Equation Discovery For Nonlinear Dynamical Systems: A Bayesian Viewpoint
MECHANICAL SYSTEMS AND SIGNAL PROCESSING(2021)
摘要
This paper presents a new Bayesian approach to equation discovery - combined structure detection and parameter estimation - for system identification (SI) in nonlinear structural dynamics. The structure detection is accomplished via a sparsity-inducing prior within a Relevance Vector Machine (RVM) framework; the prior ensures that terms making no contribution to the model are driven to zero coefficient values. Motivated by the idea of compressive sensing (CS) and recent results from the machine learning community on sparse linear regression, the paper adopts the use of an over-complete dictionary to represent a large number of candidate terms for the equation describing the system. Unlike other sparse learners, like the Lasso and its derivatives, which are potentially sensitive to hyperparameter selection, the proposed method exploits the principled means of fixing priors and hyperpriors that are available via a hierarchical Bayesian approach. The approach is successfully demonstrated and validated via a number of simulated case studies of common Single-Degree-of-Freedom (SDOF) nonlinear dynamic systems, and on two challenging experimental data sets. (C) 2020 Elsevier Ltd. All rights reserved.
更多查看译文
关键词
Equation discovery, Nonlinear system identification, Sparse Bayesian learning, Relevance Vector Machine (RVM)
AI 理解论文
溯源树
样例
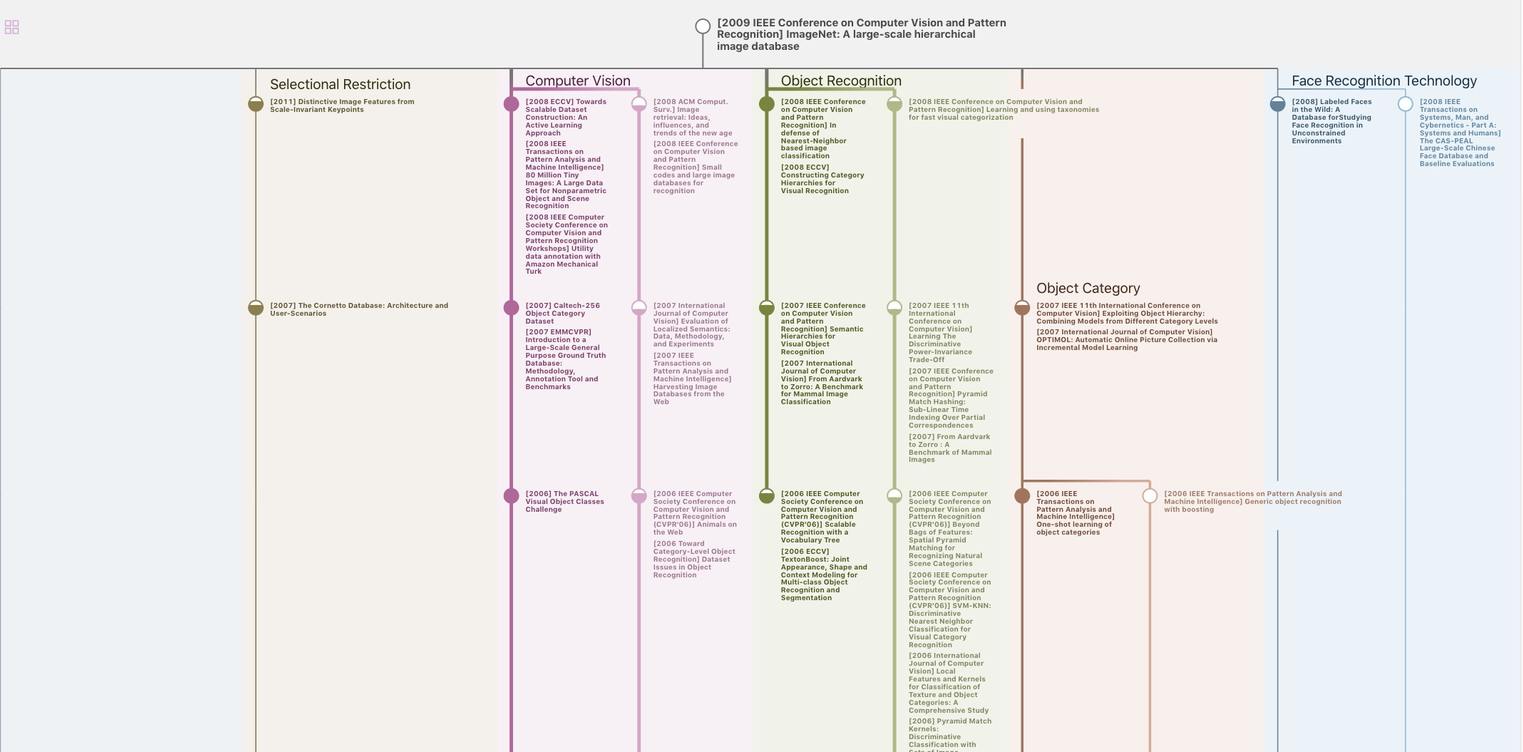
生成溯源树,研究论文发展脉络
Chat Paper
正在生成论文摘要