A Weighted Spatially Constrained Finite Mixture Model For Image Segmentation
CMC-COMPUTERS MATERIALS & CONTINUA(2021)
摘要
Spatially Constrained Mixture Model (SCMM) is an image segmentation model that works over the framework of maximum a-posteriori and Markov Random Field (MAP-MRF). It developed its own maximization step to be used within this framework. This research has proposed an improvement in the SCMM's maximization step for segmenting simulated brain Magnetic Resonance Images (MRIs). The improved model is named as the Weighted Spatially Constrained Finite Mixture Model (WSCFMM). To compare the performance of SCMM and WSCFMM, simulated T1-Weighted normal MRIs were segmented. A region of interest (ROI) was extracted from segmented images. The similarity level between the extracted ROI and the ground truth (GT) was found by using the Jaccard and Dice similarity measuring method. According to the Jaccard similarity measuring method, WSCFMM showed an overall improvement of 4.72%, whereas the Dice similarity measuring method provided an overall improvement of 2.65% against the SCMM. Besides, WSCFMM significantly stabilized and reduced the execution time by showing an improvement of 83.71%. The study concludes that WSCFMM is a stable model and performs better as compared to the SCMM in noisy and noise-free environments.
更多查看译文
关键词
Finite mixture model, maximum aposteriori, Markov random field, image segmentation
AI 理解论文
溯源树
样例
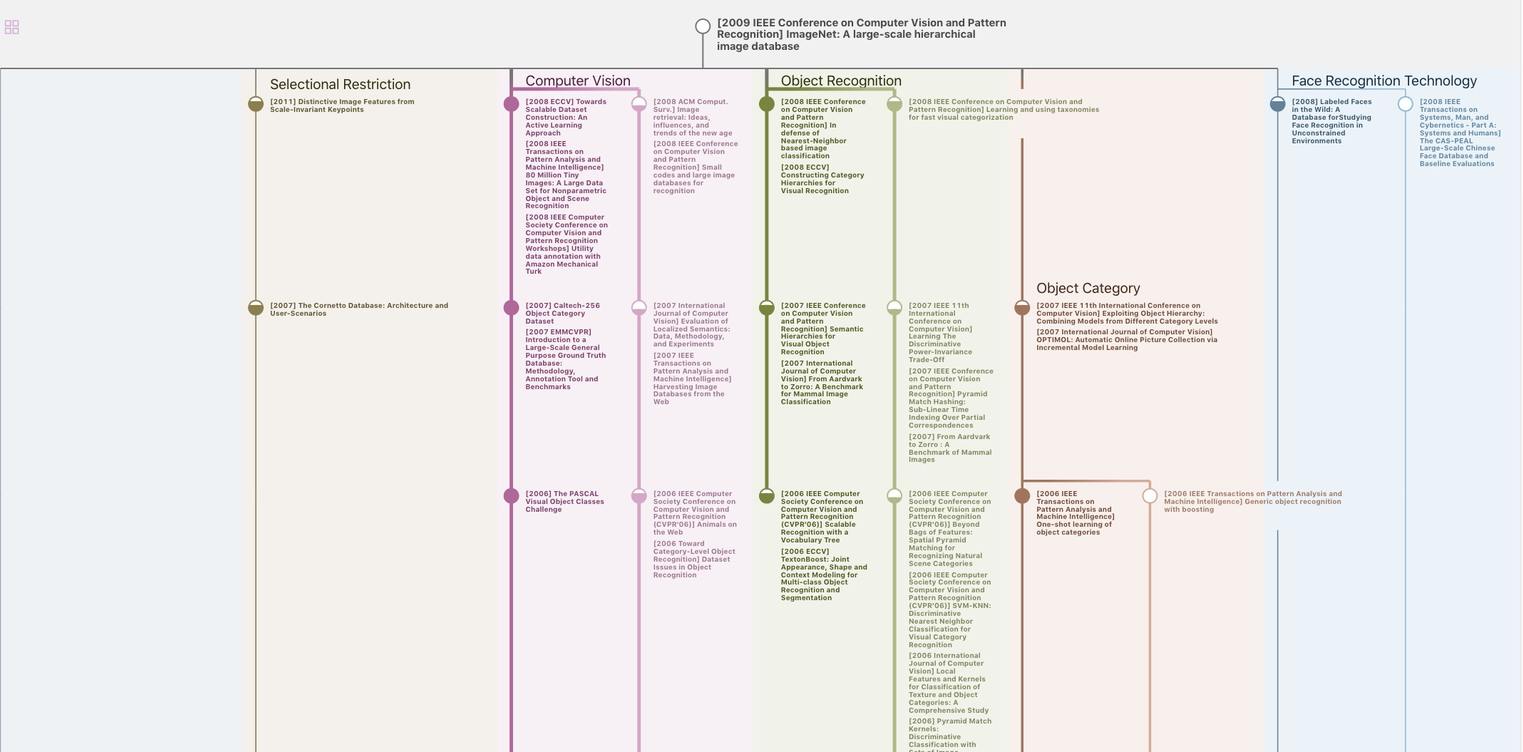
生成溯源树,研究论文发展脉络
Chat Paper
正在生成论文摘要