Automated Diagnosis Of Major Depressive Disorder Using Brain Effective Connectivity And 3d Convolutional Neural Network
IEEE ACCESS(2021)
摘要
Major depressive disorder (MDD), which is also known as unipolar depression, is one of the leading sources of functional frailty. MDD is mostly a chronic disorder that requires a long duration of treatment and clinical management. One of the critical issues in MDD treatment is the need for it's early diagnosis. Conventional tools in MDD diagnosis are based on questionnaires and other forms of psychiatric evaluations. However, the subjective nature of these tools may lead to misleading inferences. Recently, brain electroencephalography (EEG) signals have been used for the quantitative diagnosis of MDD. Nevertheless, a further improvement of the proposed methods in terms of accuracy and clinical utility is required. In this study, EEG signals from 30 MDD and 30 healthy control (HC) are used to estimate the effective connectivity within the brain default mode network (DMN). Then, effective connections between the major six regions of the DMN are used to train and test a three-dimensional (3D) convolutional neural network. Here, connectivity samples generated from half of the subjects are used for training while the rest are used for testing. The results show that the proposed MDD diagnosis algorithm achieved 100% accuracy,sensitivity and specificity in classifying MDD and HC test subjects.
更多查看译文
关键词
Electroencephalography, Depression, Brain modeling, Three-dimensional displays, Task analysis, Mood, Indexes, 3D convolutional neural networks (CNN), brain effective connectivity, default mode network (DMN), major depressive disorder (MDD), partial directed coherence (PDC)
AI 理解论文
溯源树
样例
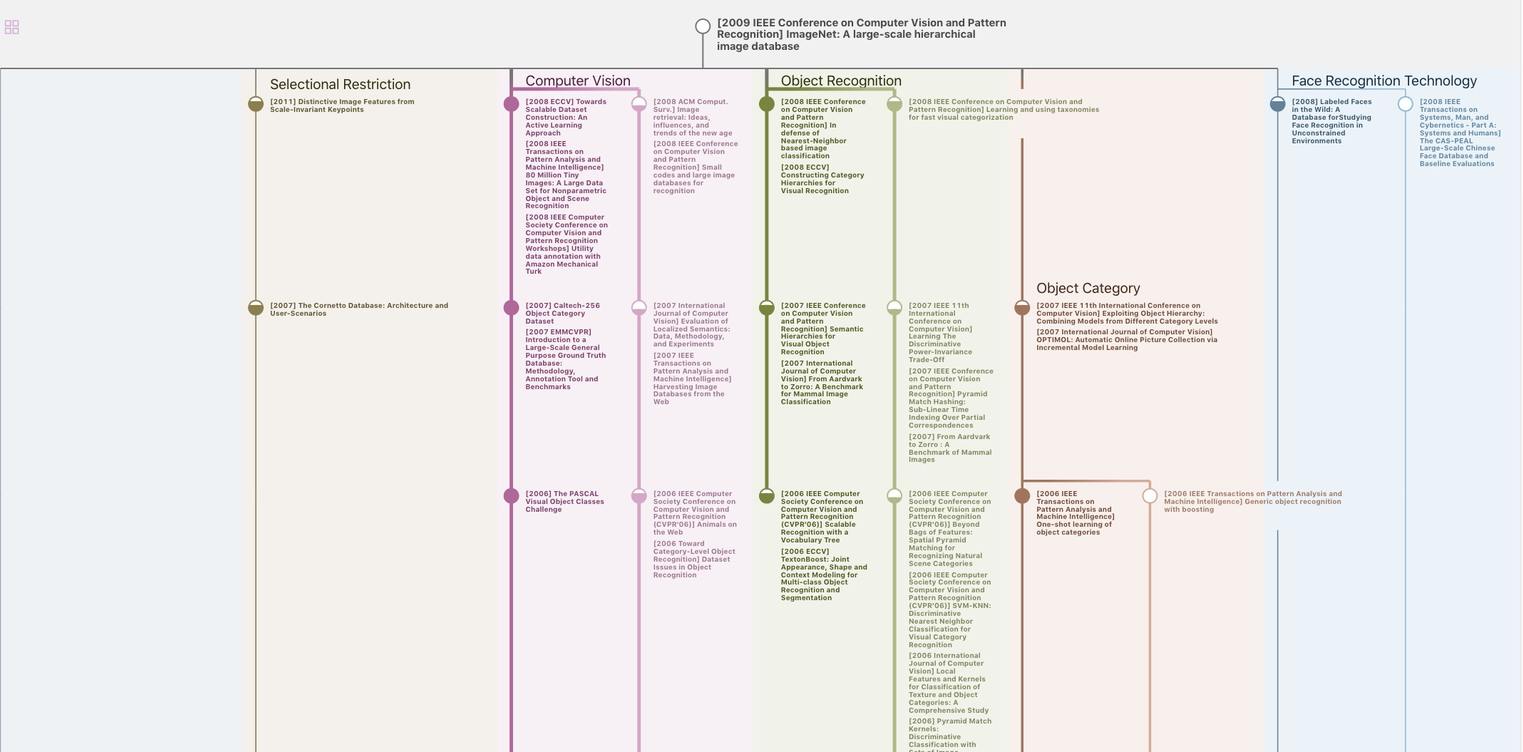
生成溯源树,研究论文发展脉络
Chat Paper
正在生成论文摘要