Reinforcement-Learning-Based Asynchronous Formation Control Scheme For Multiple Unmanned Surface Vehicles
APPLIED SCIENCES-BASEL(2021)
摘要
The high performance and efficiency of multiple unmanned surface vehicles (multi-USV) promote the further civilian and military applications of coordinated USV. As the basis of multiple USVs' cooperative work, considerable attention has been spent on developing the decentralized formation control of the USV swarm. Formation control of multiple USV belongs to the geometric problems of a multi-robot system. The main challenge is the way to generate and maintain the formation of a multi-robot system. The rapid development of reinforcement learning provides us with a new solution to deal with these problems. In this paper, we introduce a decentralized structure of the multi-USV system and employ reinforcement learning to deal with the formation control of a multi-USV system in a leader-follower topology. Therefore, we propose an asynchronous decentralized formation control scheme based on reinforcement learning for multiple USVs. First, a simplified USV model is established. Simultaneously, the formation shape model is built to provide formation parameters and to describe the physical relationship between USVs. Second, the advantage deep deterministic policy gradient algorithm (ADDPG) is proposed. Third, formation generation policies and formation maintenance policies based on the ADDPG are proposed to form and maintain the given geometry structure of the team of USVs during movement. Moreover, three new reward functions are designed and utilized to promote policy learning. Finally, various experiments are conducted to validate the performance of the proposed formation control scheme. Simulation results and contrast experiments demonstrate the efficiency and stability of the formation control scheme.
更多查看译文
关键词
deep reinforcement, formation control, formation generation, formation maintenance learning, multi-USV system
AI 理解论文
溯源树
样例
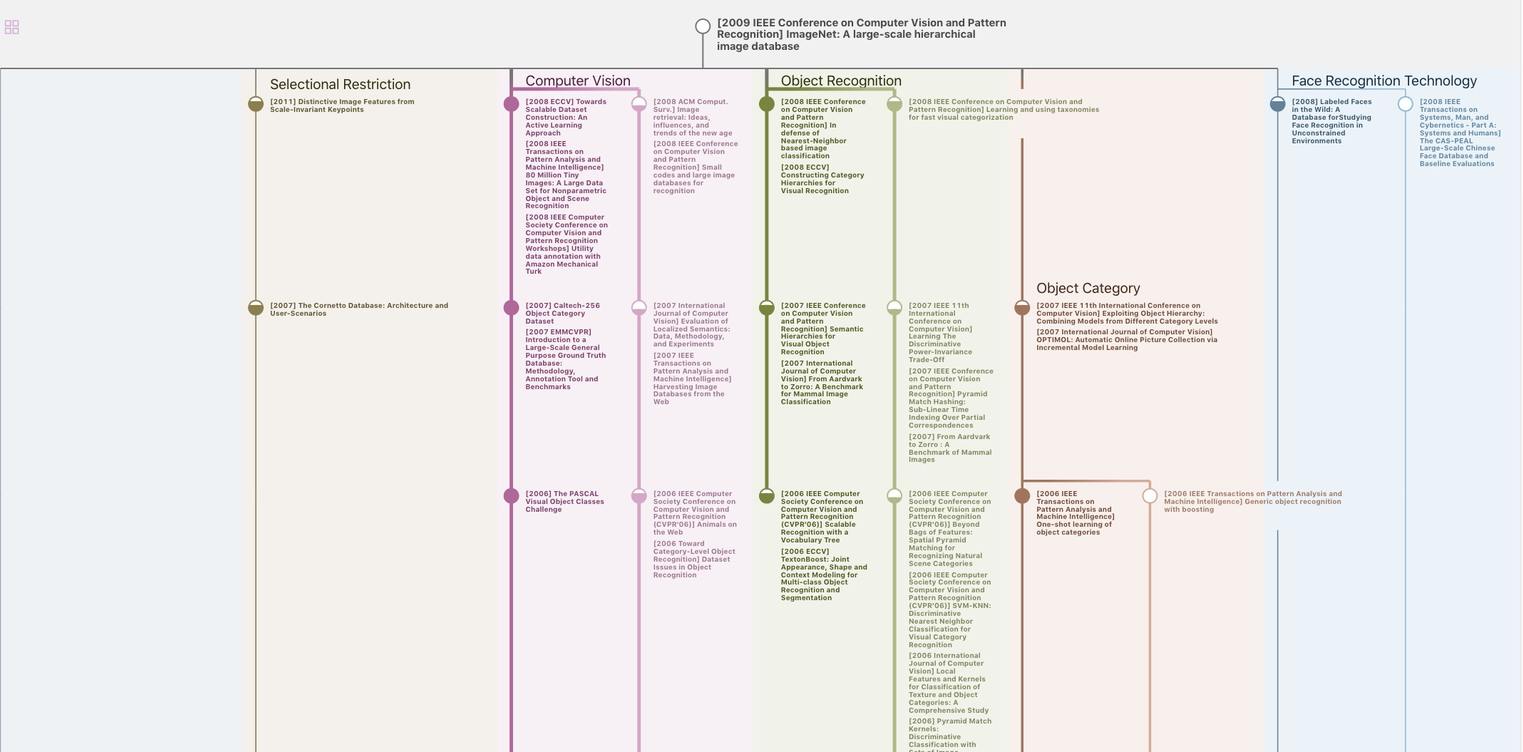
生成溯源树,研究论文发展脉络
Chat Paper
正在生成论文摘要