Regularization graph convolutional networks with data augmentation
Neurocomputing(2021)
摘要
Recently, there is a lot of progress in designing effective convolutional schemes on irregular graph structures. These graph convolutional networks mostly solve the semi-supervised learning problem on the graph where the number of labeled nodes typically is very small and the number of unlabeled nodes is very large. In the standard GCN, only labeled nodes will enter the loss function; unlabeled data will not get into the loss function. The model will be unstable due to the unconstrained nature of unlabeled nodes. This will lead to overfitting and negatively affect the generalization ability of the model. To solve this problem, we propose to regularize the graph convolutional networks using data augmentation. We provide the details of the regularization GCN and SGC model and design rich data augmentation schemes including individual features perturbation, individual edge addition, and combination of them. To verify the performance of our method, we conduct experiments on standard benchmarks and explore the intensity effect of different data augmentation strategies. The experimental results on the node classification tasks demonstrate that our method can outperform baseline methods.
更多查看译文
关键词
Semi-supervised learning,Graph convolutional networks,Regularization model,Data augmentation schemes,Generalization
AI 理解论文
溯源树
样例
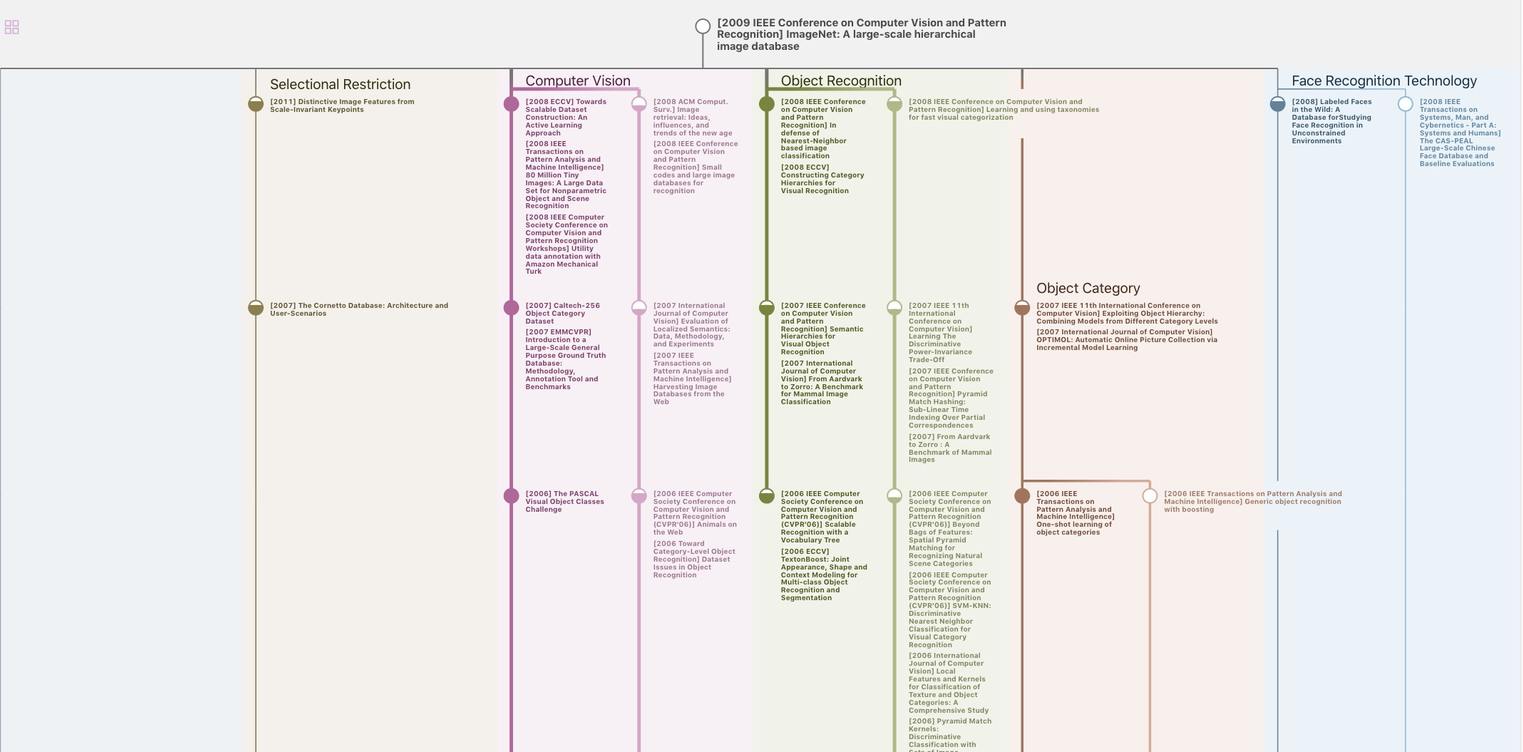
生成溯源树,研究论文发展脉络
Chat Paper
正在生成论文摘要