Exploiting feature fusion and long-term context dependencies for simultaneous ECG heartbeat segmentation and classification
INTERNATIONAL JOURNAL OF DATA SCIENCE AND ANALYTICS(2021)
摘要
Arrhythmia detection is an important research task in healthcare, which can be accomplished by classifying ECG heartbeats. Recently, there is a growing trend of applying deep learning models to solving this problem. Most existing deep learning-based methods consist of three steps: preprocessing, heartbeat segmentation and beat-wise classification. This methodology suffers from two drawbacks. First, explicit heartbeat segmentation can undermine the simplicity of the entire model. Second, performing classification on individual heartbeats fails to take into account inter-heartbeat contextual information that may be vital to accurate classification. In view of these drawbacks, we propose a novel deep learning model that simultaneously conducts heartbeat segmentation and classification. Without explicit heartbeat segmentation, the overall workflow of our method is streamlined to be simpler than existing methods. We achieve simultaneous segmentation and classification with a Faster R-CNN-based deep network that has been customized to handle ECG data. To capture inter-heartbeat contextual information, we utilize inverted residual blocks and a novel feature fusion and normalization subroutine which incorporates average pooling and max-pooling. We conduct extensive experiments on the well-known MIT-BIH arrhythmia database to validate the effectiveness of our method in both intra- and inter-patient arrhythmia detection tasks.
更多查看译文
关键词
Arrhythmia detection,ECG classification,End-to-end deep neural network,Heartbeat segmentation
AI 理解论文
溯源树
样例
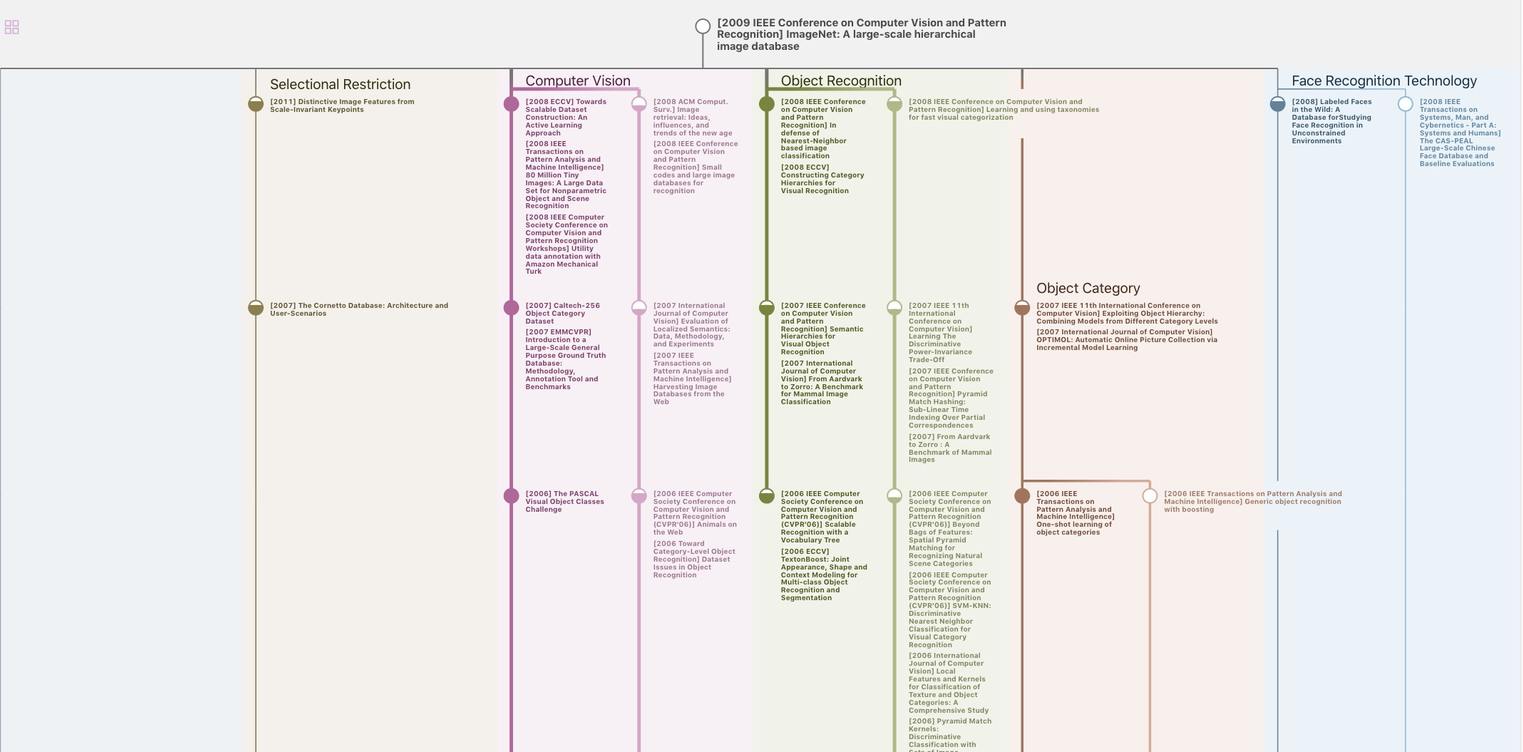
生成溯源树,研究论文发展脉络
Chat Paper
正在生成论文摘要