TransferSense: towards environment independent and one-shot wifi sensing
Personal and Ubiquitous Computing(2021)
摘要
WiFi has recently established itself as a powerful medium for radio frequency (RF) sensing due to its low cost and convenience. Many tasks, such as gesture recognition, activity recognition, and fall detection, can be implemented by measuring and calculating how the propagation of WiFi signals is affected by human activities. However, current WiFi-based sensing solutions have limited scales as they are designed for only a few activities and need to collect data and create training models in the same domain because the model established in a deployment environment is usually not applicable to new objects in the target domain. This paper presents TransferSense, an environment independent and one-shot WiFi sensing method based on deep learning. Firstly, amplitude and phase information of channel state information (CSI) are combined to increase the number of features to solve the problem of insufficient features due to single-source information. Secondly, TransferSense converts RF sensing tasks to image classification tasks and fuses low-level and high-level semantic features extracted from a pre-trained convolutional neural network to achieve an end-to-end high-precision sensing for activity recognition. Finally, TransferSense applies a transfer learning method with a small number of labeled samples in the target domain to perform high-precision cross-domain sensing, which can reduce the data collection cost in the target domain. We verified the effectiveness of TransferSense using two representative WiFi sensing applications, gait identification and sign recognition. In a single deployment environment, TransferSense achieved more than 97% human gait identification accuracy for 44 users and more than 81% sign language recognition for 100 isolated sign language words. In the case of new object recognition in the cross-domain sensing, TransferSense achieved more than 77% human gait identification accuracy for 10 new users, more than 88% sign language recognition for 10 new isolated sign language words, and more than 81% gesture identification for 2 new gestures.
更多查看译文
关键词
WiFi, Channel state information, Transfer learning, Human interaction
AI 理解论文
溯源树
样例
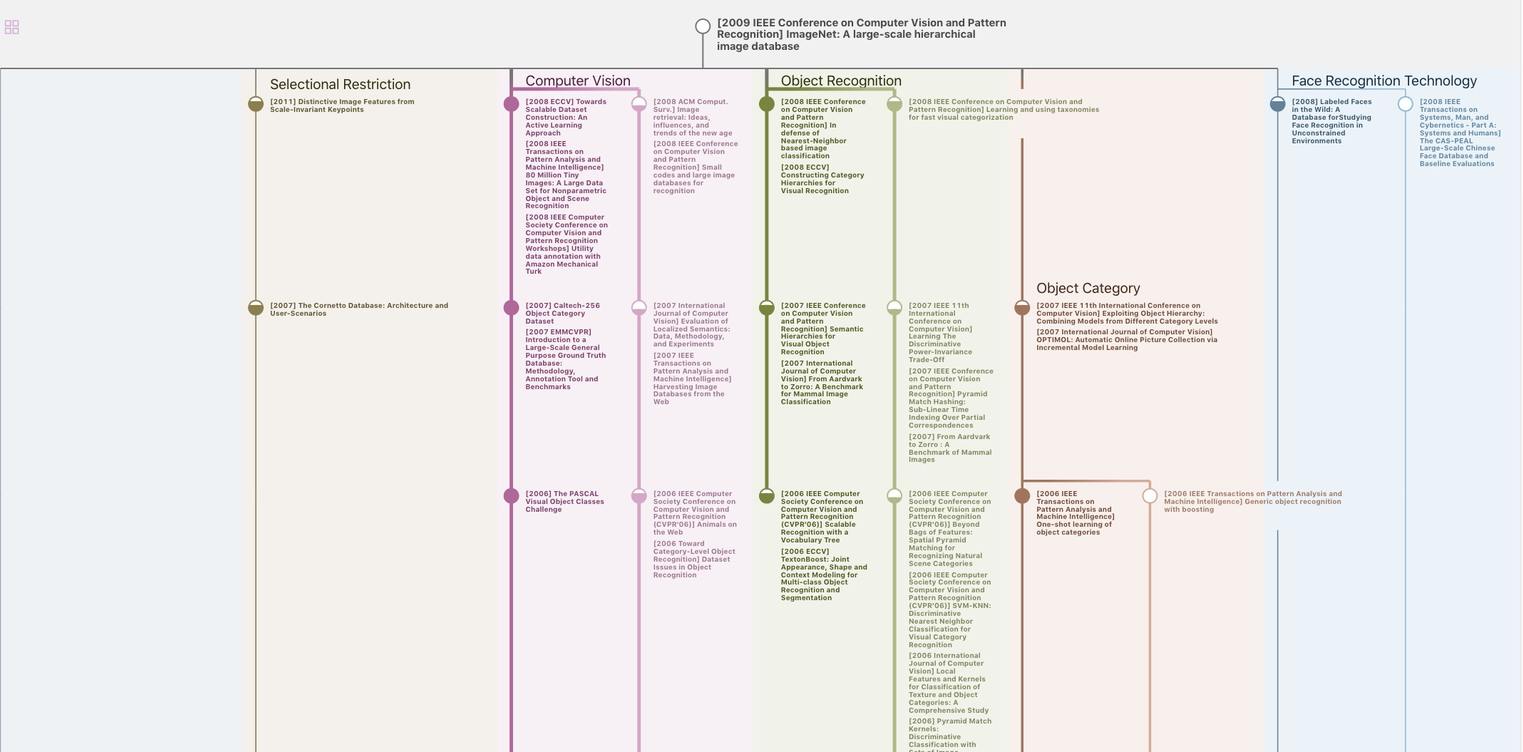
生成溯源树,研究论文发展脉络
Chat Paper
正在生成论文摘要