper proposes a novel spline adaptive filtering (SAF) algorithm for nonlinear system identification under impulsive noise environments. This algorithm combines the logarithmic hyperbolic cosine (LHC) cost function and the modified Nesterov accelerated gradient (MNAG) learning method, which is called the SAF-LHC-MNAG algorithm. The LHC cost function can reduce the sensitivity of SAF to large outliers and improve the robustness to impulsive noises. Additionally, the MNAG method can further accelerate the convergence under the premise of low steady-state error. Performance analysis of this algorithm is carried out and supported by simulations. Numerical results show that the SAF-LHC-MNAG algorithm has better convergence performance than some existing SAF algorithms. Besides, experimental results confirm the effectiveness of SAF-LHC-MNAG for the accurate identification of nonlinear hysteresis system. (C) 2021 Elsevier B.V. All rights reserved.
更多查看译文
关键词
Impulsive noise, Nesterov accelerated gradient, Nonlinear system identification, Spline adaptive filtering
AI 理解论文
溯源树
样例
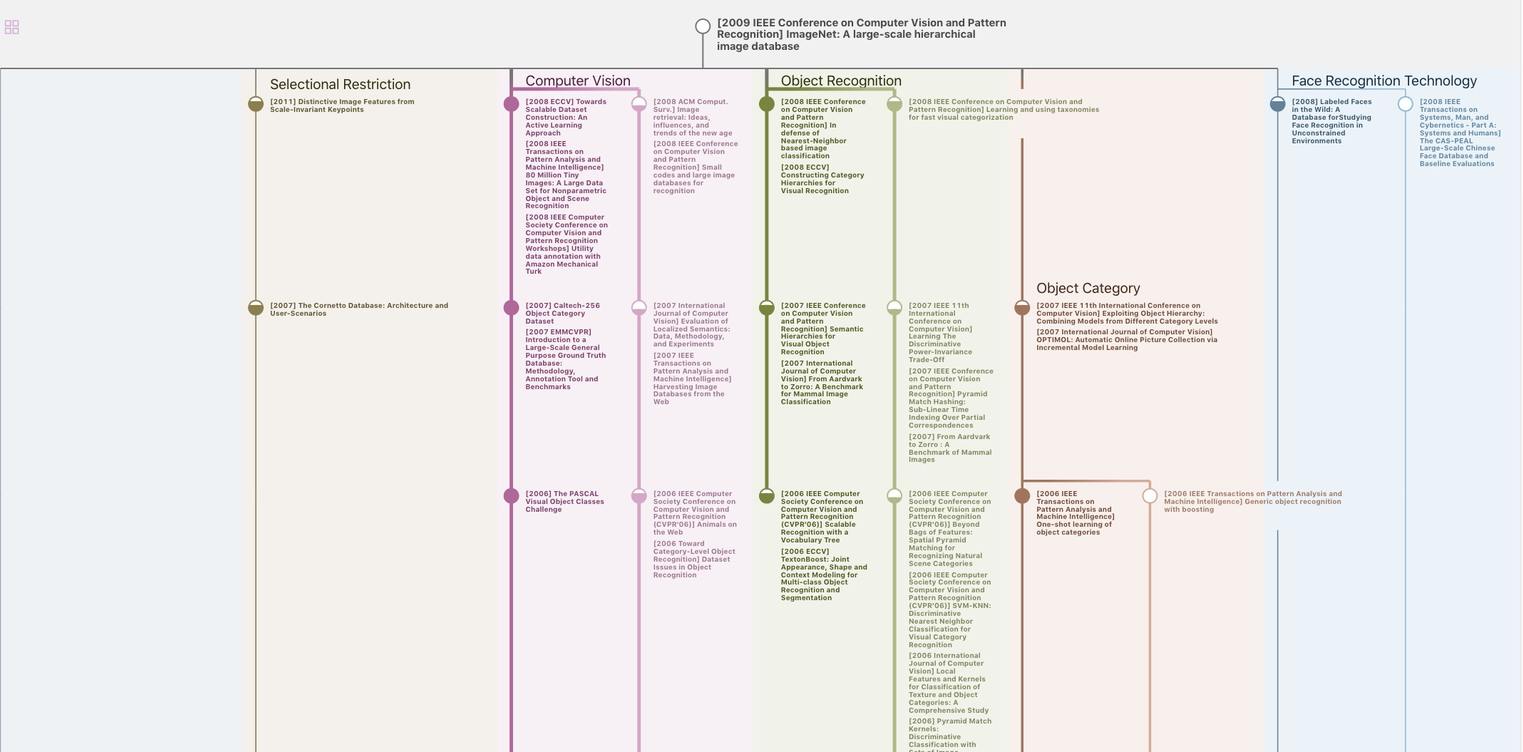
生成溯源树,研究论文发展脉络
Chat Paper
正在生成论文摘要