Graph convolutional networks with attention for multi-label weather recognition
NEURAL COMPUTING & APPLICATIONS(2021)
摘要
Weather recognition is a significant technique for many potential computer vision applications in our daily lives. Generally, most existing works treat weather recognition as a single-label classification task, which cannot describe the weather conditions comprehensively due to the complex co-occurrence dependencies between different weather conditions. In this paper, we propose a novel Graph Convolution Networks with Attention (GCN-A) model for multi-label weather recognition. To our best knowledge, this is the first attempt to introduce GCN into weather recognition. Specifically, we employ GCN to capture weather co-occurrence dependencies via a directed graph. The graph is built over weather labels, where each node (weather label) is represented by word embeddings of a weather label. Furthermore, we design a re-weighted mechanism to build weather correlation matrix for information propagation among different nodes in GCN. In addition, we develop a channel-wise attention module to extract informative semantic features of weather for effective model training. Compared with the state-of-the-art methods, experiment results on two widely used benchmark datasets demonstrate that our proposed GCN-A model achieves promising performance.
更多查看译文
关键词
Multi-label weather recognition,Weather co-occurrence dependencies,Directed graph,Attention
AI 理解论文
溯源树
样例
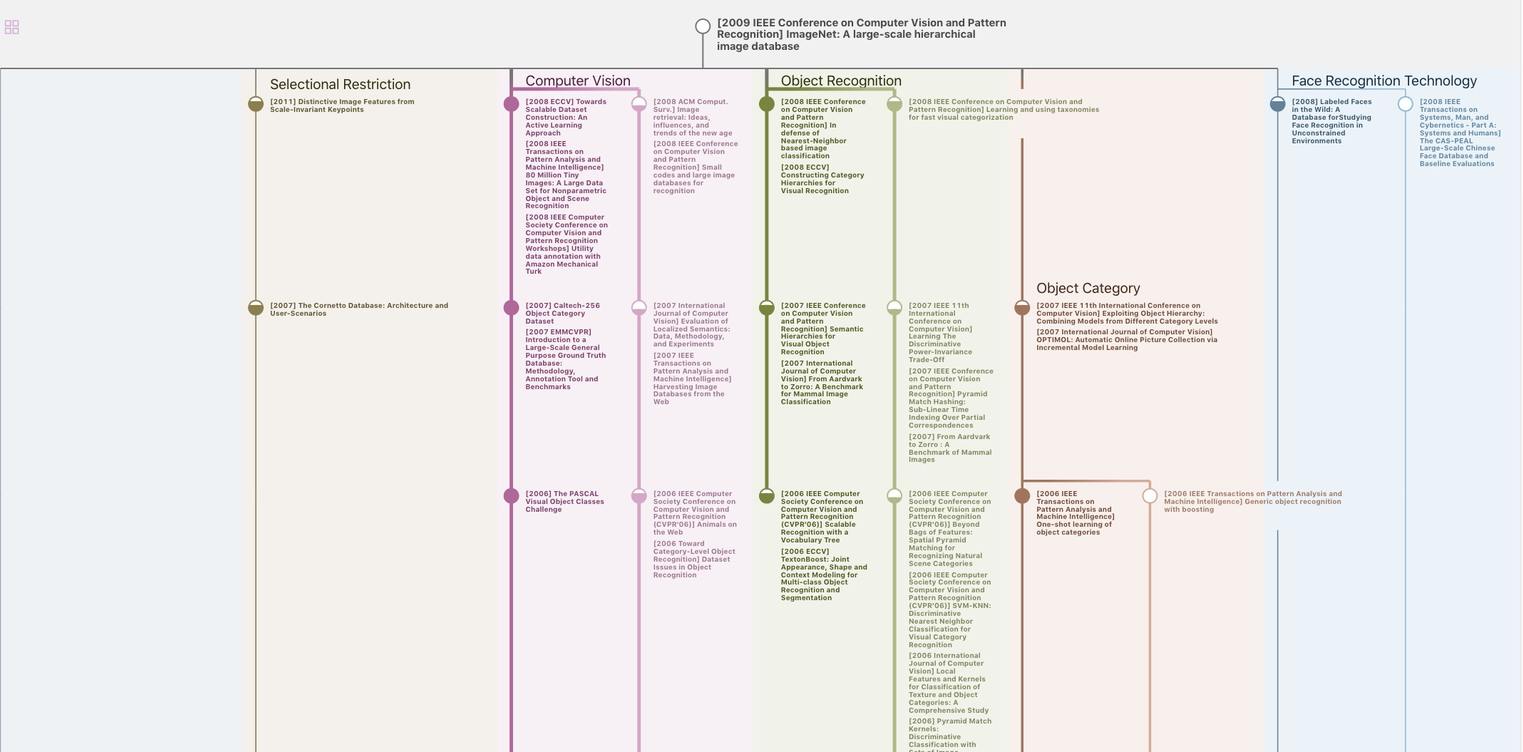
生成溯源树,研究论文发展脉络
Chat Paper
正在生成论文摘要