Machine Learning for Statistical Modeling: The Case of Perpendicular Spin-Transfer-Torque Random Access Memory
ACM Transactions on Design Automation of Electronic Systems(2021)
摘要
AbstractWe propose a methodology to perform process variation-aware device and circuit design using fully physics-based simulations within limited computational resources, without developing a compact model. Machine learning (ML), specifically a support vector regression (SVR) model, has been used. The SVR model has been trained using a dataset of devices simulated a priori, and the accuracy of prediction by the trained SVR model has been demonstrated. To produce a switching time distribution from the trained ML model, we only had to generate the dataset to train and validate the model, which needed ∼500 hours of computation. On the other hand, if 106 samples were to be simulated using the same computation resources to generate a switching time distribution from micromagnetic simulations, it would have taken ∼250 days. Spin-transfer-torque random access memory (STTRAM) has been used to demonstrate the method. However, different physical systems may be considered, different ML models can be used for different physical systems and/or different device parameter sets, and similar ends could be achieved by training the ML model using measured device data.
更多查看译文
关键词
Spin-transfer-torque random access memory, process variation, machine learning, support vector regression
AI 理解论文
溯源树
样例
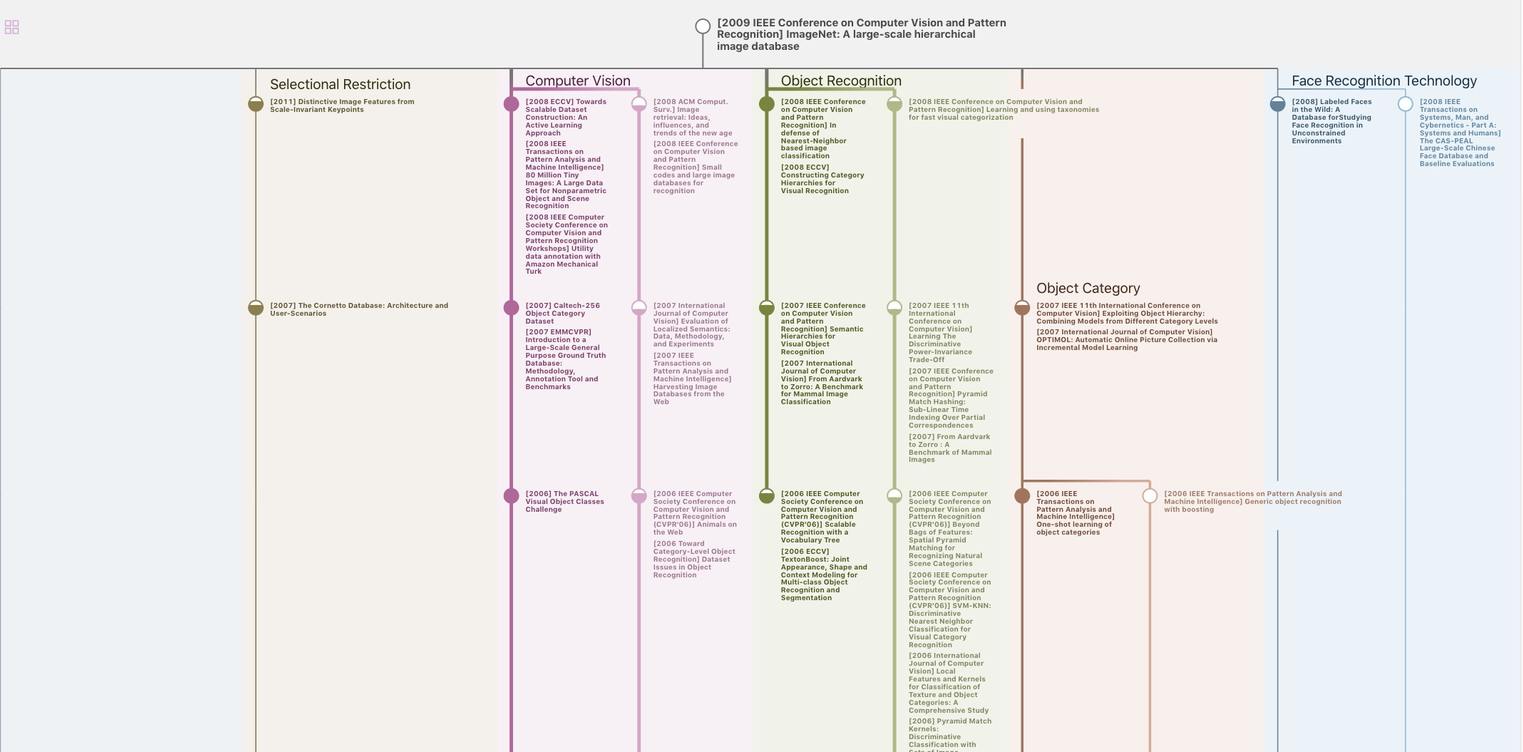
生成溯源树,研究论文发展脉络
Chat Paper
正在生成论文摘要