From reanalysis to satellite observations: gap-filling with imbalanced learning
GeoInformatica(2021)
摘要
Increasing the spatial coverage and temporal resolution of Earth surface monitoring can significantly improve forecasting or monitoring capabilities in the context of smart city, such as extreme weather forecasting, ecosystem monitoring and anthropogenic impact monitoring. As an essential data source for Earth’s surface monitoring, most satellite observations exist data gaps due to various factors like the limitations of measuring equipment, the interferences of environments, and the delay or loss of data updates. Although many efforts have been conducted to fill the gaps in the last decade, the existing techniques cannot efficiently address the problem. In this paper, we extensively study the gap-filling problem of satellite observations using imbalanced learning. Specifically, we propose a framework called Reanalysis to Satellite (R2S) to simulate satellite observations with reanalysis data. In the R2S framework, we propose a generic method called Spatial Temporal Match (STM), matching reanalysis data and satellite observations to construct the Reanalysis-Satellite (R-S) dataset used to train the model. Based on the R-S dataset, we propose a novel method called Semi-imbalanced (SIMBA) to handle the imbalance problem of gap-filling by taking advantages of traditional machine learning and imbalanced learning. We construct a hybrid model in the R2S framework for the Soil Moisture Active Passive (SMAP) satellite observations of the tropical cyclone wind speed. Extensive experiments demonstrate the hybrid model outperforms the traditional machine learning model and closely approximates in situ observations.
更多查看译文
关键词
Imbalanced learning, Gap-filling, Satellite observations, Reanalysis data, Tropical cyclone, Smart city
AI 理解论文
溯源树
样例
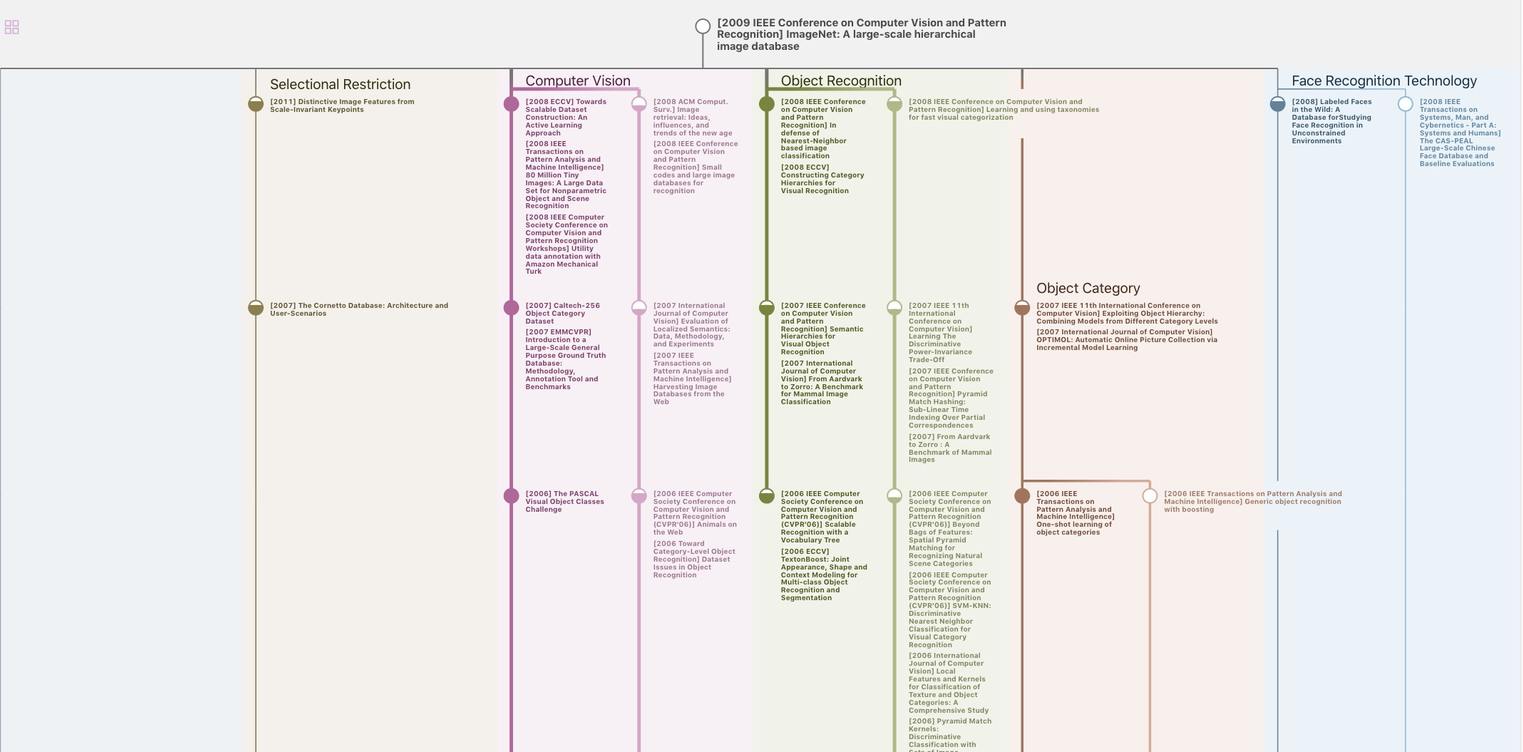
生成溯源树,研究论文发展脉络
Chat Paper
正在生成论文摘要