Adaptive Spatiotemporal Graph Convolutional Networks for Motor Imagery Classification
IEEE Signal Processing Letters(2021)
摘要
Classification of electroencephalogram-based motor imagery (MI-EEG) tasks is crucial in brain computer interfaces (BCI). In view of the characteristics of non-stationarity, time-variability and individual diversity of EEG signals, a novel framework based on graph neural network is proposed for MI-EEG classification. First, an adaptive graph convolutional layer (AGCL) is constructed, by which the electrode channel information are integrated dynamically. We further propose an adaptive spatiotemporal graph convolutional network (ASTGCN), which fully exploits the characteristics of EEG signals in time domain and the channel correlations in spatial domain simultaneously. We execute the experiments using EEG signals recorded at motor imagery scenarios, where twenty-five healthy subjects performed MI movements of the right hand and feet to generate motor commands. Experimental results reveal that the proposed method outperforms state-of-the-art methods in terms of both classification quality and robustness. The advantages of ASTGCN include high accuracy, high efficiency, and robustness to cross-trial and cross-subject variations, making it an ideal candidate for long-term MI-EEG applications.
更多查看译文
关键词
Brain computer interfaces (BCI),electroencephalogram (EEG),motor imagery (MI),graph neural network,spatiotemporal structure
AI 理解论文
溯源树
样例
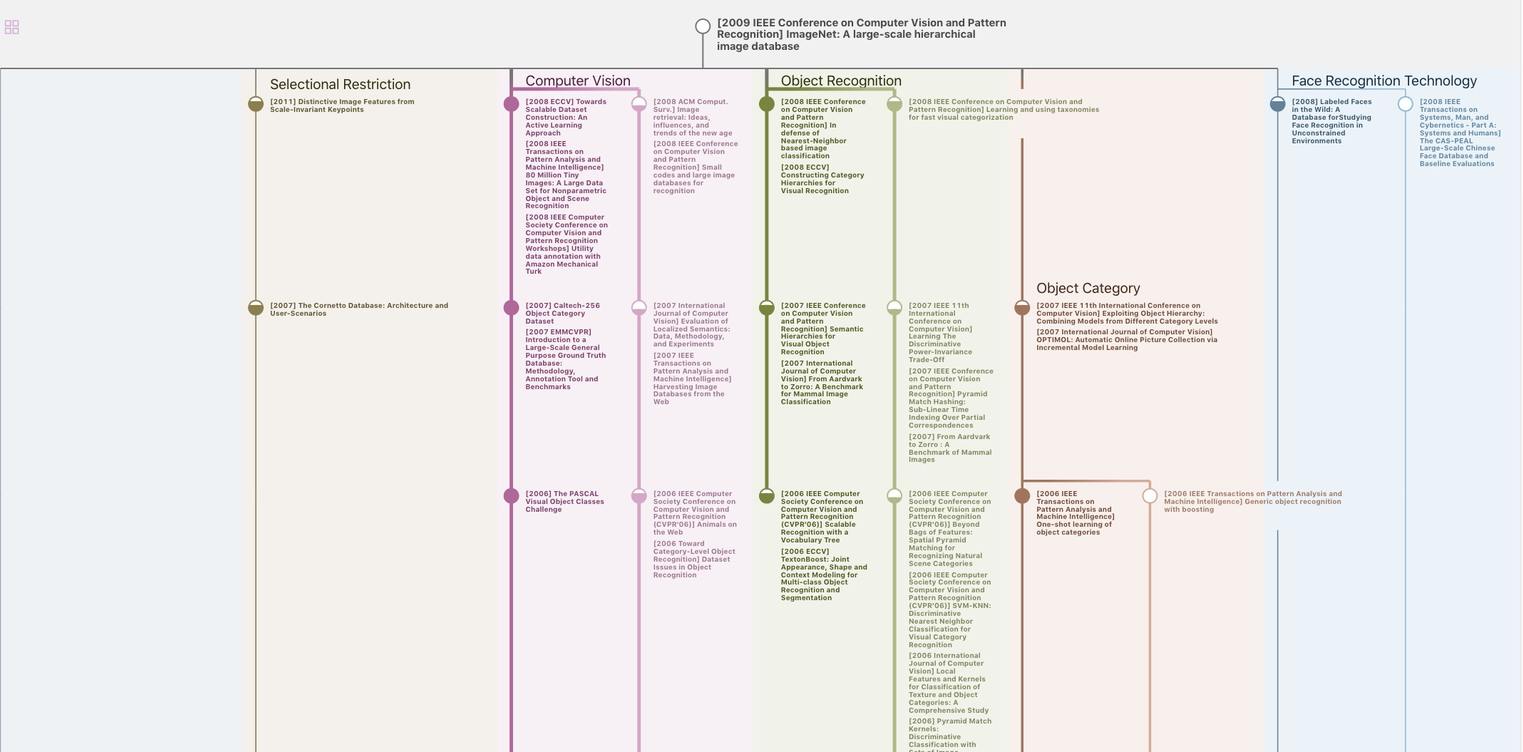
生成溯源树,研究论文发展脉络
Chat Paper
正在生成论文摘要