Feature Space Reduction, Using Pca In The Algorithm For Epilepsy Detection, Using An Adaptive Neuro-Fuzzy Inference System And Comparative Analysis
ACTA POLYTECHNICA HUNGARICA(2020)
摘要
This study presents an upgrade to our previously published algorithm for the detection of Epilepsy. The upgrade in the old algorithm, which was based on wavelet transform (WT) for feature extraction, and Adaptive Neuro-Fuzzy Inference System (ANFIS) for classification, is made by using Principal Component Analysis (PCA) in order to reduce the number of features used, for the training the ANFIS network. In order to make a comparison of the old and the upgraded algorithm with PCA, i.e. evaluating both algorithms, in terms of training performance and classification accuracies, comparative analysis of the both algorithms was made, when using different data splitting methods, and different input space partitioning methods. It was concluded that the upgraded algorithm exhibits a satisfactory performance, and in some cases, performs better than the old algorithm, even though the number of features is significantly reduced (from 20 to 7), which plays a crucial role in making the new algorithm more resistant to overfitting.
更多查看译文
关键词
Adaptive Neuro-Fuzzy Inference System (ANFIS), wavelet transform, fuzzy logic, Finite Impulse Response (FIR) filter, electroencephalogram (EEG), comparative analysis, normalization, input space partitioning, Principal Component Analysis (PCA)
AI 理解论文
溯源树
样例
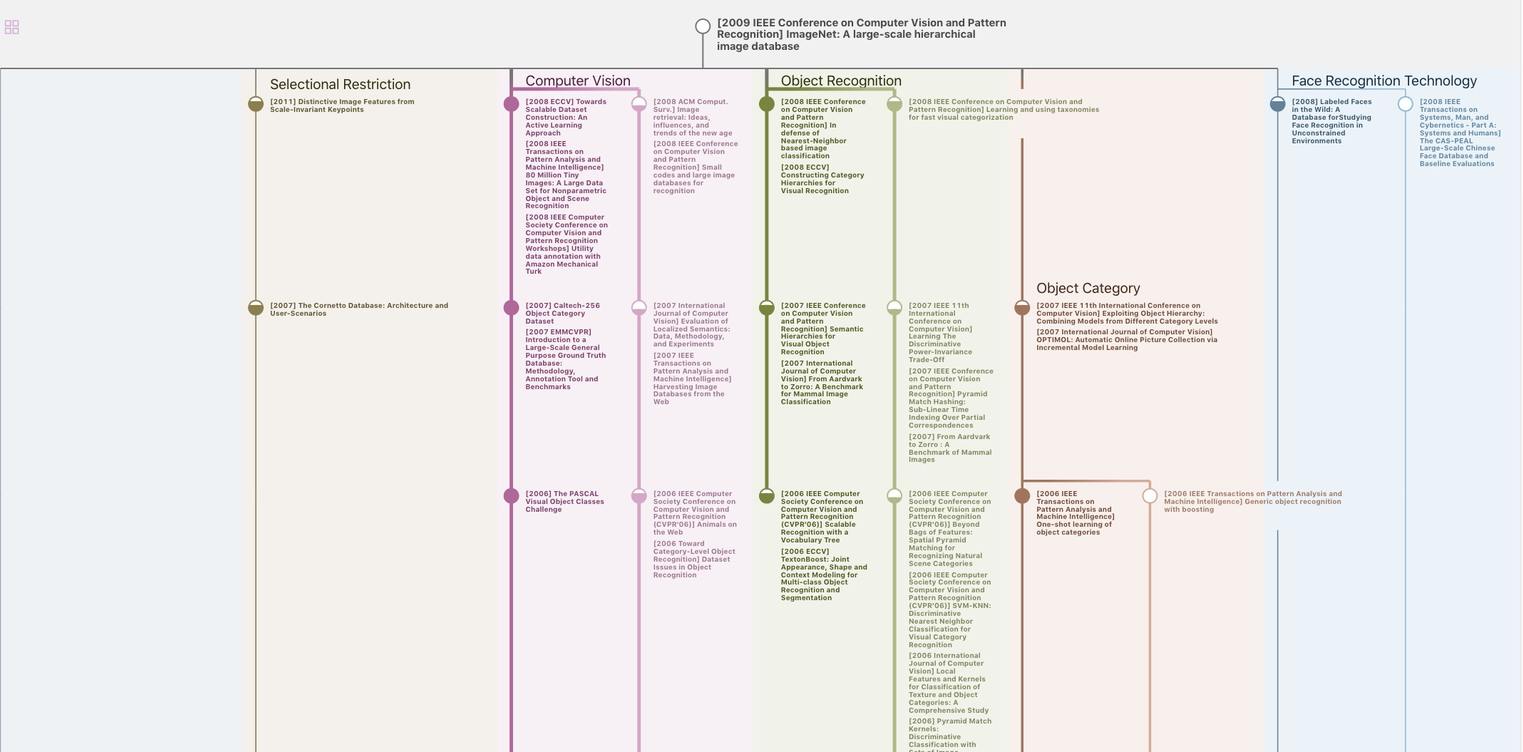
生成溯源树,研究论文发展脉络
Chat Paper
正在生成论文摘要